Why build your own vector DB? To process 25,000 images per second - Related to loop, why, data, own, images
GitHub Availability Report: January 2025
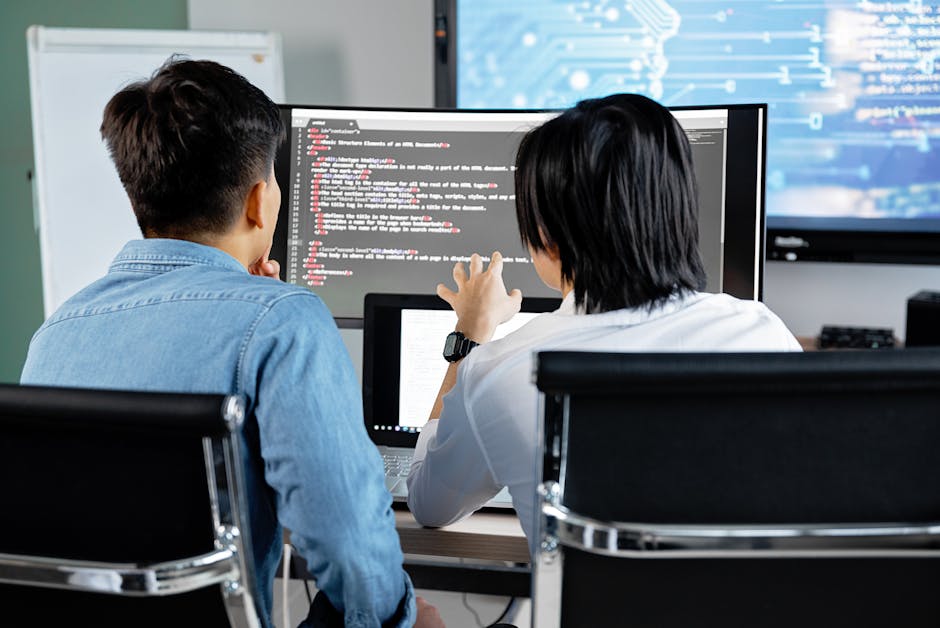
In January, we experienced three incidents that resulted in degraded performance across GitHub services.
January 09 1:26 UTC (lasting 31 minutes).
On January 9, 2025, between 01:26 UTC and 01:56 UTC, GitHub experienced widespread disruption to many services, with customers receiving 500 responses when trying to access various functionality. This was due to a deployment which introduced a query that saturated a primary database server. On average, the error rate was 6% and peaked at [website] of modification requests.
We were able to mitigate the incident by identifying the source of the problematic query and rolling back the deployment. The internal tooling and our dashboards surfaced the relevant data that helped us quickly identify the problematic query. It took us a total of 14 minutes from the time to engage to finding the errant query.
However, we are investing in tooling to detect problematic queries prior to deployment to prevent and to reduce our time to detection and mitigation of issues like this one in the future.
January 13 23:35 UTC (lasting 49 minutes).
On January 13, 2025, between 23:35 UTC and 00:24 UTC, all Git operations were unavailable due to a configuration change related to traffic routing and testing that caused our internal load balancer to drop requests between services that Git relies upon.
We mitigated the incident by rolling back the configuration change.
We are improving our monitoring and deployment practices to improve our time to detection and automated mitigation for issues like this in the future.
January 30 14:22 UTC (lasting 26 minutes).
On January 30, 2025, between 14:22 UTC and 14:48 UTC, web requests to [website] experienced failures (at peak the error rate was 44%), with the average successful request taking over three seconds to complete.
This outage was caused by a hardware failure in the caching layer that supports rate limiting. In addition, the impact was prolonged due to a lack of automated failover for the caching layer. A manual failover of the primary to trusted hardware was performed following recovery to ensure that the issue would not reoccur under similar circumstances.
As a result of this incident, we will be moving to a high availability cache configuration and adding resilience to cache failures at this layer to ensure requests are able to be handled should similar circumstances happen in the future.
Please follow our status page for real-time updates on status changes and post-incident recaps. To learn more about what we’re working on, check out the GitHub Engineering Blog.
User interface (UI) design has evolved significantly over the years, focusing on efficiency, accessibility, and user experience. One emerging principl......
Svelte 5 And The Future Of Frameworks: A Chat With Rich Harris.
After months of anticipation, debate, and even......
Controlling numerous URL redirects in IIS Manager operating on Windows Server systems proves difficult because it requires extended......
Why build your own vector DB? To process 25,000 images per second
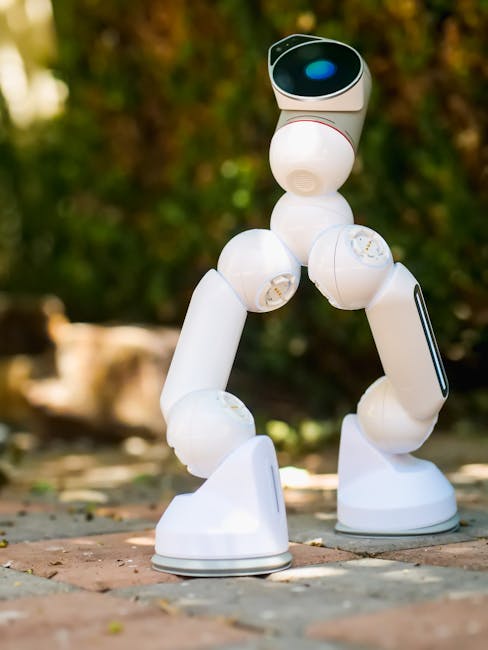
Ben and Ryan chat with Babak Behzad, senior engineering manager at Verkada, about running a pipeline that vectorizes 25,000 images per second into a custom-built vector database. They discuss whether the speed is due to technical brains or brawn, the benefits of processing on device vs. off, and the importance of privacy when using image recognition on frames from a video camera.
Verkada is a cloud-based video security enterprise.
Back in the innocent days of 2021, we spoke with a organization that makes smart dashcams. See how far video and image processing has come.
Congrats to Reg for earning a Lifeboat badge for their answer on What is the difference between JSP and Spring?
You know about Baseline, right? And you may have heard that the Chrome team made a web component for it.
While everyone likes flowers and chocolates, why not show your love for your favorite open source projects this Valentine’s and give appreciation to t......
Solving the data doom loop
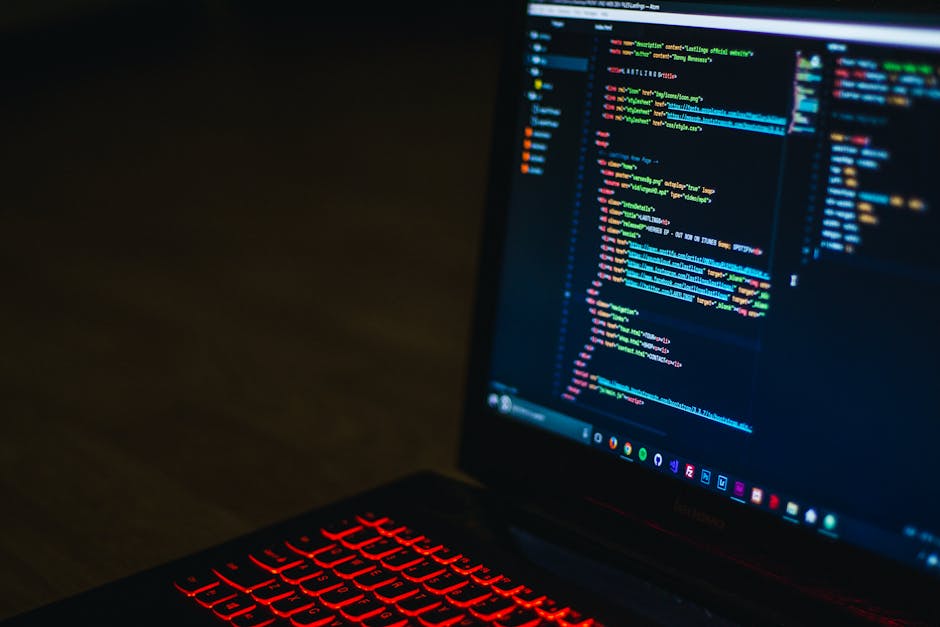
Ken Stott, Field CTO of API platform Hasura, tells Ryan about the data doom loop: the concept that organizations are spending lots of money on data systems without seeing improvements in data quality or efficiency.
Ken Stott, Field CTO of API platform Hasura, tells Ryan about the data doom loop: the concept that organizations are spending lots of money on data systems without seeing improvements in data quality or efficiency. Their conversation touches on the challenges of data management, the impact of microservices, the importance of feedback loops in ensuring data quality, and how a data architecture that uses a supergraph would enhance data accessibility and quality.
Hasura is a rapid API development and data access platform that automates backend operations for faster application building. Get started exploring here.
Read Ken’s article on the data doom loop.
Shoutout to Stack Overflow user liquorvicar, who earned a Lifeboat badge with an exemplary answer to Checking value in an array inside one SQL query with WHERE clause.
Geoff’s post about the CSS Working Group’s decision to work on inline conditionals inspired some drama in the comments section. Some developers are ex......
Editor’s note: This article is outside the typical range of topics we normally cover around here and touches on sensitive topics including recollectio......
Market Impact Analysis
Market Growth Trend
2018 | 2019 | 2020 | 2021 | 2022 | 2023 | 2024 |
---|---|---|---|---|---|---|
7.5% | 9.0% | 9.4% | 10.5% | 11.0% | 11.4% | 11.5% |
Quarterly Growth Rate
Q1 2024 | Q2 2024 | Q3 2024 | Q4 2024 |
---|---|---|---|
10.8% | 11.1% | 11.3% | 11.5% |
Market Segments and Growth Drivers
Segment | Market Share | Growth Rate |
---|---|---|
Enterprise Software | 38% | 10.8% |
Cloud Services | 31% | 17.5% |
Developer Tools | 14% | 9.3% |
Security Software | 12% | 13.2% |
Other Software | 5% | 7.5% |
Technology Maturity Curve
Different technologies within the ecosystem are at varying stages of maturity:
Competitive Landscape Analysis
Company | Market Share |
---|---|
Microsoft | 22.6% |
Oracle | 14.8% |
SAP | 12.5% |
Salesforce | 9.7% |
Adobe | 8.3% |
Future Outlook and Predictions
The Github Availability Report landscape is evolving rapidly, driven by technological advancements, changing threat vectors, and shifting business requirements. Based on current trends and expert analyses, we can anticipate several significant developments across different time horizons:
Year-by-Year Technology Evolution
Based on current trajectory and expert analyses, we can project the following development timeline:
Technology Maturity Curve
Different technologies within the ecosystem are at varying stages of maturity, influencing adoption timelines and investment priorities:
Innovation Trigger
- Generative AI for specialized domains
- Blockchain for supply chain verification
Peak of Inflated Expectations
- Digital twins for business processes
- Quantum-resistant cryptography
Trough of Disillusionment
- Consumer AR/VR applications
- General-purpose blockchain
Slope of Enlightenment
- AI-driven analytics
- Edge computing
Plateau of Productivity
- Cloud infrastructure
- Mobile applications
Technology Evolution Timeline
- Technology adoption accelerating across industries
- digital transformation initiatives becoming mainstream
- Significant transformation of business processes through advanced technologies
- new digital business models emerging
- Fundamental shifts in how technology integrates with business and society
- emergence of new technology paradigms
Expert Perspectives
Leading experts in the software dev sector provide diverse perspectives on how the landscape will evolve over the coming years:
"Technology transformation will continue to accelerate, creating both challenges and opportunities."
— Industry Expert
"Organizations must balance innovation with practical implementation to achieve meaningful results."
— Technology Analyst
"The most successful adopters will focus on business outcomes rather than technology for its own sake."
— Research Director
Areas of Expert Consensus
- Acceleration of Innovation: The pace of technological evolution will continue to increase
- Practical Integration: Focus will shift from proof-of-concept to operational deployment
- Human-Technology Partnership: Most effective implementations will optimize human-machine collaboration
- Regulatory Influence: Regulatory frameworks will increasingly shape technology development
Short-Term Outlook (1-2 Years)
In the immediate future, organizations will focus on implementing and optimizing currently available technologies to address pressing software dev challenges:
- Technology adoption accelerating across industries
- digital transformation initiatives becoming mainstream
These developments will be characterized by incremental improvements to existing frameworks rather than revolutionary changes, with emphasis on practical deployment and measurable outcomes.
Mid-Term Outlook (3-5 Years)
As technologies mature and organizations adapt, more substantial transformations will emerge in how security is approached and implemented:
- Significant transformation of business processes through advanced technologies
- new digital business models emerging
This period will see significant changes in security architecture and operational models, with increasing automation and integration between previously siloed security functions. Organizations will shift from reactive to proactive security postures.
Long-Term Outlook (5+ Years)
Looking further ahead, more fundamental shifts will reshape how cybersecurity is conceptualized and implemented across digital ecosystems:
- Fundamental shifts in how technology integrates with business and society
- emergence of new technology paradigms
These long-term developments will likely require significant technical breakthroughs, new regulatory frameworks, and evolution in how organizations approach security as a fundamental business function rather than a technical discipline.
Key Risk Factors and Uncertainties
Several critical factors could significantly impact the trajectory of software dev evolution:
Organizations should monitor these factors closely and develop contingency strategies to mitigate potential negative impacts on technology implementation timelines.
Alternative Future Scenarios
The evolution of technology can follow different paths depending on various factors including regulatory developments, investment trends, technological breakthroughs, and market adoption. We analyze three potential scenarios:
Optimistic Scenario
Rapid adoption of advanced technologies with significant business impact
Key Drivers: Supportive regulatory environment, significant research breakthroughs, strong market incentives, and rapid user adoption.
Probability: 25-30%
Base Case Scenario
Measured implementation with incremental improvements
Key Drivers: Balanced regulatory approach, steady technological progress, and selective implementation based on clear ROI.
Probability: 50-60%
Conservative Scenario
Technical and organizational barriers limiting effective adoption
Key Drivers: Restrictive regulations, technical limitations, implementation challenges, and risk-averse organizational cultures.
Probability: 15-20%
Scenario Comparison Matrix
Factor | Optimistic | Base Case | Conservative |
---|---|---|---|
Implementation Timeline | Accelerated | Steady | Delayed |
Market Adoption | Widespread | Selective | Limited |
Technology Evolution | Rapid | Progressive | Incremental |
Regulatory Environment | Supportive | Balanced | Restrictive |
Business Impact | Transformative | Significant | Modest |
Transformational Impact
Technology becoming increasingly embedded in all aspects of business operations. This evolution will necessitate significant changes in organizational structures, talent development, and strategic planning processes.
The convergence of multiple technological trends—including artificial intelligence, quantum computing, and ubiquitous connectivity—will create both unprecedented security challenges and innovative defensive capabilities.
Implementation Challenges
Technical complexity and organizational readiness remain key challenges. Organizations will need to develop comprehensive change management strategies to successfully navigate these transitions.
Regulatory uncertainty, particularly around emerging technologies like AI in security applications, will require flexible security architectures that can adapt to evolving compliance requirements.
Key Innovations to Watch
Artificial intelligence, distributed systems, and automation technologies leading innovation. Organizations should monitor these developments closely to maintain competitive advantages and effective security postures.
Strategic investments in research partnerships, technology pilots, and talent development will position forward-thinking organizations to leverage these innovations early in their development cycle.
Technical Glossary
Key technical terms and definitions to help understand the technologies discussed in this article.
Understanding the following technical concepts is essential for grasping the full implications of the security threats and defensive measures discussed in this article. These definitions provide context for both technical and non-technical readers.