Cappy: Outperforming and boosting large multi-task language models with a small scorer - Related to multi-task, understanding, table, cappy:, boosting
Cappy: Outperforming and boosting large multi-task language models with a small scorer
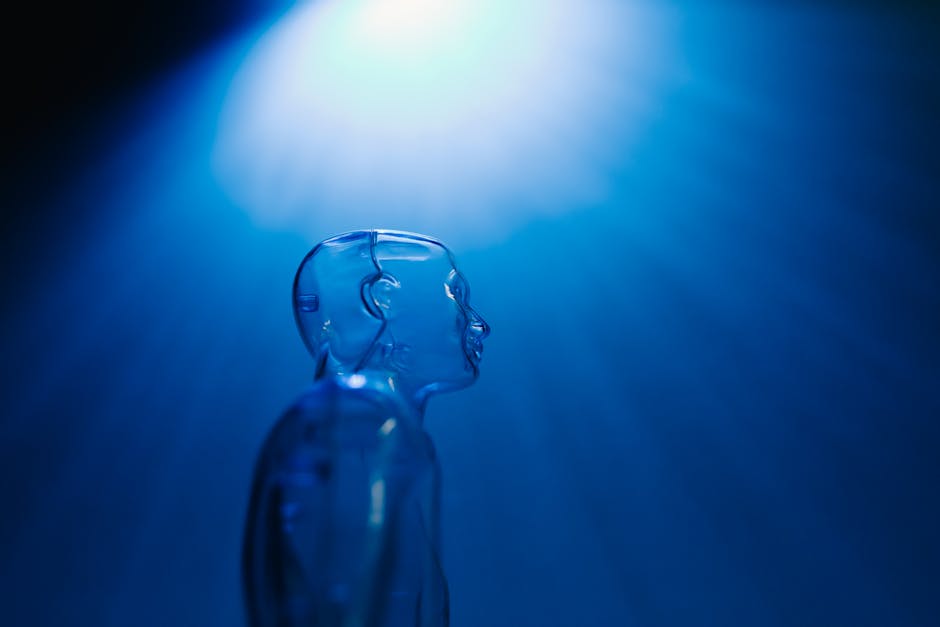
Due to the complexity of understanding and solving various tasks solely using instructions, the size of multi-task LLMs typically spans from several billion parameters to hundreds of billions (, FLAN-11B. T0-11B and OPT-IML-175B). As a result, operating such sizable models poses significant challenges because they demand considerable computational power and impose substantial requirements on the memory capacities of GPUs and TPUs. Making their training and inference expensive and inefficient. Extensive storage is required to maintain a unique LLM copy for each downstream task. Moreover, the most powerful multi-task LLMs (, FLAN-PaLM-540B) are closed-sourced, making them impossible to be adapted. However, in practical applications, harnessing a single multi-task LLM to manage all conceivable tasks in a zero-shot manner remains difficult, particularly when dealing with complex tasks. Personalized tasks and those that cannot be succinctly defined using instructions. On the other hand, the size of downstream training data is usually insufficient to train a model well without incorporating rich prior knowledge. Hence, it is long desired to adapt LLMs with downstream supervision while bypassing storage, memory, and access issues.
Certain parameter-efficient tuning strategies, including prompt tuning and adapters, substantially diminish storage requirements. But they still perform back-propagation through LLM parameters during the tuning process, thereby keeping their memory demands high. Additionally, some in-context learning techniques circumvent parameter tuning by integrating a limited number of supervised examples into the instruction. However, these techniques are constrained by the model's maximum input length, which permits only a few samples to guide task resolution.
In “Cappy: Outperforming and Boosting Large Multi-Task LMs with a Small Scorer”, presented at NeurIPS 2023. We propose a novel approach that enhances the performance and efficiency of multi-task LLMs. We introduce a lightweight pre-trained scorer, Cappy, based on continual pre-training on top of RoBERTa with merely 360 million parameters. Cappy takes in an instruction and a candidate response as input, and produces a score between 0 and. 1, indicating an estimated correctness of the response with respect to the instruction. Cappy functions either independently on classification tasks or serves as an auxiliary component for LLMs, boosting their performance. Moreover, Cappy efficiently enables downstream supervision without requiring any finetuning, which avoids the need for back-propagation through LLM parameters and reduces memory requirements. Finally, adaptation with Cappy doesn’t require access to LLM parameters as it is compatible with closed-source multi-task LLMs, such as those only accessible via WebAPIs.
Objects and their relationships are ubiquitous in the world around us, and relationships can be as critical to understanding an object as its own att...
AI-driven technologies are weaving themselves into the fabric of our daily routines, with the potential to enhance our access to knowledge and. Boost o...
It’s been a great year for the Dutch startup ecosystem.
Venture capitalists have, so far, invested $ into Netherlands-based early-stage companie...
Chain-of-table: Evolving tables in the reasoning chain for table understanding
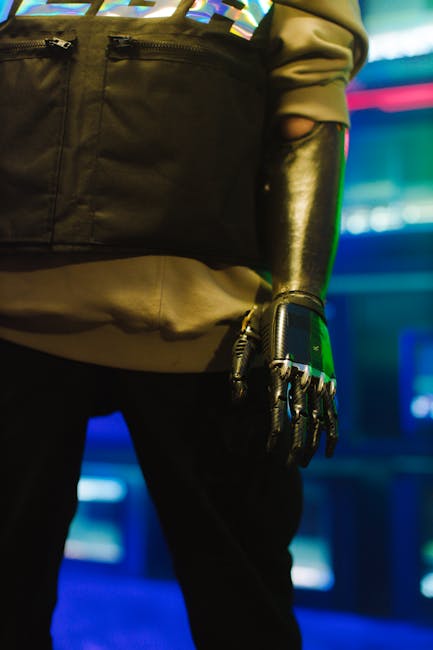
People use tables every day to organize and interpret complex information in a structured, easily accessible format. Due to the ubiquity of such tables, reasoning over tabular data has long been a central topic in natural language processing (NLP). Researchers in this field have aimed to leverage language models to help clients answer questions, verify statements, and analyze data based on tables. However, language models are trained over large amounts of plain text, so the inherently structured nature of tabular data can be difficult for language models to fully comprehend and utilize.
in recent times, large language models (LLMs) have achieved outstanding performance across diverse natural language understanding (NLU) tasks by generating reliable reasoning chains. As shown in works like Chain-of-Thought and Least-to-Most. However, the most suitable way for LLMs to reason over tabular data remains an open question.
In “Chain-of-Table: Evolving Tables in the Reasoning Chain for Table Understanding”, we propose a framework to tackle table understanding tasks, where we train LLMs to outline their reasoning step by step. Updating a given table iteratively to reflect each part of a thought process, akin to how people solve the table-based problems. This enables the LLM to transform the table into simpler and more manageable segments so that it can understand and. Analyze each part of the table in depth. This approach has yielded significant improvements and achieved new state-of-the-art results on the WikiTQ, TabFact, and FeTaQA benchmarks. The figure below presents the high-level overview of the proposed Chain-of-Table and other methods.
Google DeepMind spinoff Isomorphic Labs expects testing on its first AI-designed drugs to begin this year, as tech startups race to turn algorithmic m...
For the past few months. Meta has been sending recipes to a Dutch scaleup called VSParticle (VSP). These are not food recipes — they’re AI-generated i...
Due to the complexity of understanding and solving various tasks solely using instructions. The size of multi-task LLMs typically spans from several b...
Using AI to expand global access to reliable flood forecasts
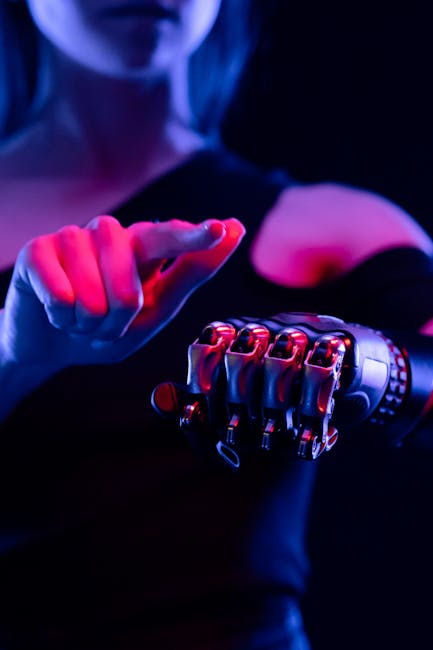
Floods are the most common natural disaster, and are responsible for roughly $50 billion in annual financial damages worldwide. The rate of flood-related disasters has more than doubled since the year 2000 partly due to climate change. Nearly billion people, making up 19% of the world’s population, are exposed to substantial risks from severe flood events. Upgrading early warning systems to make accurate and timely information accessible to these populations can save thousands of lives per year.
Driven by the potential impact of reliable flood forecasting on people’s lives globally. We started our flood forecasting effort in 2017. Through this multi-year journey, we advanced research over the years hand-in-hand with building a real-time operational flood forecasting system that provides alerts on Google Search, Maps. Android notifications and through the Flood Hub. However, in order to scale globally, especially in places where accurate local data is not available, more research advances were required.
In “Global prediction of extreme floods in ungauged watersheds”, . We demonstrate how machine learning (ML) technologies can significantly improve global-scale flood forecasting relative to the current state-of-the-art for countries where flood-related data is scarce. With these AI-based technologies we extended the reliability of currently-available global nowcasts, on average, from zero to five days, and. Improved forecasts across regions in Africa and Asia to be similar to what are currently available in Europe. The evaluation of the models was conducted in collaboration with the European Center for Medium Range Weather Forecasting (ECMWF).
These technologies also enable Flood Hub to provide real-time river forecasts up to seven days in advance. Covering river reaches across over 80 countries. This information can be used by people, communities, governments and international organizations to take anticipatory action to help protect vulnerable populations.
We leverage two key techniques to aid convergence of this ill-posed problem. The first is a very lightweight, dynamically trained convolutional neural...
People use tables every day to organize and interpret complex information in a structured. Easily accessible format. Due to the ubiquity of such table...
Machine learning models in the real world are often trained on limited data that may contain unintended statistical biases. For example, in the CELEBA...
Market Impact Analysis
Market Growth Trend
2018 | 2019 | 2020 | 2021 | 2022 | 2023 | 2024 |
---|---|---|---|---|---|---|
23.1% | 27.8% | 29.2% | 32.4% | 34.2% | 35.2% | 35.6% |
Quarterly Growth Rate
Q1 2024 | Q2 2024 | Q3 2024 | Q4 2024 |
---|---|---|---|
32.5% | 34.8% | 36.2% | 35.6% |
Market Segments and Growth Drivers
Segment | Market Share | Growth Rate |
---|---|---|
Machine Learning | 29% | 38.4% |
Computer Vision | 18% | 35.7% |
Natural Language Processing | 24% | 41.5% |
Robotics | 15% | 22.3% |
Other AI Technologies | 14% | 31.8% |
Technology Maturity Curve
Different technologies within the ecosystem are at varying stages of maturity:
Competitive Landscape Analysis
Company | Market Share |
---|---|
Google AI | 18.3% |
Microsoft AI | 15.7% |
IBM Watson | 11.2% |
Amazon AI | 9.8% |
OpenAI | 8.4% |
Future Outlook and Predictions
The Chain Table Cappy landscape is evolving rapidly, driven by technological advancements, changing threat vectors, and shifting business requirements. Based on current trends and expert analyses, we can anticipate several significant developments across different time horizons:
Year-by-Year Technology Evolution
Based on current trajectory and expert analyses, we can project the following development timeline:
Technology Maturity Curve
Different technologies within the ecosystem are at varying stages of maturity, influencing adoption timelines and investment priorities:
Innovation Trigger
- Generative AI for specialized domains
- Blockchain for supply chain verification
Peak of Inflated Expectations
- Digital twins for business processes
- Quantum-resistant cryptography
Trough of Disillusionment
- Consumer AR/VR applications
- General-purpose blockchain
Slope of Enlightenment
- AI-driven analytics
- Edge computing
Plateau of Productivity
- Cloud infrastructure
- Mobile applications
Technology Evolution Timeline
- Improved generative models
- specialized AI applications
- AI-human collaboration systems
- multimodal AI platforms
- General AI capabilities
- AI-driven scientific breakthroughs
Expert Perspectives
Leading experts in the ai tech sector provide diverse perspectives on how the landscape will evolve over the coming years:
"The next frontier is AI systems that can reason across modalities and domains with minimal human guidance."
— AI Researcher
"Organizations that develop effective AI governance frameworks will gain competitive advantage."
— Industry Analyst
"The AI talent gap remains a critical barrier to implementation for most enterprises."
— Chief AI Officer
Areas of Expert Consensus
- Acceleration of Innovation: The pace of technological evolution will continue to increase
- Practical Integration: Focus will shift from proof-of-concept to operational deployment
- Human-Technology Partnership: Most effective implementations will optimize human-machine collaboration
- Regulatory Influence: Regulatory frameworks will increasingly shape technology development
Short-Term Outlook (1-2 Years)
In the immediate future, organizations will focus on implementing and optimizing currently available technologies to address pressing ai tech challenges:
- Improved generative models
- specialized AI applications
- enhanced AI ethics frameworks
These developments will be characterized by incremental improvements to existing frameworks rather than revolutionary changes, with emphasis on practical deployment and measurable outcomes.
Mid-Term Outlook (3-5 Years)
As technologies mature and organizations adapt, more substantial transformations will emerge in how security is approached and implemented:
- AI-human collaboration systems
- multimodal AI platforms
- democratized AI development
This period will see significant changes in security architecture and operational models, with increasing automation and integration between previously siloed security functions. Organizations will shift from reactive to proactive security postures.
Long-Term Outlook (5+ Years)
Looking further ahead, more fundamental shifts will reshape how cybersecurity is conceptualized and implemented across digital ecosystems:
- General AI capabilities
- AI-driven scientific breakthroughs
- new computing paradigms
These long-term developments will likely require significant technical breakthroughs, new regulatory frameworks, and evolution in how organizations approach security as a fundamental business function rather than a technical discipline.
Key Risk Factors and Uncertainties
Several critical factors could significantly impact the trajectory of ai tech evolution:
Organizations should monitor these factors closely and develop contingency strategies to mitigate potential negative impacts on technology implementation timelines.
Alternative Future Scenarios
The evolution of technology can follow different paths depending on various factors including regulatory developments, investment trends, technological breakthroughs, and market adoption. We analyze three potential scenarios:
Optimistic Scenario
Responsible AI driving innovation while minimizing societal disruption
Key Drivers: Supportive regulatory environment, significant research breakthroughs, strong market incentives, and rapid user adoption.
Probability: 25-30%
Base Case Scenario
Incremental adoption with mixed societal impacts and ongoing ethical challenges
Key Drivers: Balanced regulatory approach, steady technological progress, and selective implementation based on clear ROI.
Probability: 50-60%
Conservative Scenario
Technical and ethical barriers creating significant implementation challenges
Key Drivers: Restrictive regulations, technical limitations, implementation challenges, and risk-averse organizational cultures.
Probability: 15-20%
Scenario Comparison Matrix
Factor | Optimistic | Base Case | Conservative |
---|---|---|---|
Implementation Timeline | Accelerated | Steady | Delayed |
Market Adoption | Widespread | Selective | Limited |
Technology Evolution | Rapid | Progressive | Incremental |
Regulatory Environment | Supportive | Balanced | Restrictive |
Business Impact | Transformative | Significant | Modest |
Transformational Impact
Redefinition of knowledge work, automation of creative processes. This evolution will necessitate significant changes in organizational structures, talent development, and strategic planning processes.
The convergence of multiple technological trends—including artificial intelligence, quantum computing, and ubiquitous connectivity—will create both unprecedented security challenges and innovative defensive capabilities.
Implementation Challenges
Ethical concerns, computing resource limitations, talent shortages. Organizations will need to develop comprehensive change management strategies to successfully navigate these transitions.
Regulatory uncertainty, particularly around emerging technologies like AI in security applications, will require flexible security architectures that can adapt to evolving compliance requirements.
Key Innovations to Watch
Multimodal learning, resource-efficient AI, transparent decision systems. Organizations should monitor these developments closely to maintain competitive advantages and effective security postures.
Strategic investments in research partnerships, technology pilots, and talent development will position forward-thinking organizations to leverage these innovations early in their development cycle.
Technical Glossary
Key technical terms and definitions to help understand the technologies discussed in this article.
Understanding the following technical concepts is essential for grasping the full implications of the security threats and defensive measures discussed in this article. These definitions provide context for both technical and non-technical readers.