DeepSeek’s R1 and OpenAI’s Deep Research just redefined AI — RAG, distillation, and custom models will never be the same - Related to build, deepseek’s, deep, making, hugging
DeepSeek’s R1 and OpenAI’s Deep Research just redefined AI — RAG, distillation, and custom models will never be the same
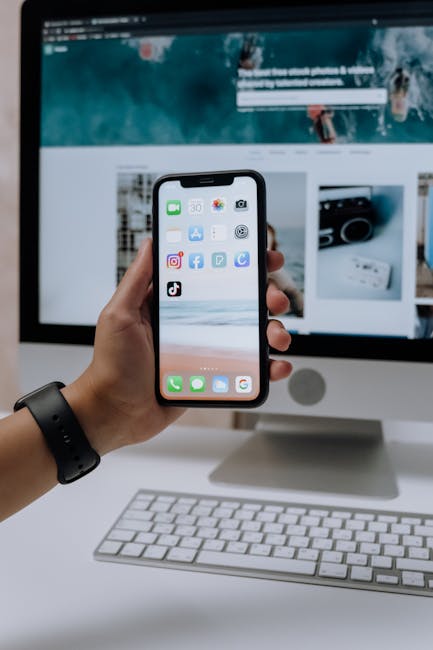
Things are moving quickly in AI — and if you’re not keeping up, you’re falling behind.
Two recent developments are reshaping the landscape for developers and enterprises alike: DeepSeek’s R1 model release and OpenAI’s new Deep Research product. Together, they’re redefining the cost and accessibility of powerful reasoning models, which has been well reported on. Less talked about, however, is how they’ll push companies to use techniques like distillation, supervised fine-tuning (SFT), reinforcement learning (RL) and retrieval-augmented generation (RAG) to build smarter, more specialized AI applications.
After the initial excitement around the amazing achievements of DeepSeek begins to settle, developers and enterprise decision-makers need to consider what it means for them. From pricing and performance to hallucination risks and the importance of clean data, here’s what these breakthroughs mean for anyone building AI today.
Cheaper, transparent, industry-leading reasoning models – but through distillation.
The headline with DeepSeek-R1 is simple: It delivers an industry-leading reasoning model at a fraction of the cost of OpenAI’s o1. Specifically, it’s about 30 times cheaper to run, and unlike many closed models, DeepSeek offers full transparency around its reasoning steps. For developers, this means you can now build highly customized AI models without breaking the bank — whether through distillation, fine-tuning or simple RAG implementations.
Distillation, in particular, is emerging as a powerful tool. By using DeepSeek-R1 as a “teacher model,” companies can create smaller, task-specific models that inherit R1’s superior reasoning capabilities. These smaller models, in fact, are the future for most enterprise companies. The full R1 reasoning model can be too much for what companies need — thinking too much, and not taking the decisive action companies need for their specific domain applications.
“One of the things that no one is really talking about, certainly in the mainstream media, is that, actually, reasoning models are not working that well for things like agents,” noted Sam Witteveen, a machine learning (ML) developer who works on AI agents that are increasingly orchestrating enterprise applications.
As part of its release, DeepSeek distilled its own reasoning capabilities onto a number of smaller models, including open-source models from Meta’s Llama family and Alibaba’s Qwen family, as described in its paper. It’s these smaller models that can then be optimized for specific tasks. This trend toward smaller, fast models to serve custom-built needs will accelerate: Eventually there will be armies of them.
“We are starting to move into a world now where people are using multiple models. They’re not just using one model all the time,” noted Witteveen. And this includes the low-cost, smaller closed-sourced models from Google and OpenAI as well. “The means that models like Gemini Flash, GPT-4o Mini, and these really cheap models actually work really well for 80% of use cases.”.
If you work in an obscure domain, and have resources: Use SFT….
After the distilling step, enterprise companies have a few options to make sure the model is ready for their specific application. If you’re a corporation in a very specific domain, where details are not on the web or in books — which large language models (LLMs) typically train on — you can inject it with your own domain-specific data sets, with SFT. One example would be the ship container-building industry, where specifications, protocols and regulations are not widely available.
DeepSeek showed that you can do this well with “thousands” of question-answer data sets. For an example of how others can put this into practice, IBM engineer Chris Hay demonstrated how he fine-tuned a small model using his own math-specific datasets to achieve lightning-fast responses — outperforming OpenAI’s o1 on the same tasks (View the hands-on video here.).
Additionally, companies wanting to train a model with additional alignment to specific preferences — for example, making a customer support chatbot sound empathetic while being concise — will want to do some RL. This is also good if a organization wants its chatbot to adapt its tone and recommendation based on user feedback. As every model gets good at everything, “personality” is going to be increasingly big, Wharton AI professor Ethan Mollick expressed on X.
These SFT and RL steps can be tricky for companies to implement well, however. Feed the model with data from one specific domain area, or tune it to act a certain way, and it suddenly becomes useless for doing tasks outside of that domain or style.
For most companies, RAG will be good enough.
For most companies, however, RAG is the easiest and safest path forward. RAG is a relatively straight-forward process that allows organizations to ground their models with proprietary data contained in their own databases — ensuring outputs are accurate and domain-specific. Here, an LLM feeds a user’s prompt into vector and graph databases to search information relevant to that prompt. RAG processes have gotten very good at finding only the most relevant content.
This approach also helps counteract some of the hallucination issues associated with DeepSeek, which currently hallucinates 14% of the time compared to 8% for OpenAI’s o3 model, , a vendor that helps companies with the RAG process.
This distillation of models plus RAG is where the magic will come for most companies. It has become so incredibly easy to do, even for those with limited data science or coding expertise. I personally downloaded the DeepSeek distilled [website] Qwen model, the smallest one, so that it could fit nicely on my Macbook Air. I then loaded up some PDFs of job applicant resumes into a vector database, then asked the model to look over the applicants to tell me which ones were qualified to work at VentureBeat. (In all, this took me 74 lines of code, which I basically borrowed from others doing the same).
I loved that the Deepseek distilled model showed its thinking process behind why or why not it recommended each applicant — a transparency that I wouldn’t have gotten easily before Deepseek’s release.
In my recent video discussion on DeepSeek and RAG, I walked through how simple it has become to implement RAG in practical applications, even for non-experts. Witteveen also contributed to the discussion by breaking down how RAG pipelines work and why enterprises are increasingly relying on them instead of fully fine-tuning models. (Watch it here).
OpenAI Deep Research: Extending RAG’s capabilities — but with caveats.
While DeepSeek is making reasoning models cheaper and more transparent, OpenAI’s Deep Research represents a different but complementary shift. It can take RAG to a new level by crawling the web to create highly customized research. The output of this research can then be inserted as input into the RAG documents companies can use, alongside their own data.
This functionality, often referred to as agentic RAG, allows AI systems to autonomously seek out the best context from across the internet, bringing a new dimension to knowledge retrieval and grounding.
Open AI’s Deep Research is similar to tools like Google’s Deep Research, Perplexity and [website], but OpenAI tried to differentiate its offering by suggesting its superior chain-of-thought reasoning makes it more accurate. This is how these tools work: A firm researcher requests the LLM to find all the information available about a topic in a well-researched and cited analysis. The LLM then responds by asking the researcher to answer another 20 sub-questions to confirm what is wanted. The research LLM then goes out and performs 10 or 20 web searches to get the most relevant data to answer all those sub-questions, then extract the knowledge and present it in a useful way.
However, this innovation isn’t without its challenges. Vectara CEO Amr Awadallah cautioned about the risks of relying too heavily on outputs from models like Deep Research. He questions whether indeed it is more accurate: “It’s not clear that this is true,” Awadallah noted. “We’re seeing articles and posts in various forums saying no, they’re getting lots of hallucinations still, and Deep Research is only about as good as other solutions out there on the market.”.
In other words, while Deep Research offers promising capabilities, enterprises need to tread carefully when integrating its outputs into their knowledge bases. The grounding knowledge for a model should come from verified, human-approved insights to avoid cascading errors, Awadallah expressed.
The cost curve is crashing: Why this matters.
The most immediate impact of DeepSeek’s release is its aggressive price reduction. The tech industry expected costs to come down over time, but few anticipated just how quickly it would happen. DeepSeek has proven that powerful, open models can be both affordable and efficient, creating opportunities for widespread experimentation and cost-effective deployment.
Awadallah emphasized this point, noting that the real game-changer isn’t just the training cost — it’s the inference cost, which for DeepSeek is about 1/30th of OpenAI’s o1 or o3 for inference cost per token. “The margins that OpenAI, Anthropic and Google Gemini were able to capture will now have to be squished by at least 90% because they can’t stay competitive with such high pricing,” stated Awadallah.
Not only that, those costs will continue to go down. Anthropic CEO Dario Amodei expressed not long ago that the cost of developing models continues to drop at around a 4x rate each year. It follows that the rate that LLM providers charge to use them will continue to drop as well.
“I fully expect the cost to go to zero,” stated Ashok Srivastava, CDO of Intuit, a corporation that has been driving AI hard in its tax and accounting software offerings like TurboTax and Quickbooks. “…and the latency to go to zero. They’re just going to be commodity capabilities that we will be able to use.”.
This cost reduction isn’t just a win for developers and enterprise individuals; it’s a signal that AI innovation is no longer confined to big labs with billion-dollar budgets. The barriers to entry have dropped, and that’s inspiring smaller companies and individual developers to experiment in ways that were previously unthinkable. Most importantly, the models are so accessible that any business professional will be using them, not just AI experts, expressed Srivastava.
DeepSeek’s disruption: Challenging “Big AI’s” stronghold on model development.
Most importantly, DeepSeek has shattered the myth that only major AI labs can innovate. For years, companies like OpenAI and Google positioned themselves as the gatekeepers of advanced AI, spreading the belief that only top-tier PhDs with vast resources could build competitive models.
DeepSeek has flipped that narrative. By making reasoning models open and affordable, it has empowered a new wave of developers and enterprise companies to experiment and innovate without needing billions in funding. This democratization is particularly significant in the post-training stages — like RL and fine-tuning — where the most exciting developments are happening.
DeepSeek exposed a fallacy that had emerged in AI — that only the big AI labs and companies could really innovate. This fallacy had forced a lot of other AI builders to the sidelines. DeepSeek has put a stop to that. It has given everyone inspiration that there’s a ton of ways to innovate in this area.
The Data imperative: Why clean, curated data is the next action-item for enterprise companies.
While DeepSeek and Deep Research offer powerful tools, their effectiveness ultimately hinges on one critical factor: Data quality. Getting your data in order has been a big theme for years, and has accelerated over the past nine years of the AI era. But it has become even more crucial with generative AI, and now with DeepSeek’s disruption, it’s absolutely key.
Hilary Packer, CTO of American Express, underscored this in an interview with VentureBeat: “The aha! moment for us, honestly, was the data. You can make the best model selection in the world… but the data is key. Validation and accuracy are the holy grail right now of generative AI.”.
This is where enterprises must focus their efforts. While it’s tempting to chase the latest models and techniques, the foundation of any successful AI application is clean, well-structured data. Whether you’re using RAG, SFT or RL, the quality of your data will determine the accuracy and reliability of your models.
And, while many companies aspire to perfect their entire data ecosystems, the reality is that perfection is elusive. Instead, businesses should focus on cleaning and curating the most critical portions of their data to enable point AI applications that deliver immediate value.
Related to this, a lot of questions linger around the exact data that DeepSeek used to train its models on, and this in turn raises questions about the inherent bias of the knowledge stored in its model weights. But that’s no different from questions around other open-source models, such as Meta’s Llama model series. Most enterprise individuals have found ways to fine-tune or ground the models with RAG enough so that they can mitigate any problems around such biases. And that’s been enough to create serious momentum within enterprise companies toward accepting open source, indeed even leading with open source.
Similarly, there’s no question that many companies will be using DeepSeek models, regardless of the fear around the fact that the firm is from China. Although it’s also true that a lot of companies in highly regulated industries such as finance or healthcare are going to be cautious about using any DeepSeek model in any application that interfaces directly with customers, at least in the short-term.
Conclusion: The future of enterprise AI Is open, affordable and data-driven.
DeepSeek and OpenAI’s Deep Research are more than just new tools in the AI arsenal — they’re signals of a profound shift where enterprises will be rolling out masses of purpose-built models, extremely affordably, competent and grounded in the enterprise’s own data and approach.
For enterprises, the message is clear: The tools to build powerful, domain-specific AI applications are at your fingertips. You risk falling behind if you don’t leverage these tools. But real success will come from how you curate your data, leverage techniques like RAG and distillation and innovate beyond the pre-training phase.
As AmEx’s Packer put it: The companies that get their data right will be the ones leading the next wave of AI innovation.
Strauss Zelnick, CEO of Take-Two Interactive Software, talked about the latest plans the triple-A game publisher has with its upcoming titles and his ......
Mantle8, a French natural hydrogen exploration organization, has raised a €[website] seed round with investment from Kiko Ventures and Breakthrough Energy Ventu......
You can do a lot of things with a Bluetooth speaker, especially when the product in question is weather-resistant. Having a strong IP rating guarantee......
Hugging Face brings ‘Pi-Zero’ to LeRobot, making AI-powered robots easier to build and deploy
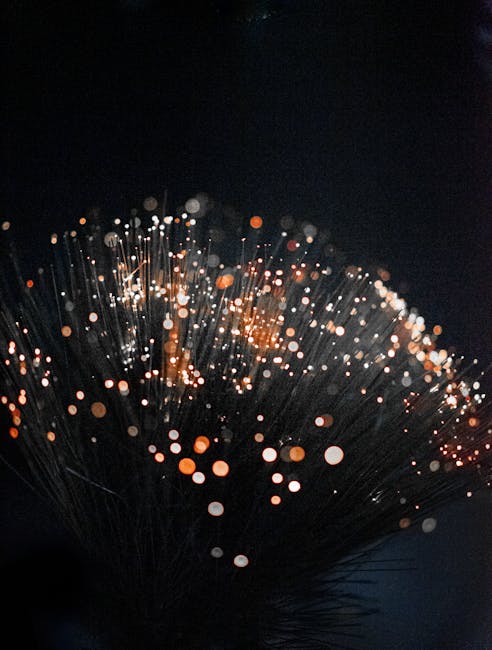
Hugging Face and Physical Intelligence have quietly launched Pi0 (Pi-Zero) this week, the first foundational model for robots that translates natural language commands directly into physical actions.
“Pi0 is the most advanced vision language action model,” Remi Cadene, a principal research scientist at Hugging Face, revealed in an X post that quickly gained attention across the AI community. “It takes natural language commands as input and directly outputs autonomous behavior.”.
This release marks a pivotal moment in robotics: The first time a foundation model for robots has been made widely available through an open-source platform. Much like ChatGPT revolutionized text generation, Pi0 aims to transform how robots learn and execute tasks.
Excited to see Pi0 by @physical_int being the first foundational robotics model to be open-sourced on @huggingface @LeRobotHF. You can now fine-tune it on your own dataset.
??? [website] — clem ? (@ClementDelangue) February 4, 2025.
How Pi0 brings ChatGPT-style learning to robotics, unlocking complex tasks.
The technology behind Pi0 represents a significant technical achievement. The model was trained on data from seven different robotic platforms and 68 unique tasks, enabling it to handle everything from delicate manipulation tasks to complex multi-step procedures. It employs a novel technique called flow matching to produce smooth, real-time action trajectories at 50Hz, making it highly precise and adaptable for real-world deployment.
New FAST technology accelerates robot training by 5X, expanding AI’s potential.
Building on this foundation, the team also introduced “Pi0-FAST,” an enhanced version of the model that incorporates a new tokenization scheme called frequency-space action sequence tokenization (FAST). This version trains five times faster than its predecessor and exhibits improved generalization across different environments and robot types.
The implications for industry are substantial. Manufacturing facilities could potentially reprogram robots for new tasks through simple verbal instructions rather than complex coding. Warehouses could deploy more flexible automation systems that adapt to changing needs. Even small businesses might find robotics more accessible, as the barrier to programming and deployment significantly decreases.
However, challenges remain. While Pi0 represents a significant advance, it still has limitations. The model occasionally struggles with very complex tasks and requires substantial computational resources. There are also questions about reliability and safety in industrial settings.
The release comes at a crucial time in the AI industry’s evolution. As companies race to develop and deploy artificial general intelligence (AGI), Pi0 represents one of the first successful attempts to bridge the gap between language models and physical world interaction.
The technology is now available through Hugging Face’s platform, where developers can download and use the pretrained policy with just a few lines of code:
policy = Pi0Policy.from_pretrained("lerobot/pi0").
For enterprise consumers, this accessibility could accelerate the adoption of advanced robotics across industries. Companies can now fine-tune the model for specific use cases, potentially reducing the time and cost associated with deploying robotic solutions.
Why enterprise leaders should pay attention to open-source robotics.
The development team has also released comprehensive documentation and training materials, making the technology accessible to a broader range of individuals. This democratization of robotics technology could lead to innovative applications across various sectors, from healthcare to retail.
As the technology matures, it could reshape how we think about automation and human-robot interaction. The ability to control robots through natural language could make robotic assistance more accessible in homes, hospitals and small businesses — areas where traditional robotics has struggled to gain traction due to programming complexity.
With this release, the future of robotics looks increasingly conversational, adaptive and accessible. While there’s still work to be done, Pi0 represents a significant step toward making versatile, intelligent robots a practical reality rather than a science fiction fantasy.
The iOS App Store is awash with apps using subscriptions and in-app payments, but our attention has been drawn to a brand new release that goes back t......
Table of Contents Table of Contents The wrong priority? Taking the long view.
We’ve not yet seen the full rollout of Apple’s M4 chip family — the M4 U......
Apple is set to unveil the long-awaited iPhone SE 4 “ in the coming days,” .
Your Netgear router might be an open door for hackers
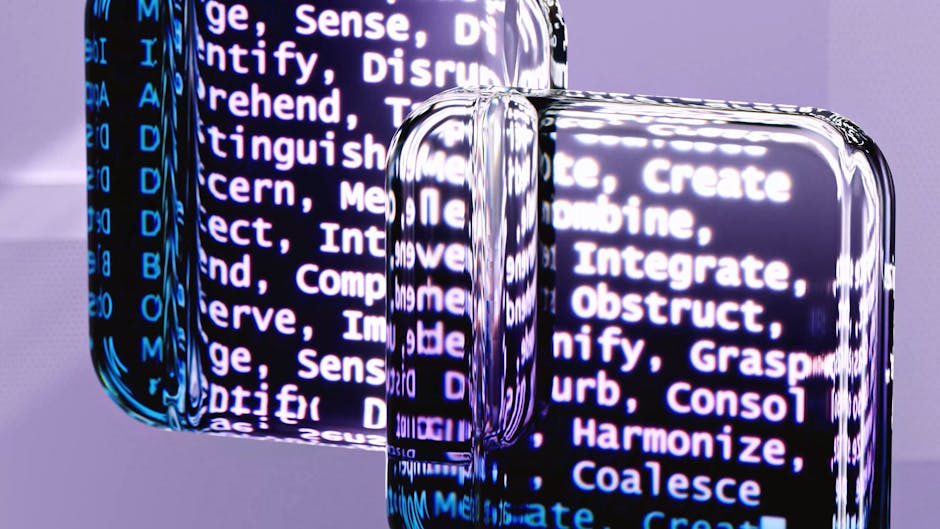
Netgear has released a security advisory addressing two critical vulnerabilities affecting Nighthawk Pro Gaming routers and certain Wi-Fi 6 access points. The firm strongly recommends that customers improvement their devices’ firmware promptly to mitigate potential risks.
The first vulnerability, identified as PSV-2023-0039, is a Remote Code Execution (RCE) flaw. This security issue allows attackers to execute arbitrary code on affected devices remotely, potentially leading to unauthorized control over the router. The second vulnerability, PSV-2021-0017, is an authentication bypass flaw, which enables attackers to circumvent authentication mechanisms and gain unauthorized access to the device’s management interface.
The affected models include Nighthawk Pro Gaming Routers such as the XR1000, XR1000v2, and XR500, as well as Wi-Fi 6 Access Points like the WAX206, WAX220, and WAX214v2.
The firm has released firmware updates to address these vulnerabilities. If you do own any of the above mentioned products, it is strongly advised to download and install the latest firmware versions for your respective devices. Detailed instructions on updating firmware can be found on Netgear’s official support page.
Routers are prime targets for cyberattacks because they serve as the main gateway between the internet and home or business networks. They are always online, making them a persistent attack surface for hackers. Many routers ship with weak default security settings, including easily guessable credentials and outdated firmware, which customers often neglect to modification. Attackers exploit these vulnerabilities to gain control over the router, using it to monitor internet traffic, launch further attacks, or redirect customers to malicious websites. Additionally, unsecured remote access attributes can allow hackers to take over routers from anywhere in the world.
Once compromised, routers can be used for various malicious activities, including botnet recruitment, DNS hijacking, and man-in-the-middle attacks. Hackers can exploit them to intercept sensitive data, gain access to IoT devices, and even use them as launch points for large-scale cyberattacks like DDoS attacks.
Since many customers are unaware of router security risks, these devices often remain unpatched and vulnerable for extended periods. To reduce the risk, customers should regularly upgrade firmware, change default credentials, disable unnecessary remote management functions, and enable strong encryption to secure their networks.
Table of Contents Table of Contents What are Super Bowl squares? How do Super Bowl squares work? What are the Super Bowl squares?
MacroCycle Technologies, a biotech aiming to reduce waste in the plastic industry, has raised $[website] in Seed funding.
Table of Contents Table of Contents Costner can be an excellent director It’s achingly sincere Its three hours breeze by.
Market Impact Analysis
Market Growth Trend
2018 | 2019 | 2020 | 2021 | 2022 | 2023 | 2024 |
---|---|---|---|---|---|---|
12.0% | 14.4% | 15.2% | 16.8% | 17.8% | 18.3% | 18.5% |
Quarterly Growth Rate
Q1 2024 | Q2 2024 | Q3 2024 | Q4 2024 |
---|---|---|---|
16.8% | 17.5% | 18.2% | 18.5% |
Market Segments and Growth Drivers
Segment | Market Share | Growth Rate |
---|---|---|
Digital Transformation | 31% | 22.5% |
IoT Solutions | 24% | 19.8% |
Blockchain | 13% | 24.9% |
AR/VR Applications | 18% | 29.5% |
Other Innovations | 14% | 15.7% |
Technology Maturity Curve
Different technologies within the ecosystem are at varying stages of maturity:
Competitive Landscape Analysis
Company | Market Share |
---|---|
Amazon Web Services | 16.3% |
Microsoft Azure | 14.7% |
Google Cloud | 9.8% |
IBM Digital | 8.5% |
Salesforce | 7.9% |
Future Outlook and Predictions
The Deepseek Openai Deep landscape is evolving rapidly, driven by technological advancements, changing threat vectors, and shifting business requirements. Based on current trends and expert analyses, we can anticipate several significant developments across different time horizons:
Year-by-Year Technology Evolution
Based on current trajectory and expert analyses, we can project the following development timeline:
Technology Maturity Curve
Different technologies within the ecosystem are at varying stages of maturity, influencing adoption timelines and investment priorities:
Innovation Trigger
- Generative AI for specialized domains
- Blockchain for supply chain verification
Peak of Inflated Expectations
- Digital twins for business processes
- Quantum-resistant cryptography
Trough of Disillusionment
- Consumer AR/VR applications
- General-purpose blockchain
Slope of Enlightenment
- AI-driven analytics
- Edge computing
Plateau of Productivity
- Cloud infrastructure
- Mobile applications
Technology Evolution Timeline
- Technology adoption accelerating across industries
- digital transformation initiatives becoming mainstream
- Significant transformation of business processes through advanced technologies
- new digital business models emerging
- Fundamental shifts in how technology integrates with business and society
- emergence of new technology paradigms
Expert Perspectives
Leading experts in the digital innovation sector provide diverse perspectives on how the landscape will evolve over the coming years:
"Technology transformation will continue to accelerate, creating both challenges and opportunities."
— Industry Expert
"Organizations must balance innovation with practical implementation to achieve meaningful results."
— Technology Analyst
"The most successful adopters will focus on business outcomes rather than technology for its own sake."
— Research Director
Areas of Expert Consensus
- Acceleration of Innovation: The pace of technological evolution will continue to increase
- Practical Integration: Focus will shift from proof-of-concept to operational deployment
- Human-Technology Partnership: Most effective implementations will optimize human-machine collaboration
- Regulatory Influence: Regulatory frameworks will increasingly shape technology development
Short-Term Outlook (1-2 Years)
In the immediate future, organizations will focus on implementing and optimizing currently available technologies to address pressing digital innovation challenges:
- Technology adoption accelerating across industries
- digital transformation initiatives becoming mainstream
These developments will be characterized by incremental improvements to existing frameworks rather than revolutionary changes, with emphasis on practical deployment and measurable outcomes.
Mid-Term Outlook (3-5 Years)
As technologies mature and organizations adapt, more substantial transformations will emerge in how security is approached and implemented:
- Significant transformation of business processes through advanced technologies
- new digital business models emerging
This period will see significant changes in security architecture and operational models, with increasing automation and integration between previously siloed security functions. Organizations will shift from reactive to proactive security postures.
Long-Term Outlook (5+ Years)
Looking further ahead, more fundamental shifts will reshape how cybersecurity is conceptualized and implemented across digital ecosystems:
- Fundamental shifts in how technology integrates with business and society
- emergence of new technology paradigms
These long-term developments will likely require significant technical breakthroughs, new regulatory frameworks, and evolution in how organizations approach security as a fundamental business function rather than a technical discipline.
Key Risk Factors and Uncertainties
Several critical factors could significantly impact the trajectory of digital innovation evolution:
Organizations should monitor these factors closely and develop contingency strategies to mitigate potential negative impacts on technology implementation timelines.
Alternative Future Scenarios
The evolution of technology can follow different paths depending on various factors including regulatory developments, investment trends, technological breakthroughs, and market adoption. We analyze three potential scenarios:
Optimistic Scenario
Rapid adoption of advanced technologies with significant business impact
Key Drivers: Supportive regulatory environment, significant research breakthroughs, strong market incentives, and rapid user adoption.
Probability: 25-30%
Base Case Scenario
Measured implementation with incremental improvements
Key Drivers: Balanced regulatory approach, steady technological progress, and selective implementation based on clear ROI.
Probability: 50-60%
Conservative Scenario
Technical and organizational barriers limiting effective adoption
Key Drivers: Restrictive regulations, technical limitations, implementation challenges, and risk-averse organizational cultures.
Probability: 15-20%
Scenario Comparison Matrix
Factor | Optimistic | Base Case | Conservative |
---|---|---|---|
Implementation Timeline | Accelerated | Steady | Delayed |
Market Adoption | Widespread | Selective | Limited |
Technology Evolution | Rapid | Progressive | Incremental |
Regulatory Environment | Supportive | Balanced | Restrictive |
Business Impact | Transformative | Significant | Modest |
Transformational Impact
Technology becoming increasingly embedded in all aspects of business operations. This evolution will necessitate significant changes in organizational structures, talent development, and strategic planning processes.
The convergence of multiple technological trends—including artificial intelligence, quantum computing, and ubiquitous connectivity—will create both unprecedented security challenges and innovative defensive capabilities.
Implementation Challenges
Technical complexity and organizational readiness remain key challenges. Organizations will need to develop comprehensive change management strategies to successfully navigate these transitions.
Regulatory uncertainty, particularly around emerging technologies like AI in security applications, will require flexible security architectures that can adapt to evolving compliance requirements.
Key Innovations to Watch
Artificial intelligence, distributed systems, and automation technologies leading innovation. Organizations should monitor these developments closely to maintain competitive advantages and effective security postures.
Strategic investments in research partnerships, technology pilots, and talent development will position forward-thinking organizations to leverage these innovations early in their development cycle.
Technical Glossary
Key technical terms and definitions to help understand the technologies discussed in this article.
Understanding the following technical concepts is essential for grasping the full implications of the security threats and defensive measures discussed in this article. These definitions provide context for both technical and non-technical readers.