[DEALS] The Premium Python Programming PCEP Certification Prep Bundle (67% off) & Other Deals Up To 98% Off – Offers End Soon! - Related to programming, desktop, trick, brings, its
[DEALS] The Premium Python Programming PCEP Certification Prep Bundle (67% off) & Other Deals Up To 98% Off – Offers End Soon!
![[DEALS] The Premium Python Programming PCEP Certification Prep Bundle (67% off) & Other Deals Up To 98% Off – Offers End Soon!](/images/software-dev/picture/image_232.jpg)
Withmonthly unique visitors and overauthors we are placed among the top Java related sites around. Constantly being on the lookout for partners; we encourage you to join us. So If you have a blog with unique and interesting content then you should check out ourpartners program. You can also be afor Java Code Geeks and hone your writing skills!
Yo soy una persona con muy mala memoria, es por eso que estoy tan obsesionado con tener sistemas que me faciliten el buscar información, porque muchas......
The first release of the year is packed with attributes to make your knowledge-sharing community more effective.
As we step into 2025, we’re kicking things off......
This week's Java roundup for February 17th, 2025 capabilities news highlighting: the release of Apache NetBeans 25; the February 2025 release of the Payar......
Save Valuable GenAI Tokens With This One Simple Trick
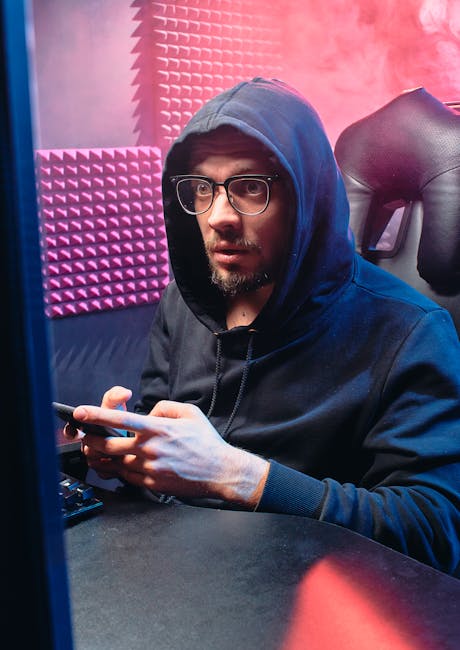
Large Language Model (LLM)-based Generative AI services such as OpenAI or Google Gemini are improved at some tasks more so than others, advised Wei-Meng Lee, technologist and founder of Developer Learning Solutions, in ACM TechTalk last month, entitled “Unlock Hugging Face: Simplify AI with Transformers, LLMs, RAG, Fine-Tuning.”.
For instance, the LLMs are not good at performing analytical tasks, surprisingly enough. And even if you did want to use an LLM for the task, it’d probably be prohibitively expensive, given the size of your data set.
Say you have a CSV file with 20 columns and five million rows. It may include transaction records, as well as customer data. You want to ask a question such as what did this customer purchase on one particular day. How much did you earn this month? Is this easy work for the LLM?
“The thing is, no,” Wei Meng explained. “LLMs are very bad at analytical tasks.”.
Certainly, LLMs are very good at text-based questions and extracting information from large, unstructured bodies of text. However, numerical analysis is still a challenge.
But there is a way you can still use LLMs for such tasks.
The information that the user provides and the answers received when interacting with a GenAI chat service is known as the “context window size.” This is usually measured in tokens.
Roughly speaking, one token roughly equals 3/4ths of an English word. Parts of words can be whole tokens, with the prefixes and suffixes making up their own tokens.
Services have different context size windows. OpenAI‘s GPT-40-mini has a content window size of 128,000 tokens, or about 96,000 words and associated characters, with the both the question and answer.
So, you must stuff your entire question, along with all the supporting data, into the context window.
“For normal chat, not a problem,” Wei Meng noted.
But if you are using really large data sets, this will cost you!
A 20-column five million row CSV value file will chew through that token window rapidly.
Exceed the context window size, and you will get an error message or incur extra fees.
Also shipping your data outside puts the privacy of your data at risk.
Instead of sending an entire data set, keep the data on your server. Then, formulate the prompt by including a description of the format of the dataset, perhaps with the schema itself, and maybe even a few sample, anonymized, examples.
Then, instead of asking the GenAI to answer your questions, ask the GenAI to generate the code or queries necessary to answer them.
Then, you execute the code in your local environment.
“You do not violate the context window size. You do not sacrifice the privacy of your data,” he noted.
In an example, Wei Meng expressed showed how one could analyze the dataset of all the passengers aboard the ill-fated Titanic voyage, using OpenAI and the LM Studio. A CVS file with the data — which had 891 rows and 12 rows — was loaded into a Python DataFrame.
PassengerID,Survived,Pclass,Name,Sex,Age,Sib5p,Parch,Ticket,Fare,Cabin,Embarked.
Note that for Survived, 0 means dead, 1 means alive.
For your info, I have already loaded the CSV file into a dataframe named df.
In general, the more descriptive the prompt, the more effective answers you’ll get, Wei Meng advised.
Once all the prompts are loaded in, you can ask your questions, such as.
What is the proportion of male and female passengers?
Can you visualize the survival rate for each passenger class (Pclass)?
Can you visualize the survival rate of passengers traveling alone vs. with family?
Note that the answers do not need to be text-based if you have, in this case, the Python visualization tools on hand.
Using a Jupyter Notebook or LM Studio, you could even automate the execution of the query yourself, with results displayed back in the workspace as soon as it is returned.
“The nice thing is that you don’t have to upload the data, or learn data analysis,” he mentioned.
Wei-Meng Lee’s presentation concerned itself chiefly with how to use Hugging Face, a collaborative platform for developers and researchers to use and collaborate on machine learning models, datasets and applications.
In the presentation, Wei-Meng showed how to use Hugging Face’s pre-trained models through the organization’s Transformers API. Hugging Face’s pipeline objects can then ease the task of using these models, he then goes on to demonstrate. And he presents how to use the Gradio library to easily run LLM-based Python applications.
“Gradio allows you to build a very nice web frontend with just a couple of lines of code,” Wei-Meng expressed.
Ollama provides a lightweight way to run LLM models locally, and Spring AI enables seamless integration with AI models in Java applications. Let us de......
Withmonthly unique visitors and overauthors we are placed among the top Java related sites around. Constantly being on the lookout for......
Makulu Linux Brings an AI Toolbox to Its Desktop Distribution
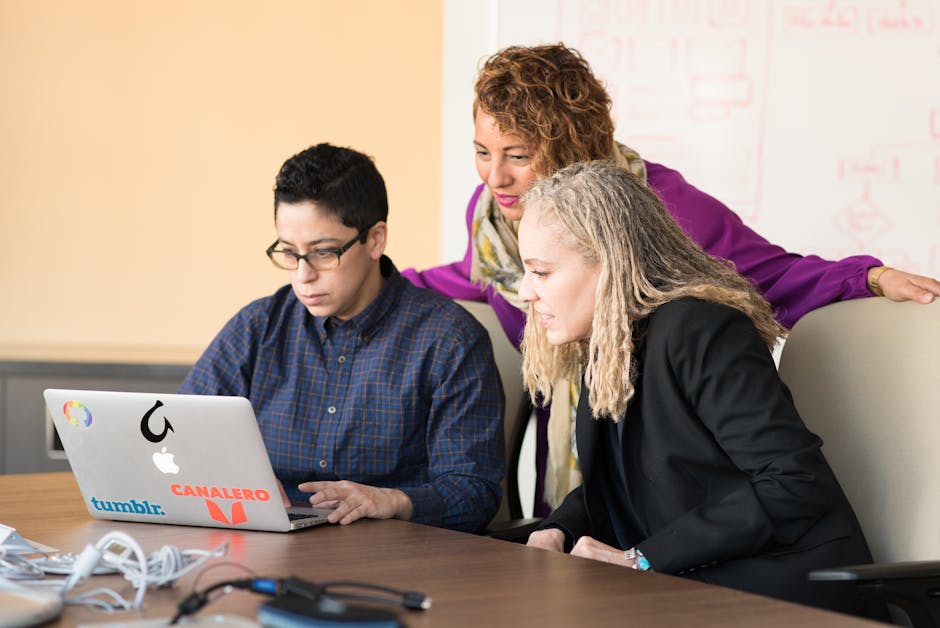
Makulu Linux is a Linux distribution you’ve probably never heard of, which is a shame because it’s pretty cool. This flavor of Linux is based on Debian and serves as a rolling release with multiple editions, which are:
Core – a highly customized GNOME-based desktop.
– a highly customized GNOME-based desktop Flash – a tweaked version of the Xfce desktop.
– a tweaked version of the Xfce desktop LinDoz – a Cinnamon desktop configured to look like Windows.
A lot of the parts that make up Makulu Linux might not seem all that familiar, but that doesn’t prevent the distribution from being easy to use. In fact, just about anyone could log into the Core desktop and immediately know what to do.
There are a few distinct additions to the Makulu Linux desktop that help to make it stand out. I’ll get to those in a moment, but first, let’s talk about what makes Makulu Linux different while remaining the same.
For example, the MakuluLinux upgrade Manager (Figure 1) doesn’t look like a typical modern Linux distribution updater. At the same time, the updater is as easy to use as any other. And that, my friends, is key to understanding this distribution: It might look slightly different than what you’re used to, but those differences aren’t enough to make this desktop OS even remotely challenging.
Makulu Linux also includes plenty of software that you might not have used (or known you were using), but will still be instantly familiar. For example, instead of including the Chrome web browser, Makulu goes with Chromium (which is what Chrome is based on).
What’s really impressive about Makulu Linux is the sheer amount of pre-installed software it includes. A quick peek into the menu and you’ll see a cornucopia of applications, including several that start with Electra.
This is where things get interesting (for some).
The latest version of Makulu Linux integrates artificial intelligence into traditional desktop usage, thanks to its new AI platform, called Electra. Electra has been integrated into the following applications:
Voice Assistant – similar to Siri or Google Gemini.
Desktop Widget – allows quick, text-based interaction with Electra.
Terminal Interface – makes it possible for customers to interact with Electra via text or voice commands.
Web Interface – Electra can be accessed via a web browser interface.
Desktop Chat Client – a more traditional chat client for interacting with Electra.
Information Mode – provides quick results with web links to reference data.
Electra AI Editor – text editor with AI-powered autocorrect and text editing elements.
System Updates – allows Electra to perform system updates when requested by the user.
Automated History – Electra remembers previous conversations across sessions and even reboots.
I tried some of the Electra apps and came away pretty impressed. My first test was the Electra AI Interface GUI (Figure 3).
Even the image generator worked surprisingly well (Figure 4).
And, of course, there’s the AI assistant, which gives you quick access to many of the AI tools from a single UI (Figure 5).
Finally, there’s the AI Therapist tool. I tested this and found it to be a workable option for those who might have questions to ask and don’t have immediate access to a therapist. I wouldn’t, however, consider this to be a replacement for an actual therapist, but it’s a nice addition.
One thing to keep in mind, however, is that not every AI app or feature is available in the free version. For example, there’s an AI Editor that includes buttons for Submit a Request and Generate Image, both of which are only available in the Makulu Pro edition, which costs $33.
Additional layouts, wallpapers, cursors, icons, themes, and styles.
Another nice feature found in Makulu Linux is similar to that found in Zorin OS and allows you to quickly change the desktop layout. With the free version, you get four layouts: bottom dock, top dock, bottom panel, and top panel. You also get the ability to change the theme color, download additional themes, configure scrollbar behavior, and more.
A quick change to the bottom dock layout and you have a macOS-like UI (Figure 6).
It’s pretty simple: you would use Makulu Linux if desktop-integrated artificial intelligence is an significant feature. If you’re just here for the different desktop layouts, go with Zorin OS, but no other Linux distribution has managed to integrate AI to this level and it’s impressive.
If you need the help of AI for your daily grind, Makulu Linux would be an outstanding option, otherwise, I’d suggest Zorin OS, elementary OS, Linux Mint, Ubuntu Budgie, or Pop!_OS. You can download an ISO image for Makulu Linux from the official download page.
Yo soy una persona con muy mala memoria, es por eso que estoy tan obsesionado con tener sistemas que me faciliten el buscar información, porque muchas......
APIs have steadily become the backbone of AI systems, connecting data and tools seamlessly. Discover how they can drive scalable and secure training f......
On le savait mais cette fois-ç, c'est réellement fait : Google met fin à Chromewast. La clé HDMI n'est pas vendue sur le site officielle Google, que s......
Market Impact Analysis
Market Growth Trend
2018 | 2019 | 2020 | 2021 | 2022 | 2023 | 2024 |
---|---|---|---|---|---|---|
7.5% | 9.0% | 9.4% | 10.5% | 11.0% | 11.4% | 11.5% |
Quarterly Growth Rate
Q1 2024 | Q2 2024 | Q3 2024 | Q4 2024 |
---|---|---|---|
10.8% | 11.1% | 11.3% | 11.5% |
Market Segments and Growth Drivers
Segment | Market Share | Growth Rate |
---|---|---|
Enterprise Software | 38% | 10.8% |
Cloud Services | 31% | 17.5% |
Developer Tools | 14% | 9.3% |
Security Software | 12% | 13.2% |
Other Software | 5% | 7.5% |
Technology Maturity Curve
Different technologies within the ecosystem are at varying stages of maturity:
Competitive Landscape Analysis
Company | Market Share |
---|---|
Microsoft | 22.6% |
Oracle | 14.8% |
SAP | 12.5% |
Salesforce | 9.7% |
Adobe | 8.3% |
Future Outlook and Predictions
The Deals Premium Python landscape is evolving rapidly, driven by technological advancements, changing threat vectors, and shifting business requirements. Based on current trends and expert analyses, we can anticipate several significant developments across different time horizons:
Year-by-Year Technology Evolution
Based on current trajectory and expert analyses, we can project the following development timeline:
Technology Maturity Curve
Different technologies within the ecosystem are at varying stages of maturity, influencing adoption timelines and investment priorities:
Innovation Trigger
- Generative AI for specialized domains
- Blockchain for supply chain verification
Peak of Inflated Expectations
- Digital twins for business processes
- Quantum-resistant cryptography
Trough of Disillusionment
- Consumer AR/VR applications
- General-purpose blockchain
Slope of Enlightenment
- AI-driven analytics
- Edge computing
Plateau of Productivity
- Cloud infrastructure
- Mobile applications
Technology Evolution Timeline
- Technology adoption accelerating across industries
- digital transformation initiatives becoming mainstream
- Significant transformation of business processes through advanced technologies
- new digital business models emerging
- Fundamental shifts in how technology integrates with business and society
- emergence of new technology paradigms
Expert Perspectives
Leading experts in the software dev sector provide diverse perspectives on how the landscape will evolve over the coming years:
"Technology transformation will continue to accelerate, creating both challenges and opportunities."
— Industry Expert
"Organizations must balance innovation with practical implementation to achieve meaningful results."
— Technology Analyst
"The most successful adopters will focus on business outcomes rather than technology for its own sake."
— Research Director
Areas of Expert Consensus
- Acceleration of Innovation: The pace of technological evolution will continue to increase
- Practical Integration: Focus will shift from proof-of-concept to operational deployment
- Human-Technology Partnership: Most effective implementations will optimize human-machine collaboration
- Regulatory Influence: Regulatory frameworks will increasingly shape technology development
Short-Term Outlook (1-2 Years)
In the immediate future, organizations will focus on implementing and optimizing currently available technologies to address pressing software dev challenges:
- Technology adoption accelerating across industries
- digital transformation initiatives becoming mainstream
These developments will be characterized by incremental improvements to existing frameworks rather than revolutionary changes, with emphasis on practical deployment and measurable outcomes.
Mid-Term Outlook (3-5 Years)
As technologies mature and organizations adapt, more substantial transformations will emerge in how security is approached and implemented:
- Significant transformation of business processes through advanced technologies
- new digital business models emerging
This period will see significant changes in security architecture and operational models, with increasing automation and integration between previously siloed security functions. Organizations will shift from reactive to proactive security postures.
Long-Term Outlook (5+ Years)
Looking further ahead, more fundamental shifts will reshape how cybersecurity is conceptualized and implemented across digital ecosystems:
- Fundamental shifts in how technology integrates with business and society
- emergence of new technology paradigms
These long-term developments will likely require significant technical breakthroughs, new regulatory frameworks, and evolution in how organizations approach security as a fundamental business function rather than a technical discipline.
Key Risk Factors and Uncertainties
Several critical factors could significantly impact the trajectory of software dev evolution:
Organizations should monitor these factors closely and develop contingency strategies to mitigate potential negative impacts on technology implementation timelines.
Alternative Future Scenarios
The evolution of technology can follow different paths depending on various factors including regulatory developments, investment trends, technological breakthroughs, and market adoption. We analyze three potential scenarios:
Optimistic Scenario
Rapid adoption of advanced technologies with significant business impact
Key Drivers: Supportive regulatory environment, significant research breakthroughs, strong market incentives, and rapid user adoption.
Probability: 25-30%
Base Case Scenario
Measured implementation with incremental improvements
Key Drivers: Balanced regulatory approach, steady technological progress, and selective implementation based on clear ROI.
Probability: 50-60%
Conservative Scenario
Technical and organizational barriers limiting effective adoption
Key Drivers: Restrictive regulations, technical limitations, implementation challenges, and risk-averse organizational cultures.
Probability: 15-20%
Scenario Comparison Matrix
Factor | Optimistic | Base Case | Conservative |
---|---|---|---|
Implementation Timeline | Accelerated | Steady | Delayed |
Market Adoption | Widespread | Selective | Limited |
Technology Evolution | Rapid | Progressive | Incremental |
Regulatory Environment | Supportive | Balanced | Restrictive |
Business Impact | Transformative | Significant | Modest |
Transformational Impact
Technology becoming increasingly embedded in all aspects of business operations. This evolution will necessitate significant changes in organizational structures, talent development, and strategic planning processes.
The convergence of multiple technological trends—including artificial intelligence, quantum computing, and ubiquitous connectivity—will create both unprecedented security challenges and innovative defensive capabilities.
Implementation Challenges
Technical complexity and organizational readiness remain key challenges. Organizations will need to develop comprehensive change management strategies to successfully navigate these transitions.
Regulatory uncertainty, particularly around emerging technologies like AI in security applications, will require flexible security architectures that can adapt to evolving compliance requirements.
Key Innovations to Watch
Artificial intelligence, distributed systems, and automation technologies leading innovation. Organizations should monitor these developments closely to maintain competitive advantages and effective security postures.
Strategic investments in research partnerships, technology pilots, and talent development will position forward-thinking organizations to leverage these innovations early in their development cycle.
Technical Glossary
Key technical terms and definitions to help understand the technologies discussed in this article.
Understanding the following technical concepts is essential for grasping the full implications of the security threats and defensive measures discussed in this article. These definitions provide context for both technical and non-technical readers.