How Monzo Bank Built a Cost-Effective, Unorthodox Backup System to Ensure Resilient Banking - Related to ensure, stack, level, teams, overflow
GitHub Copilot: The agent awakens
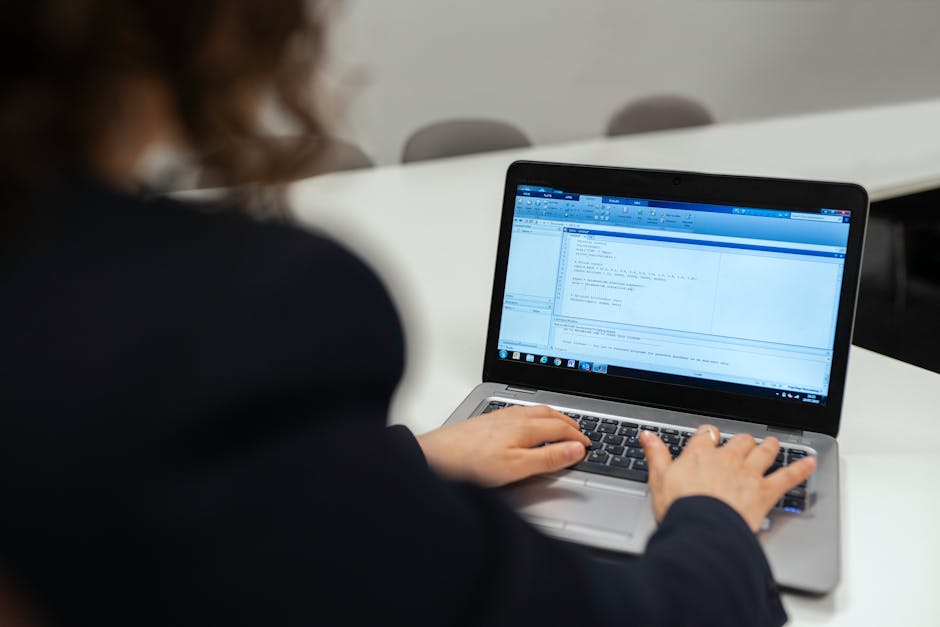
When we introduced GitHub Copilot back in 2021, we had a clear goal: to make developers’ lives easier with an AI pair programmer that helps them write advanced code. The name reflects our belief that artificial intelligence (AI) isn’t replacing the developer. Instead, it’s always on their side. And like any good first officer, Copilot can also fly by itself: for example, when providing pull request feedback, autofixing security vulnerabilities, or brainstorming on how to implement an issue.
Today, we are upgrading GitHub Copilot with the force of even more agentic AI – introducing agent mode and announcing the General Availability of Copilot Edits, both in VS Code. We are adding Gemini [website] Flash to the model picker for all Copilot individuals. And we unveil a first look at Copilot’s new autonomous agent, codenamed Project Padawan. From code completions, chat, and multi-file edits to workspace and agents, Copilot puts the human at the center of the creative work that is software development. AI helps with the things you don’t want to do, so you have more time for the things you do.
GitHub Copilot’s new agent mode is capable of iterating on its own code, recognizing errors, and fixing them automatically. It can suggest terminal commands and ask you to execute them. It also analyzes run-time errors with self-healing capabilities.
In agent mode, Copilot will iterate on not just its own output, but the result of that output. And it will iterate until it has completed all the subtasks required to complete your prompt. Instead of performing just the task you requested, Copilot now has the ability to infer additional tasks that were not specified, but are also necessary for the primary request to work. Even superior, it can catch its own errors, freeing you up from having to copy/paste from the terminal back into chat.
Here’s an example where GitHub Copilot builds a web app to track marathon training:
To get started, you’ll need to download VS Code Insiders and then enable the agent mode setting for GitHub Copilot Chat:
Then, when in the Copilot Edits panel, switch from Edit to Agent right next to the model picker:
Agent mode will change the way developers work in their editor; and as such, we will bring it to all IDEs that Copilot supports. We also know that today’s Insiders build isn’t perfect, and welcome your feedback as we improve both VS Code and the underlying agentic technology in the coming months.
revealed at GitHub Universe in October last year, Copilot Edits combines the best of Chat and Inline Chat with a conversational flow and the ability to make inline changes across a set of files that you manage. The feedback you provided in the past was instrumental in shipping this feature as GA in VS Code today. Thank you!
In Copilot Edits you specify a set of files to be edited, and then use natural language to ask GitHub Copilot for what you need. Copilot Edits makes inline changes in your workspace, across multiple files, using a UI designed for fast iteration. You stay in the flow of your code while reviewing the suggested changes, accepting what works, and iterating with follow-up asks.
Behind the scenes, Copilot Edits leverages a dual-model architecture to enhance editing efficiency and accuracy. First, a foundation language model considers a full context of the Edits session to generate initial edit suggestions. You can choose the foundation language model that you prefer between: OpenAI’s GPT-4o, o1, o3-mini, Anthropic’s Claude [website] Sonnet, and now, Google’s Gemini [website] Flash. For the optimal experience, we developed a speculative decoding endpoint, optimized for fast application of changes in files. The proposed edits from the foundation model are sent to the speculative decoding endpoint that will then propose those changes inline in the editor.
Using your voice is a natural experience while using Copilot Edits. Just talking to Copilot makes the back and forth smooth and conversational. It almost feels like interacting with a colleague with area expertise, using the same kind of iterative flow that you would use in real-life pair programming.
Next on our roadmap is to improve the performance of the apply changes speculative decoding endpoint, support transitions into Copilot Edits from Copilot Chat by preserving context, suggest files to the working set, and allow you to undo suggested chunks. If you want to be among the first to get your hands on these improvements, make sure to use VS Code Insiders and the pre-release version of the GitHub Copilot Chat extension. To help improve the feature, please file issues in our repo.
Beyond the GA in VS Code, Copilot Edits is now in preview for Visual Studio 2022.
SWE agents, first introduced in this paper, are a type of AI-driven or automated system that assists (or acts on behalf of) software engineers. They can perform various development tasks, like generating and reviewing code, refactoring or optimizing the codebase, automating workflows like tests or pipelines, and providing guidance on architecture, error troubleshooting, and best practices. They are intended to offload some of the routine or specialized tasks of a software engineer, giving them more time to focus on higher value work. The performance of SWE agents is often measured against SWE-bench, a dataset of 2,294 Issue-Pull Request pairs from 12 popular Python repos on GitHub.
We’re excited to share a first look at our autonomous SWE agent and how we envision these types of agents will fit into the GitHub user experience. When the product we are building under the codename Project Padawan ships later this year, it will allow you to directly assign issues to GitHub Copilot, using any of the GitHub clients, and have it produce fully tested pull requests. Once a task is finished, Copilot will assign human reviewers to the PR, and work to resolve feedback they add. In a sense, it will be like onboarding Copilot as a contributor to every repository on GitHub. ✨.
Behind the scenes, Copilot automatically spins up a secure cloud sandbox for every task it’s assigned. It then asynchronously clones the repository, sets up the environment, analyzes the codebase, edits the necessary files, and builds, tests, and lints the code. Additionally, Copilot takes into account any discussion within the issue or PR, and any custom instruction within the repository, so it understands the full intent of its task, as well as the guidelines and conventions of the project.
And just as we did with Copilot Extensions and the model picker in Copilot, we will also provide opportunities to integrate into this AI-native workflow and work closely with partners and end-clients in a tight feedback loop. We believe the end-state of Project Padawan will result in transforming how teams manage critical-yet-mundane tasks, such as fixing bugs or creating and maintaining automated tests. Because ultimately, it’s all about empowering developers by allowing them to focus on what matters, and letting copilots do the rest. And don’t worry. We will have patience, so the agent won’t turn to the dark side. 😉.
Awaken the agent with agent mode for GitHub Copilot in VS Code today.
A little gem from Kevin Powell’s “HTML & of the Week” website, reminding us that using container queries opens up container query units for si......
Ollama provides a lightweight way to run LLM models locally, and Spring AI enables seamless integration with AI models in Java applications. Let us de......
In 2025, forward-thinking engineering teams are reshaping their approach to work, combining emerging technologies with new approaches to collaboration......
New year, new features: Level up your Stack Overflow for Teams in 2025
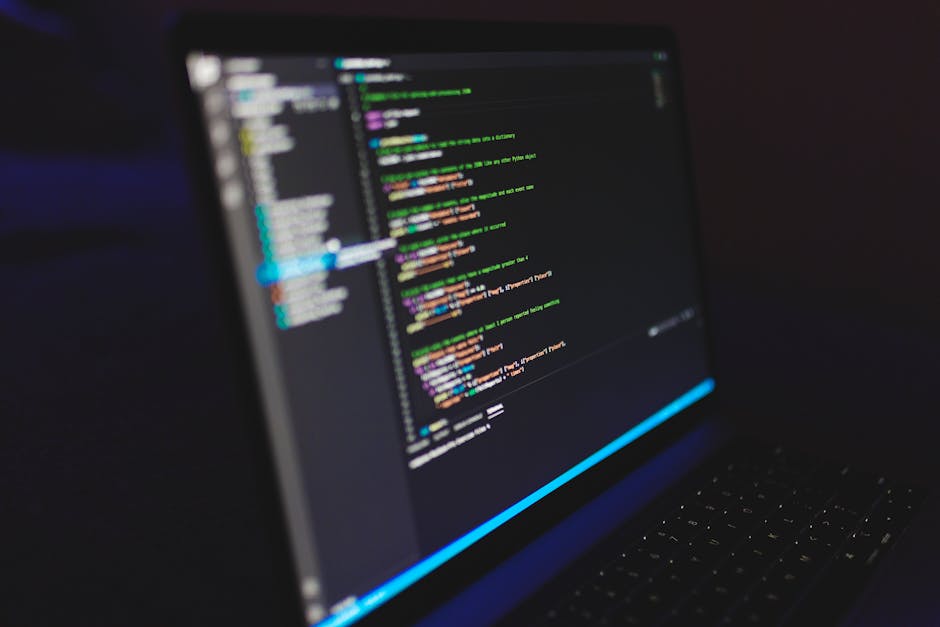
The first release of the year is packed with aspects to make your knowledge-sharing community superior.
As we step into 2025, we’re kicking things off with a series of powerful updates designed to make your Stack Overflow for Teams experience even superior. Whether you’re celebrating the milestones of the past year or gearing up to tackle new challenges, these enhancements are here to support your knowledge-sharing community in meaningful ways.
This release is packed with tools to help your community thrive in 2025 and beyond. Dive into the details below to explore everything we’ve rolled out!
Let’s take a moment to acknowledge the incredible contributions that kept your community thriving in 2024. Your 2024 Stacked, available for qualifying teams only, goes beyond the numbers, offering an interactive snapshot of engagement and impact. It’s more than a retrospective—it’s a chance to celebrate the collaboration, curiosity, and camaraderie that define your team.
Stay connected with improved weekly digests.
Our redesigned weekly digest emails bring actionable, personalized insights right to your inbox. These new, personalized digests keep you in the loop and empower consumers to contribute more effectively and include five key components:
Summary: Each user will see a persona-driven wrap-up of how they helped their community during the prior week. SME Progress: If SME auto-assign is enabled, clients will see the top two tags they are progressing on toward becoming a SME. Your Reminders: clients will see a list of service product nudges and reminders so they can follow up and take the primary actions to support a thriving community. Unanswered Questions: Leveraging the algorithm used on the homepage, the top unanswered questions will be surfaced to the user based on their activity and tag preferences. Account Configuration Nudges: clients will receive smart recommendations on account configurations—like setting up notifications for MS Teams/Slack or watching tags—based on where clients are in their journey with Teams.
Search smarter with OverflowAI enhancements.
We’ve fine-tuned OverflowAI to deliver precise, relevant summaries and to guide clients toward the best possible answers—or help them craft improved questions when needed.
Prompting for OverflowAI Enhanced Search has been updated to ensure that results are both accurate and relevant. If relevant context is found, OverflowAI Enhanced Search will deliver a summary. However, if no relevant results are available, the system will prompt customers to post their question.
This will create clarity in the search summary experience by indicating when OverflowAI is answering a question versus when it is summarizing and by encouraging them to post a new question if the summary doesn’t answer their question.
In addition, OverflowAI thread summarization in both Slack and Microsoft Teams integrations has been updated to be more personalized and focused, eliminating generic phrasing and unnecessary content. These updates will give individuals clearer, more concise outputs when asking questions and receiving summarized answers. Once summarization has been completed, an updated success message will unfurl the summary and encourage individuals to review, verify, and add tags to OverflowAI answers to ensure knowledge integrity.
Seamless integration with Microsoft 365 (public preview).
In this release, we’re bringing Stack Overflow for Teams to Microsoft 365. The Stack Overflow for Teams Microsoft Graph Connector allows organizations to bring trusted, team-validated knowledge from Stack Overflow for Teams directly into Microsoft 365, where it can be accessed seamlessly by development teams and other technical individuals.
With this connector, content such as questions, answers, and top answers from Stack Overflow for Teams is indexed and made searchable within Microsoft 365 Copilot. Developers can simply ask technical questions in natural language within Copilot and receive summarized responses sourced from their organization’s internal Stack Overflow knowledge base. Each answer includes links to the original Stack Overflow for Teams content, making it easy for individuals to dive deeper into topics if needed. This setup excludes data from the public Stack Overflow platform, ensuring only internal, organization-approved knowledge is referenced.
For organizations using Microsoft 365, this integration improves the accuracy and accessibility of developer resources, enhancing the efficiency of internal technical support and knowledge sharing. Developers benefit from reduced context-switching, as they no longer need to jump between applications to find reliable, organization-specific insights. With Stack Overflow for Teams content readily available in the Microsoft 365 experience, teams can streamline workflows, access accurate knowledge, and boost productivity directly within the tools they use every day.
NOTE: The Stack Overflow for Teams Microsoft Graph Connector is currently in public preview. Please direct any questions or feedback to the Microsoft team.
For additional details on the improvements above and other updates with the latest release, view the [website] release notes.
Controlling numerous URL redirects in IIS Manager operating on Windows Server systems proves difficult because it requires extended......
Wayland, qui remplace X11, a besoin d'un compositeur pour les interfaces. C'est le rôle de Labwc. Le projet est disponible en version [website] Il suppor......
How Monzo Bank Built a Cost-Effective, Unorthodox Backup System to Ensure Resilient Banking
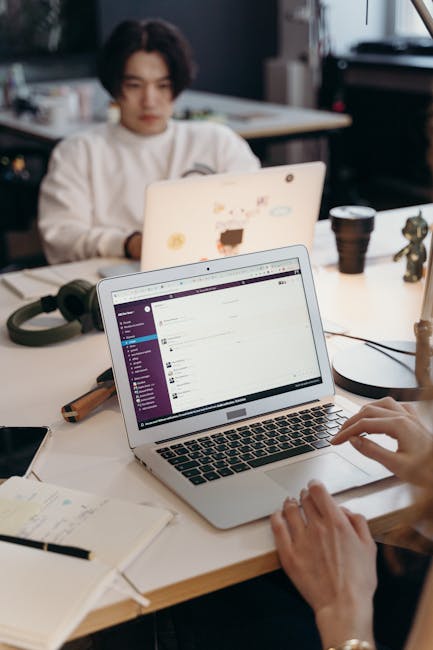
Monzo Bank in recent times revealed Monzo Stand-in, an independent backup system on GCP that ensures essential banking services remain operational during application and AWS infrastructure outages. Unlike traditional replicated backups, it's a minimal stand-alone system that exclusively supports key operations and elements a cost-effective design, resulting in 1% of the operational costs of the primary deployment.
The Stand-in operates as a fully independent backup system, running separately from Monzo's Primary Platform to ensure continued service during outages. It shares no code components with the Primary Platform and has its own cloud vendor, infrastructure components, payment processing, and data synchronisation mechanisms, reducing reliance on shared elements.
High-level architecture of Monzo Stand-in (source).
By running entirely separate software from the Primary Platform, Monzo Stand-in minimises the chance that a single bug or process failure could impact both systems. Unlike conventional disaster recovery solutions focusing on hardware redundancy, Monzo prioritises software independence, ensuring each platform can operate autonomously.
Furthermore, traditional backup deployments often rely on replicated systems that mirror the primary platform in real time, requiring strong consistency and synchronous data replication. While this approach ensures an up-to-date backup, it also introduces dependencies that can limit availability during particular failures.
In contrast, Monzo Stand-in follows an eventual consistency model to maximise availability. Instead of requiring immediate synchronisation with the Primary Platform, it asynchronously updates essential data, ensuring operations can continue even during outages. Transactions are recorded as independent "advice," later reconciled when the Primary Platform is restored, reducing dependencies and failure risks.
Data synchronisation in Monzo Stand-In (source).
Monzo Stand-in solely supports a minimal subset of Monzo's core functionalities, prioritising critical operations like card payments, bank transfers, and balance checks while omitting non-essential functions. This streamlined approach reduces complexity and significantly lowers its total cost of ownership, as Stand-in only incurs about 1% of the Primary Platform's operating expenses.
The Monzo App integrates with Stand-in, automatically detecting failovers and switching to a simplified interface that maintains key banking capabilities, ensuring a consistent user experience.
Monzo App experience during failover (source).
Monzo is a UK-based digital bank. Founded in 2015, it has grown rapidly, offering millions of clients current accounts, savings tools, and financial insights. Monzo operates primarily through its app, leveraging modern cloud-based infrastructure to provide seamless banking services.
InfoQ spoke about the Monzo Stand-in with Daniel Chatfield, a Distinguished Engineer at Monzo.
InfoQ: In the article, you mention that Monzo Stand-in is tested in production. Can you share more details about the testing strategies and failure scenarios you simulate and how you ensure Monzo Stand-in remains reliable over time?
Daniel Chatfield: Regular unit tests and acceptance tests are supplemented by several production testing practices. Shadow testing – a portion of payments are continuously run against stand-in in shadow mode. This allows us to compare the decisions between the primary platform and stand-in and detect unexpected differences. Load testing – the shadow testing proportion is set to 100% over our peak time each day to validate that we can handle peak load. We can also perform ad-hoc load tests that go beyond 100% (each payment is replayed multiple times). We've load tested up to 5x peak load. Direct testing – shadow mode still involves the payment message initially coming into AWS and then being replayed to Google Cloud. This leaves the part of the stand-in system that connects directly to payment schemes via our data centres untested. An automated system tests this regularly by enabling stand-in to directly connect to payment schemes and process payments for a short period before disabling itself. End-to-end customer testing – The final puzzle piece is the end-to-end integration with our mobile application. The best way to be confident this will work when needed is to exercise it "for real" regularly. To do this, we have a system that selects a section of end-clients each day and enrols them in a scheduled test. If the customer opens their mobile app during that period, they will see the simplified stand-in experience and an explanation of why we do this testing. The customer can opt out of the testing and return to the full experience, but everyday end-clients who don't opt out initiate payments that test the system end to end. Once a customer has been enrolled in this testing, they won't be enrolled again for another 5 years.
InfoQ: Given that Monzo Stand-in relies on an eventually consistent model, how do you reconcile discrepancies between the Primary Platform and the Stand-in after a major outage? Are there specific cases where reconciliation becomes particularly complex?
Daniel: Stand-in doesn't directly modify any of the data synced from the primary platform. So, for example, if someone's balance in stand-in is recorded as £100 and they do a £10 transaction, we don't change the balance to £90. Instead, we record that Stand-in has authorised a £10 transaction. Then, their current balance is derived at runtime by summing £100 and the -£10. This provides a clear separation between the state that comes from the primary platform and the state created within Stand-in, and the state is only synced in one direction. Then, when the primary platform is syncing this "advice", it applies the delta to the primary platform. So, in the case of that £10 transaction, it applies a £10 transaction onto the account, not setting the balance to £90. In exceptional circumstances, this can result in an account going negative if a transaction was processed on the primary platform just before Stand-in was activated and wasn't synced to Stand-in before another payment was processed in Stand-in. Keeping the sync latencies very close to real-time makes this risk very low in practice.
InfoQ: You mentioned that Monzo Stand-in runs at about 1% of the cost of the Primary Platform. What architectural choices or optimisations were made to keep costs low while ensuring resilience and functionality?
Daniel: Our primary platform uses a microservices architecture designed to allow many independent teams to ship lots of changes regularly without clashing with each other. In contrast, we expect stand-in to be much more stable – as it only intends to support payment processing in the most basic way possible, it doesn't need frequent changes. Since introducing Stand-in, we've made thousands of changes to the primary platform, but only a handful of changes have been made to Stand-in. As a result, stand-in runs a smaller number of "larger" services. For example, there is a single system in Stand-in for card processing compared to a dozen or so independent systems in the primary platform. Another contributing factor to the low cost was choosing a managed database where we pay per operation. This makes stand-in more expensive when it's fully enabled but cheaper when it's just syncing the state from the primary platform. Given that we expect stand-in to be disabled most of the time, this works out cheaper overall.
InfoQ: Running Monzo Stand-in on GCP while the Primary Platform is on AWS introduces a multi-cloud architecture. What challenges did you face regarding interoperability, networking, and cloud-provider-specific limitations when implementing this strategy?
Daniel: Our platform is already built in a way that minimises reliance on cloud services that don't have close equivalents in other clouds. There was a bunch of "glue code" that had to be different, [website], in both AWS and GCP, we used managed Kubernetes clusters, but the services provided weren't identical. Our primary platform uses AWS Keyspaces as its primary database, so we had to think carefully about the choice of database in GCP. To make this decision more reversible, we invested in building tooling such that the choice of database is abstracted from the application code.
Shell scripting is a powerful tool for automation, system administration, and software deployment. However, as your shell scripts grow in size and com......
When we introduced GitHub Copilot back in 2021, we had a clear goal: to make developers’ lives easier with an AI pair programmer that helps them write......
This week's Java roundup for February 17th, 2025 capabilities news highlighting: the release of Apache NetBeans 25; the February 2025 release of the Payar......
Market Impact Analysis
Market Growth Trend
2018 | 2019 | 2020 | 2021 | 2022 | 2023 | 2024 |
---|---|---|---|---|---|---|
7.5% | 9.0% | 9.4% | 10.5% | 11.0% | 11.4% | 11.5% |
Quarterly Growth Rate
Q1 2024 | Q2 2024 | Q3 2024 | Q4 2024 |
---|---|---|---|
10.8% | 11.1% | 11.3% | 11.5% |
Market Segments and Growth Drivers
Segment | Market Share | Growth Rate |
---|---|---|
Enterprise Software | 38% | 10.8% |
Cloud Services | 31% | 17.5% |
Developer Tools | 14% | 9.3% |
Security Software | 12% | 13.2% |
Other Software | 5% | 7.5% |
Technology Maturity Curve
Different technologies within the ecosystem are at varying stages of maturity:
Competitive Landscape Analysis
Company | Market Share |
---|---|
Microsoft | 22.6% |
Oracle | 14.8% |
SAP | 12.5% |
Salesforce | 9.7% |
Adobe | 8.3% |
Future Outlook and Predictions
The Github Copilot Agent landscape is evolving rapidly, driven by technological advancements, changing threat vectors, and shifting business requirements. Based on current trends and expert analyses, we can anticipate several significant developments across different time horizons:
Year-by-Year Technology Evolution
Based on current trajectory and expert analyses, we can project the following development timeline:
Technology Maturity Curve
Different technologies within the ecosystem are at varying stages of maturity, influencing adoption timelines and investment priorities:
Innovation Trigger
- Generative AI for specialized domains
- Blockchain for supply chain verification
Peak of Inflated Expectations
- Digital twins for business processes
- Quantum-resistant cryptography
Trough of Disillusionment
- Consumer AR/VR applications
- General-purpose blockchain
Slope of Enlightenment
- AI-driven analytics
- Edge computing
Plateau of Productivity
- Cloud infrastructure
- Mobile applications
Technology Evolution Timeline
- Technology adoption accelerating across industries
- digital transformation initiatives becoming mainstream
- Significant transformation of business processes through advanced technologies
- new digital business models emerging
- Fundamental shifts in how technology integrates with business and society
- emergence of new technology paradigms
Expert Perspectives
Leading experts in the software dev sector provide diverse perspectives on how the landscape will evolve over the coming years:
"Technology transformation will continue to accelerate, creating both challenges and opportunities."
— Industry Expert
"Organizations must balance innovation with practical implementation to achieve meaningful results."
— Technology Analyst
"The most successful adopters will focus on business outcomes rather than technology for its own sake."
— Research Director
Areas of Expert Consensus
- Acceleration of Innovation: The pace of technological evolution will continue to increase
- Practical Integration: Focus will shift from proof-of-concept to operational deployment
- Human-Technology Partnership: Most effective implementations will optimize human-machine collaboration
- Regulatory Influence: Regulatory frameworks will increasingly shape technology development
Short-Term Outlook (1-2 Years)
In the immediate future, organizations will focus on implementing and optimizing currently available technologies to address pressing software dev challenges:
- Technology adoption accelerating across industries
- digital transformation initiatives becoming mainstream
These developments will be characterized by incremental improvements to existing frameworks rather than revolutionary changes, with emphasis on practical deployment and measurable outcomes.
Mid-Term Outlook (3-5 Years)
As technologies mature and organizations adapt, more substantial transformations will emerge in how security is approached and implemented:
- Significant transformation of business processes through advanced technologies
- new digital business models emerging
This period will see significant changes in security architecture and operational models, with increasing automation and integration between previously siloed security functions. Organizations will shift from reactive to proactive security postures.
Long-Term Outlook (5+ Years)
Looking further ahead, more fundamental shifts will reshape how cybersecurity is conceptualized and implemented across digital ecosystems:
- Fundamental shifts in how technology integrates with business and society
- emergence of new technology paradigms
These long-term developments will likely require significant technical breakthroughs, new regulatory frameworks, and evolution in how organizations approach security as a fundamental business function rather than a technical discipline.
Key Risk Factors and Uncertainties
Several critical factors could significantly impact the trajectory of software dev evolution:
Organizations should monitor these factors closely and develop contingency strategies to mitigate potential negative impacts on technology implementation timelines.
Alternative Future Scenarios
The evolution of technology can follow different paths depending on various factors including regulatory developments, investment trends, technological breakthroughs, and market adoption. We analyze three potential scenarios:
Optimistic Scenario
Rapid adoption of advanced technologies with significant business impact
Key Drivers: Supportive regulatory environment, significant research breakthroughs, strong market incentives, and rapid user adoption.
Probability: 25-30%
Base Case Scenario
Measured implementation with incremental improvements
Key Drivers: Balanced regulatory approach, steady technological progress, and selective implementation based on clear ROI.
Probability: 50-60%
Conservative Scenario
Technical and organizational barriers limiting effective adoption
Key Drivers: Restrictive regulations, technical limitations, implementation challenges, and risk-averse organizational cultures.
Probability: 15-20%
Scenario Comparison Matrix
Factor | Optimistic | Base Case | Conservative |
---|---|---|---|
Implementation Timeline | Accelerated | Steady | Delayed |
Market Adoption | Widespread | Selective | Limited |
Technology Evolution | Rapid | Progressive | Incremental |
Regulatory Environment | Supportive | Balanced | Restrictive |
Business Impact | Transformative | Significant | Modest |
Transformational Impact
Technology becoming increasingly embedded in all aspects of business operations. This evolution will necessitate significant changes in organizational structures, talent development, and strategic planning processes.
The convergence of multiple technological trends—including artificial intelligence, quantum computing, and ubiquitous connectivity—will create both unprecedented security challenges and innovative defensive capabilities.
Implementation Challenges
Technical complexity and organizational readiness remain key challenges. Organizations will need to develop comprehensive change management strategies to successfully navigate these transitions.
Regulatory uncertainty, particularly around emerging technologies like AI in security applications, will require flexible security architectures that can adapt to evolving compliance requirements.
Key Innovations to Watch
Artificial intelligence, distributed systems, and automation technologies leading innovation. Organizations should monitor these developments closely to maintain competitive advantages and effective security postures.
Strategic investments in research partnerships, technology pilots, and talent development will position forward-thinking organizations to leverage these innovations early in their development cycle.
Technical Glossary
Key technical terms and definitions to help understand the technologies discussed in this article.
Understanding the following technical concepts is essential for grasping the full implications of the security threats and defensive measures discussed in this article. These definitions provide context for both technical and non-technical readers.