Go 1.24 Brings Generic Type Aliases, Weak Pointers, Improved Finalizers, and More - Related to go, kernel, improved, perceptron, vs
Go 1.24 Brings Generic Type Aliases, Weak Pointers, Improved Finalizers, and More
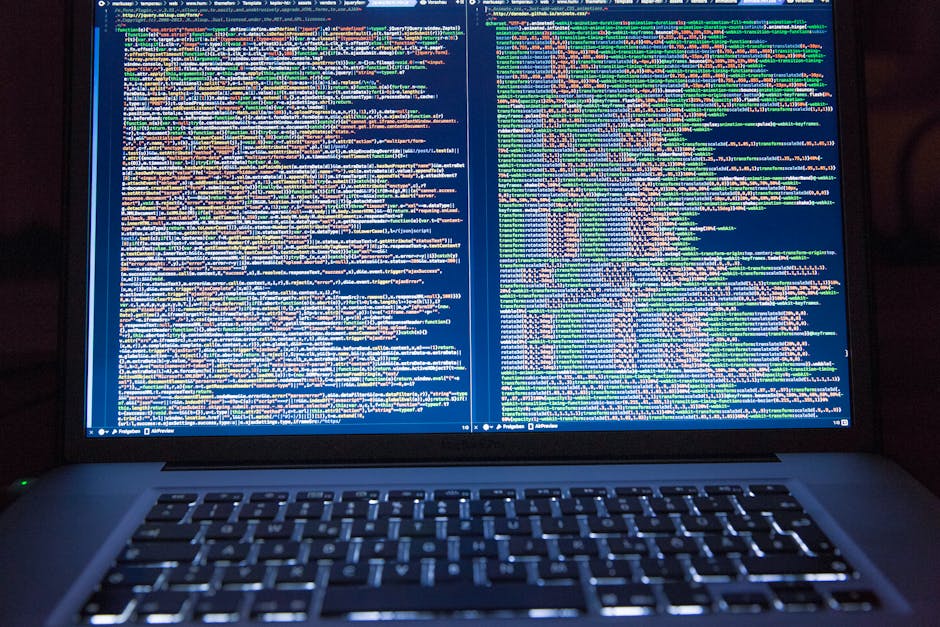
The latest release of the Go language, Go [website], introduces several critical elements, including generic type aliases, weak pointers, improved cleanup finalizers, and more. It also enhances runtime performance in map default implementation, small object allocation, and mutexes handling.
A type alias in Go provides a synonym for an existing type, which can be useful for readability and conciseness. Now, Go [website] allows creating type aliases for generic types, that is, a type alias can specify a type parameter.
type ComparableVector[T comparable] = Vector[T] type ComparableVectorOfInts = ComparableVector[int] type ThisWouldBeAnError = ComparableVector[[]int].
It's worth recalling here that Go provides a similar syntax for defining a new type based on an existing type, [website] type NewInt int . Albeit the syntax only differs in the missing = , the implications are great since NewInt cannot be used in place of int .
Interestingly, the discussion about whether introducing generic type aliases and their implications on the language has been going on for over three years.
Weak pointers do not increase the reference count of an object, so when an object is referenced only by weak pointers, the garbage collector can free it. As a consequence, you should check a weak pointer is not nil before attempting to use its value:
var strongInt int = 5 var weakInt *int weakInt = &strongInt ... [website].
Weak pointers may be useful when you want to implement, for example, an object cache to avoid objects being retained for the mere fact of being included in the cache.
Go finalizers serve the purpose of cleaning up things when an object is garbage collected. Prior to Go [website], this could be accomplished using [runtime.SetFinalizer]([website]#SetFinalizer) , which has several caveats, including the impossibility of defining more than one finalizer on the same object, the fact that finalizers will not work on objects involved in a reference cycle, and so on. To overcome these limitations, Go [website] provides a new runtime function, AddCleanup , which can be used to register a cleanup function with an object:
runtime.AddCleanup(objPointer, cleanupFunc, resourceToCleanUp) ... func cleanupFunc(resourceToCleanUp CleanUpArgType) { ... }.
The cleanup mechanism fixes the issues with finalizers mentioned above. Additionally, it ensures all cleanup functions are called sequentially in a separate goroutine.
As mentioned, Go [website] improves the runtime performance of maps. In particular, it adopts SwissTable as a base for map implementation and uses a concurrent hash-trie for the implementation of [website] .
Using SwissTable brings 30% faster access and assignment of large maps, 35% faster assignment on pre-sized maps, and 10-60% faster iteration depending on the number and size of items in the map.
Similarly, adopting a concurrent hash-trie enables the new [website] implementation to beat the old one on almost every benchmark.
Go [website] includes many more improvements and changes than what can be covered here, including new functions in bytes and strings packages, omitzero json tag, directory-limited filesystem access, etc. While the release notes are as usual quite terse you can find great video summaries on Reddit user GreenTowel3732's YouTube channel.
Telemetry data feeds are beneficial for developers and operations teams. However, observability feeds come at a cost. Some large clients spend tens ......
In modern application development, the need for real-time data processing and reactive programming has become increasingly critical. Reactive program......
Hi Buddy! Welcome back to the part 4 of the MS Rules Engine series. In this article we will be concentrating on how to use different in-built post rul......
The Perceptron Algorithm and the Kernel Trick
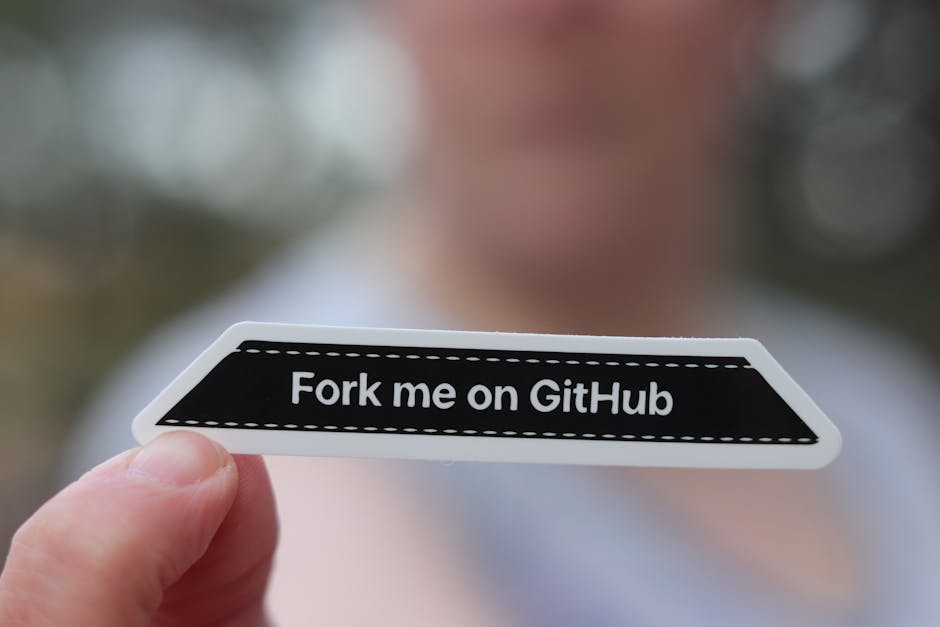
The Perceptron Algorithm is one of the earliest and most influential machine learning models, forming the foundation for modern neural networks and support vector machines (SVMs). Proposed by Frank Rosenblatt in 1958 (Rosenblatt, 1958), the perceptron is a simple yet powerful linear classifier designed for binary classification tasks.
Despite its simplicity, the perceptron introduced key concepts that remain central to machine learning today, such as iterative weight updates, the use of activation functions, and learning a decision boundary (Goodfellow, Bengio & Courville, 2016). These ideas have directly influenced the development of multi-layer neural networks by introducing weight adjustment rules that underpin backpropagation (LeCun, Bengio & Hinton, 2015).
The perceptron's weight update mechanism, based on error correction, serves as an early precursor to the gradient-based optimization used in modern deep learning (Rumelhart, Hinton & Williams, 1986). However, its limitations in handling non-linearly separable data led to the development of advanced techniques like the Kernel Trick, which extends the perceptron's capabilities to more complex datasets (Schölkopf & Smola, 2002).
The perceptron operates by learning a hyperplane that separates two classes in a dataset. Given a set of input functions and corresponding labels, the algorithm initializes weights and iteratively updates them based on classification errors. The goal is to find a hyperplane that correctly classifies all training instances, assuming the data is linearly separable.
While the perceptron is effective for linearly separable data, it has significant limitations:
Linear separability . The perceptron can only classify data that is linearly separable. If no hyperplane can perfectly separate the two classes, the algorithm will fail to converge and will continue updating weights indefinitely (Minsky & Papert, 1969).
Noisy data . The perceptron is sensitive to noise and outliers, which can prevent convergence even if the data is mostly separable.
Lack of probabilistic output . The perceptron provides a hard decision boundary without any measure of confidence or probability.
The Kernel Trick: Extending the Perceptron.
To handle non-linearly separable data, one approach is to map the input into a higher-dimensional space where it becomes linearly separable. For example, a 2D input space can be transformed into a 3D space using non-linear mapping, making linear separation possible. However, such transformations can be computationally expensive — if the input has DDD aspects, a polynomial transformation of degree p can lead to O(d^p) aspects. This exponential growth quickly becomes infeasible for large datasets.
To overcome this limitation, Aizerman et al. (1964) introduced the Kernel Trick. This technique enables models like the perceptron and support vector machines (SVMs) to operate in a high-dimensional space without explicitly computing the transformation, significantly reducing computational complexity.
Instead of computing the transformed aspects explicitly, the Kernel Trick computes the inner product of two transformed feature vectors directly using a kernel function:
This avoids the need for explicit transformation while still benefiting from the higher-dimensional representation.
The classical perceptron algorithm relies on dot products to modification weights. In a kernelized perceptron, we replace all dot products with kernel evaluations, allowing the perceptron to operate in an implicit higher-dimensional space.
This formulation means the perceptron does not need explicit feature mappings, making it computationally efficient while still benefiting from high-dimensional representations.
Non-linear decision boundaries . The kernel trick enables the perceptron to learn complex, non-linear decision boundaries.
Computational efficiency . By avoiding explicit computation of higher dimensions, the kernel trick reduces computational complexity.
Versatility . The choice of kernel function significantly impacts model performance. Different kernels capture different types of relationships in the data. Polynomial kernels are effective for capturing interactions between features, while RBF kernels are well-suited for complex, high-dimensional datasets. The sigmoid kernel is sometimes used as a proxy for neural network architectures. Selecting an appropriate kernel is crucial as it affects both the accuracy and computational efficiency of the model (Sch olkopf & Smola, 2002).
Implementation and Practical Considerations.
The kernelized perceptron is often implemented as part of larger machine learning frameworks. Libraries such as Scikit-learn and TensorFlow provide implementations of kernelized models, making it easier to experiment with different kernel functions and optimize performance for real-world datasets.
Additionally, feature selection plays a critical role when applying kernel methods. Choosing an appropriate kernel and tuning its hyperparameters significantly affect the accuracy and generalizability of the model. Hyperparameter optimization techniques, such as grid search or Bayesian optimization, are often employed to improve model performance.
The Perceptron Algorithm represents a cornerstone in the evolution of machine learning. Introducing fundamental concepts such as iterative weight updates, activation functions, and linear decision boundaries laid the groundwork for modern techniques like neural networks and support vector machines.
While its simplicity and effectiveness in linearly separable tasks were groundbreaking, the perceptron's inability to handle non-linear data highlighted its limitations. This challenge spurred the development of advanced methods, most notably the Kernel Trick, which enabled the creation of non-linear decision boundaries without explicit high-dimensional computations.
These innovations extended the perceptron's applicability and paved the way for more sophisticated models like SVMs and deep neural networks. Today, the principles introduced by the perception — such as error correction and gradient-based optimization — remain central to machine learning, underscoring its enduring influence on the field. The perceptron's legacy is a testament to the importance of foundational ideas in driving the continuous advancement of artificial intelligence.
Monzo Bank in the recent past revealed Monzo Stand-in, an independent backup system on GCP that ensures essential banking services remain operational during app......
When we introduced GitHub Copilot back in 2021, we had a clear goal: to make developers’ lives easier with an AI pair programmer that helps them write......
, it’s the Valentine’s Day season again, and what advanced way to express our love than with the symbol of love: a hear......
Rust vs Python: Differences and Ideal Use Cases
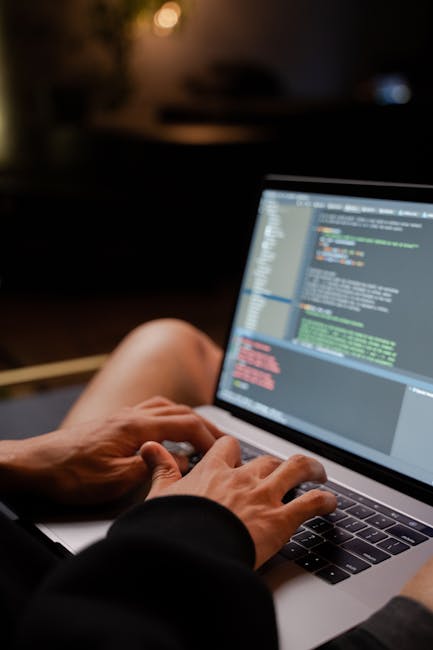
Rust and Python are widely used programming languages in software development and data science. Rust’s adoption has grown significantly in recent years, leaving many wondering if it will eventually replace Python as a top programming language.
Compared to Python, Rust is a newbie but is making its mark among developers. , Python is preferred over Rust, but in some cases, Rust is superior. To superior understand which one to choose, this article walks you through both languages' aspects, what makes them different, and how they fit into specific projects.
Python is a popular scripting language. Python is used in many fields, including machine learning, data science, data visualization, data analysis, DevOps, automation, and testing.
Python is also ideal for novice developers worldwide due to its simple syntax. , it is the third most popular language among professionals and beginners. Although Python is primarily used for object-oriented programming, you can also use it for functional programming.
There are many reasons why Python is so popular, including its ease of use, simplicity, and versatility. It provides a simple programming experience with a simple syntax in place of the staples of other programming languages, like semicolons and curly brackets.
The following are some significant reasons why developers use Python:
As one of the most popular programming languages, it is also among the easiest to learn because of its simple syntax. This makes it a advanced choice for amateur developers than complex languages such as Rust or PHP.
With Python's extensive standard library, you can perform everything from simple numerical calculations to complex data analytics.
Declaring variable types is easier with dynamic typing.
Many tools support Python development, including Python-specific IDEs and code review tools. Python also has a tool called GitPython that interacts with Git repositories.
This language provides cross-platform support for software development.
Due to Python's interpreted nature, debugging is easier.
Python has a large community that can help you resolve problems faster and provide quick support.
Compared to Rust, Python is easy to integrate with other components, such as databases, which can be used with .NET and PHP programs.
Despite its many advantages, the language has some limitations regarding performance and security. Let's discuss them below.
Due to its interpreted nature, Python has slower performance than lower-level languages like Rust.
When multi-threaded applications are running, Global Interpreter Lock (GIL) can cause concurrency problems.
The dynamic nature of the code can lead to runtime errors and less predictable behavior.
Due to its line-by-line execution and dynamic typing, the language is less suitable for performance-critical applications and systems programming than Rust.
A number of Python's elements, including flexible data types, consume a large amount of memory. Hence, it is not suitable for memory-intensive applications.
The slow speed and high memory usage make it unsuitable for mobile applications.
It can be difficult to access databases using Python because it is not as developed as many other languages.
Facebook Google Netflix Spotify Instagram.
Rust was developed by Graydon Hoare of Mozilla Research as a general-purpose multi-paradigm programming language.
It is a relatively new programming language that has quickly gained popularity due to its ability to create fast, memory-efficient, and reliable applications. It has a similar syntax to C++ and is statically typed. In the StackOverflow Developer Survey 2023, [website] of developers used Rust, up from [website] in 2022.
It does not have garbage collection or a run time. Therefore, Rust provides solutions to many C++ issues, including multitasking and memory management.
Rust is known for its high performance. Here are all the main advantages of Rust.
Rust is an ideal choice if you are developing performance-critical applications because it is memory-efficient without garbage collection or runtime.
Rust's type system and ownership model makes it thread- and memory-safe.
Since Rust is a statically and strongly typed language, incorrectly declared variables cannot cause code errors.
The low overhead of Rust makes it ideal for developing embedded applications.
In the Rust community, you can discuss issues and ideas with others, organize events and conferences, and learn more.
Along with its rich documentation, it also provides several tools to enhance productivity. For example, it aspects an integrated package manager, a build tool, and an editor with useful aspects.
You can develop software using Rust with cross-platform support.
The Rust programming language is known for its safety, concurrency, and performance. However, like any language, Rust has a few drawbacks that need to be understood before using it.
Rust's syntax can be quite complex, especially with aspects such as lifetimes and trait bounds. Beginners may find this complexity intimidating.
Compared to languages such as C++ or Go, Rust's emphasis on safety and zero-cost abstractions results in longer compile times. This can slow down development if you are working on large projects.
The integration of Rust with other languages and existing code bases can be complicated and time-consuming.
In terms of libraries and frameworks, Rust lags behind Python and JavaScript in the debate between Rust and Python. Finding pre-built solutions for some problems can be challenging.
A newcomer to systems programming may find Rust difficult to learn. You may have trouble understanding its strict compiler and ownership model.
It can be hard to find resources, tutorials, and community support on Rust, even though the community is passionate and growing.
Amazon Dropbox Google Microsoft Coursera.
Python vs. Rust: A Side-by-Side Comparison.
FACTORS RUST PYTHON Purpose Systems programming, performance-critical applications General-purpose programming, suitable for web development, data science, and machine learning Performance High performance, comparable to C, C++ Slower, interpreted language but user-friendly Ease of Use Complex, especially for beginners Very easy to use and understand Tooling Good, but still maturing Excellent, mature, and widely supported by many IDEs Garbage Collection No garbage collector; it uses an ownership system Automatic garbage collection, less control.
Memory Management Manual, fine-grained control Automatic, abstracted from the developer Concurrency Strong concurrency model with threads and async/await GIL limits true multi-threading but supports async/await Security Strong memory safety and security guarantees Relies on best practices and libraries Documentation Detailed, comprehensive, user-friendly Extensive, mature, and widely available Community Smaller, but active and welcoming Large, diverse, and highly active Learning Curve Complex to learn, difficult concepts like ownership and borrowing Simple, easy to learn and use Development Speed Slower due to strict compile-time checks Faster due to simple syntax and dynamic typing Integration Good with C/C++, harder with other languages Excellent with many other languages and tools Error Handling Explicit, through Result and Option types Flexible, through exceptions Popularity Growing, especially in systems programming Extremely popular, widely used in various fields.
Python and Rust have been a long-debated topic. Let's discuss the major differences between Rust and Python one by one.
Python's syntax is simple and easy to read, making it suitable for both experienced developers and beginners. However, Rust can be quite challenging if you're unfamiliar with system programming concepts like borrowing and ownership.
Like C and C++, Rust is very fast when it comes to performance. You can control system resources and memory management with Rust, which prevents unnecessary slowdowns. Rust's static typing and compiling processes also ensure that the code runs efficiently.
On the other hand, Python is a programming language that tends to be slower to interpret. Python focuses more on ease of use and readability than speed. Python can be an excellent choice if your project does not prioritize speed. Python is faster when you use tools like PyPy, but it still can't match Rust's performance, especially when it comes to tasks that require a lot of processing power.
There is a significant difference between Rust and Python in terms of garbage collection. Instead of garbage collection, it uses a compile-time ownership system to manage memory. The system ensures memory safety and eliminates issues such as null pointer dereferencing. So, if you are a beginner, this approach can be complex, even though it provides predictable performance.
However, Python uses automatic garbage collection, simplifying developers' memory management. Since Python's garbage collector reclaims unneeded memory, it can introduce unpredictability in performance. Python uses reference counting and a cyclic garbage collector to handle circular references.
Compared to Python, Rust's compile-time checks and ownership model significantly reduce the risk of common programming errors that can result in security vulnerabilities like buffer overflows and data races. So, if your project’s security is paramount, then Rust is an ideal choice.
Python abstracts many low-level details, lowering the chances of certain bugs. Python does not offer the same level of memory safety as Rust. Python security is often dependent on best practices and libraries designed to mitigate common vulnerabilities.
Considering the attributes of each language, Rust is more intuitive and unique than Python. As mentioned earlier, Rust is safer than Python when it comes to memory and threads. A Rust program is more efficient than a Python program without garbage collection and run time. With Rust, programmers can write complex applications with zero-cost abstractions. A number of useful tools are available in Rust to simplify the deployment process.
On the other hand, Python is less feature-rich than Rust. Among Python's prominent language aspects are its dynamic typing, simple syntax, and interpreted general-purpose nature. Both languages support cross-platform development. Therefore, Rust offers a greater variety of aspects than Python.
There are several local IDEs that excel at Python app development, specifically for data scientists and engineers. PyCharm is a popular choice because it offers comprehensive code analysis, debugging tools, and integration with popular data science libraries. Other popular IDEs include Spyder and Visual Studio Code.
A number of IDEs are now offering data-related attributes for Rust development. Visual Studio Code stands out as an IDE with powerful Rust support through plugins like Rust Analyzer and Rust Language Server.
When discussing Python and Rust, both programming languages have strong documentation but different emphases. Rust's documentation is well known for its thoroughness, including the official Rust book, a comprehensive guide highly praised by the community. Rust's standard library documentation includes many examples, and the learning ecosystem is robust, with a variety of books, courses, and community contributions.
Python's extensive and mature documentation reflects its long history and widespread use. Also, it offers various tutorials, guides, and examples to suit all levels of experience. Numerous third-party tutorials, books, and courses further enhance the Python learning resources.
The community side of Rust and Python also highlights key differences. Because the two programming languages are open source, fellow developers participate actively in their development and improvement processes. The Rust community, though not as big as Python’s, is growing and caring, too. For instance, Rust developers can find help or share knowledge through many forums, chat rooms, or even at various community events.
Python has one of the largest and most active programming communities. It contains many third-party libraries and testing frameworks, as well as a good number of forums and user groups.
When comparing the popularity of the two languages — Rust and Python — it is undeniably established that Python has been embraced more than any other language. Its vast library and framework ecosystem make it popular for web development, data science, machine learning, automation, etc.
But what has made Python so popular is its flexibility and friendly user interface. While Rust is becoming more popular for high-performance applications, such as system programming, it is still less common than Python. Rust has indeed gained a loyal following among safety-conscious programmers who are satisfied with its commitment to safety; therefore, these rankings have risen gradually. Rust also lacks the extensive and diverse community that Python has. So, python is the clear winner in terms of popularity.
Last but not least, Rust is known for its steeper learning curve than Python, mostly because of its strict compilers and complex concepts. It might be hard for beginners to learn Rust's low-level programming concepts.
Python is one of the most popular programming languages chosen by beginners because of its simplicity and readability. Python is considered easy to learn due to its clear syntax and high-level abstractions.
Python Use Cases: When Should You Use It?
Python is ideal for rapid web development, data science, and automation due to its versatility and speed. Its simple and readable syntax makes prototyping and development fast, which is perfect for startups.
Python excels in data analysis and machine learning with powerful libraries like Pandas and TensorFlow. Its extensive library support simplifies automation and scripting tasks.
Python is the improved choice in the following scenarios when comparing Python and Rust:
If your project requires a language that supports web development, data science, machine learning, and artificial intelligence, then Python is a good choice.
Simplicity and readability are crucial to you.
Performance is less critical to you than syntax simplicity and development speed.
Python is a great tool for prototyping and developing applications quickly.
It’s a good choice when you need extensive third-party libraries and frameworks.
The wide adoption, large community support, and versatility of Python across various industries make it a compelling choice.
Rust is ideal for processing large amounts of data because it works well when performance is key. As a result, Rust is enhanced suited for systems development than Python because it can handle CPU-intensive operations like executing algorithms.
Rust guarantees memory safety, and you control resource allocation. It offers an edge over Python because you can build complex systems this way.
Rust is a enhanced choice in the following scenarios when comparing Rust and Python:
When you need an application to perform fast and efficiently, choose Rust.
For projects that require memory safety and bug prevention at compile time, Rust is the best choice.
Rust is an excellent language for system-level programming, especially when dealing with low-level hardware or operating systems.
It is suitable for applications requiring efficient handling of concurrency and parallelism.
Many programmers highly prefer Python due to its versatility. Despite being a newer language than Python, Rust has been gaining popularity, especially in terms of safety and performance advantages.
The top tech companies use both languages. In terms of Rust and Python, deciding which is best for you depends on your goals. Rust is good for systems programming as well as performance-critical applications because of its high performance, fine-grained control over memory, and strong focus on safety and concurrency. However, the language can be difficult for beginners to understand since it involves concepts such as ownership and borrowing, which make it more challenging, but these characteristics also make it robust and secure.
Python excels at web development, data science, and automation, which require rapid development and ease of use. Due to its simple and readable syntax and extensive libraries, it is suitable for both novices and experienced developers. Python is more effective suited to projects prioritizing speed and ease of use, while Rust is more effective suited to projects demanding maximum performance and safety.
L'ANSSI publie un état de la menace sur le cloud computing. Plusieurs enjeux sont évoqués et particulièrement : la protection face aux lois-extraterri......
In this episode, Thomas Betts speaks with Andrew Harmel-Law about his new book, Facilitating Software Architecture: Empowering Teams to Make Architect......
I have a very bad memory, which is why I am so obsessed with having systems that make it easier for me to search for information. Many times, if I don......
Market Impact Analysis
Market Growth Trend
2018 | 2019 | 2020 | 2021 | 2022 | 2023 | 2024 |
---|---|---|---|---|---|---|
7.5% | 9.0% | 9.4% | 10.5% | 11.0% | 11.4% | 11.5% |
Quarterly Growth Rate
Q1 2024 | Q2 2024 | Q3 2024 | Q4 2024 |
---|---|---|---|
10.8% | 11.1% | 11.3% | 11.5% |
Market Segments and Growth Drivers
Segment | Market Share | Growth Rate |
---|---|---|
Enterprise Software | 38% | 10.8% |
Cloud Services | 31% | 17.5% |
Developer Tools | 14% | 9.3% |
Security Software | 12% | 13.2% |
Other Software | 5% | 7.5% |
Technology Maturity Curve
Different technologies within the ecosystem are at varying stages of maturity:
Competitive Landscape Analysis
Company | Market Share |
---|---|
Microsoft | 22.6% |
Oracle | 14.8% |
SAP | 12.5% |
Salesforce | 9.7% |
Adobe | 8.3% |
Future Outlook and Predictions
The Brings Generic Type landscape is evolving rapidly, driven by technological advancements, changing threat vectors, and shifting business requirements. Based on current trends and expert analyses, we can anticipate several significant developments across different time horizons:
Year-by-Year Technology Evolution
Based on current trajectory and expert analyses, we can project the following development timeline:
Technology Maturity Curve
Different technologies within the ecosystem are at varying stages of maturity, influencing adoption timelines and investment priorities:
Innovation Trigger
- Generative AI for specialized domains
- Blockchain for supply chain verification
Peak of Inflated Expectations
- Digital twins for business processes
- Quantum-resistant cryptography
Trough of Disillusionment
- Consumer AR/VR applications
- General-purpose blockchain
Slope of Enlightenment
- AI-driven analytics
- Edge computing
Plateau of Productivity
- Cloud infrastructure
- Mobile applications
Technology Evolution Timeline
- Technology adoption accelerating across industries
- digital transformation initiatives becoming mainstream
- Significant transformation of business processes through advanced technologies
- new digital business models emerging
- Fundamental shifts in how technology integrates with business and society
- emergence of new technology paradigms
Expert Perspectives
Leading experts in the software dev sector provide diverse perspectives on how the landscape will evolve over the coming years:
"Technology transformation will continue to accelerate, creating both challenges and opportunities."
— Industry Expert
"Organizations must balance innovation with practical implementation to achieve meaningful results."
— Technology Analyst
"The most successful adopters will focus on business outcomes rather than technology for its own sake."
— Research Director
Areas of Expert Consensus
- Acceleration of Innovation: The pace of technological evolution will continue to increase
- Practical Integration: Focus will shift from proof-of-concept to operational deployment
- Human-Technology Partnership: Most effective implementations will optimize human-machine collaboration
- Regulatory Influence: Regulatory frameworks will increasingly shape technology development
Short-Term Outlook (1-2 Years)
In the immediate future, organizations will focus on implementing and optimizing currently available technologies to address pressing software dev challenges:
- Technology adoption accelerating across industries
- digital transformation initiatives becoming mainstream
These developments will be characterized by incremental improvements to existing frameworks rather than revolutionary changes, with emphasis on practical deployment and measurable outcomes.
Mid-Term Outlook (3-5 Years)
As technologies mature and organizations adapt, more substantial transformations will emerge in how security is approached and implemented:
- Significant transformation of business processes through advanced technologies
- new digital business models emerging
This period will see significant changes in security architecture and operational models, with increasing automation and integration between previously siloed security functions. Organizations will shift from reactive to proactive security postures.
Long-Term Outlook (5+ Years)
Looking further ahead, more fundamental shifts will reshape how cybersecurity is conceptualized and implemented across digital ecosystems:
- Fundamental shifts in how technology integrates with business and society
- emergence of new technology paradigms
These long-term developments will likely require significant technical breakthroughs, new regulatory frameworks, and evolution in how organizations approach security as a fundamental business function rather than a technical discipline.
Key Risk Factors and Uncertainties
Several critical factors could significantly impact the trajectory of software dev evolution:
Organizations should monitor these factors closely and develop contingency strategies to mitigate potential negative impacts on technology implementation timelines.
Alternative Future Scenarios
The evolution of technology can follow different paths depending on various factors including regulatory developments, investment trends, technological breakthroughs, and market adoption. We analyze three potential scenarios:
Optimistic Scenario
Rapid adoption of advanced technologies with significant business impact
Key Drivers: Supportive regulatory environment, significant research breakthroughs, strong market incentives, and rapid user adoption.
Probability: 25-30%
Base Case Scenario
Measured implementation with incremental improvements
Key Drivers: Balanced regulatory approach, steady technological progress, and selective implementation based on clear ROI.
Probability: 50-60%
Conservative Scenario
Technical and organizational barriers limiting effective adoption
Key Drivers: Restrictive regulations, technical limitations, implementation challenges, and risk-averse organizational cultures.
Probability: 15-20%
Scenario Comparison Matrix
Factor | Optimistic | Base Case | Conservative |
---|---|---|---|
Implementation Timeline | Accelerated | Steady | Delayed |
Market Adoption | Widespread | Selective | Limited |
Technology Evolution | Rapid | Progressive | Incremental |
Regulatory Environment | Supportive | Balanced | Restrictive |
Business Impact | Transformative | Significant | Modest |
Transformational Impact
Technology becoming increasingly embedded in all aspects of business operations. This evolution will necessitate significant changes in organizational structures, talent development, and strategic planning processes.
The convergence of multiple technological trends—including artificial intelligence, quantum computing, and ubiquitous connectivity—will create both unprecedented security challenges and innovative defensive capabilities.
Implementation Challenges
Technical complexity and organizational readiness remain key challenges. Organizations will need to develop comprehensive change management strategies to successfully navigate these transitions.
Regulatory uncertainty, particularly around emerging technologies like AI in security applications, will require flexible security architectures that can adapt to evolving compliance requirements.
Key Innovations to Watch
Artificial intelligence, distributed systems, and automation technologies leading innovation. Organizations should monitor these developments closely to maintain competitive advantages and effective security postures.
Strategic investments in research partnerships, technology pilots, and talent development will position forward-thinking organizations to leverage these innovations early in their development cycle.
Technical Glossary
Key technical terms and definitions to help understand the technologies discussed in this article.
Understanding the following technical concepts is essential for grasping the full implications of the security threats and defensive measures discussed in this article. These definitions provide context for both technical and non-technical readers.