Semantic understanding, not just vectors: How Intuit’s data architecture powers agentic AI with measurable ROI - Related to agentic, powers, enhanced, granite, just
IBM Granite 3.2 adds Enhanced Reasoning to its AI mix
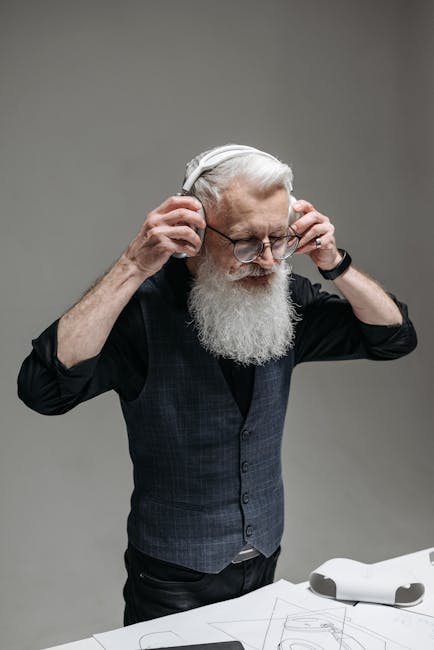
In its latest addition to its Granite family of large language models (LLMs), IBM has unveiled Granite This new release focuses on delivering small, efficient. Practical artificial intelligence (AI) solutions for businesses.
IBM has continued to improvement its Granite LLMs line at a rapid rate. Its last release, Granite , appeared at the end of 2024. That version was essentially an improvement. This new model, however, adds experimental chain-of-thought (CoT) reasoning capabilities to its bag of tricks.
Also: Most US workers don't use AI at work yet. This study points to a reason why.
CoT reasoning is an advanced AI technique that enables LLMs to break down complex problems into logical steps. This process is meant to imitate human-like reasoning processes. In theory, this approach significantly enhances an LLM's ability to handle tasks requiring multi-step reasoning, calculation, and. Decision-making.
In particular, IBM CoT uses a Thought Preference Optimization framework that enhances reasoning across a broad spectrum of instruction-following tasks. Unlike traditional reinforcement learning approaches focused mainly on logic-driven tasks, TPO allows for improved reasoning performance without sacrificing general task effectiveness. This approach helps mitigate common performance trade-offs seen in other models that specialize in reasoning.
So, what does this advance mean for you and me? IBM explained that if you think about giving an AI chatbot a prompt, a process called "prompt chaining", you get a specific answer. For example, with prompt chaining the question "What color is the sky?", you should get the answer "Blue."
"However, if asked to explain 'Why is the sky blue?' using CoT prompting, the AI would first define what 'blue' means (a primary color). Then deduce that the sky appears blue due to the absorption of other colors by the atmosphere. This response demonstrates the AI's ability to construct a logical argument," or the appearance that the LLM is reasoning its way to an answer.
Also: 15 ways AI has saved me time at work - and how I plan to use it now.
CoT is available in the Granite 8B and. 2B versions. Developers can toggle reasoning on or off programmatically. This option enables businesses to optimize computational resources based on task complexity. After all, sometimes you want to know what the sky is like without any scientific details. This approach, IBM indicates, enables the 8B model to rival the performance of much larger models, such as Claude Sonnet and. GPT-4o on complex mathematical reasoning tasks.
IBM has also introduced a new two-billion-parameter Vision Language Model (VLM), specifically designed for document-understanding tasks. This development is not, as you might first think, a graphics function. Instead, the VLM is meant to improve Granite's document-understanding abilities. IBM used its open-source Docling toolkit to process 85 million PDFs and generated 26 million synthetic question-answer pairs to enhance the VLM's ability to handle complex document-heavy workflows.
While other AI companies appear to swerve safety issues. IBM still considers safety a top-of-mind function. Granite Guardian , the latest in IBM's suite of AI safety models, offers enhanced risk detection in prompts and responses. This updated version maintains performance while reducing model size by 30%, introducing a new "verbalized confidence" feature for more nuanced risk assessment.
Also: OpenAI finally unveils Here's what it can do.
Businesses may also be interested in Granite's advanced forecasting capabilities. The new TinyTimeMixers (TTM) models with sub-10M parameters can run long-term forecasting up to two years into the future. These models are useful for trend analysis in finance, economics, and supply chain management. These models might not help you assemble your fantasy baseball team roster yet, but. Give them time.
As before, IBM is the most open-source friendly AI firm. All Granite models are available under the Apache license on Hugging Face. Some models are available on platforms, including IBM , Ollama, Replicate, and LM Studio. This open approach aligns with IBM's strategy to make AI more accessible and cost-effective for enterprises.
As Sriram Raghavan, IBM AI research VP, emphasized: "The next era of AI is about efficiency, integration, and. Real-world impact -- where enterprises can achieve powerful outcomes without excessive spend on compute."
Amblyopia, often referred to as ‘lazy eye’, is a prevalent yet frequently overlooked vision disorder that affects 1-5% of the global population. Its p...
I've been experimenting with using ChatGPT to help turbocharge my programming output for over two years. When ChatGPT helped me fi...
Microsoft has expanded its Copilot AI to Mac consumers. On Thursday, the official Copilot app landed in the Mac App Store in the US, Canada, and th...
Why Walmart Built Wallaby
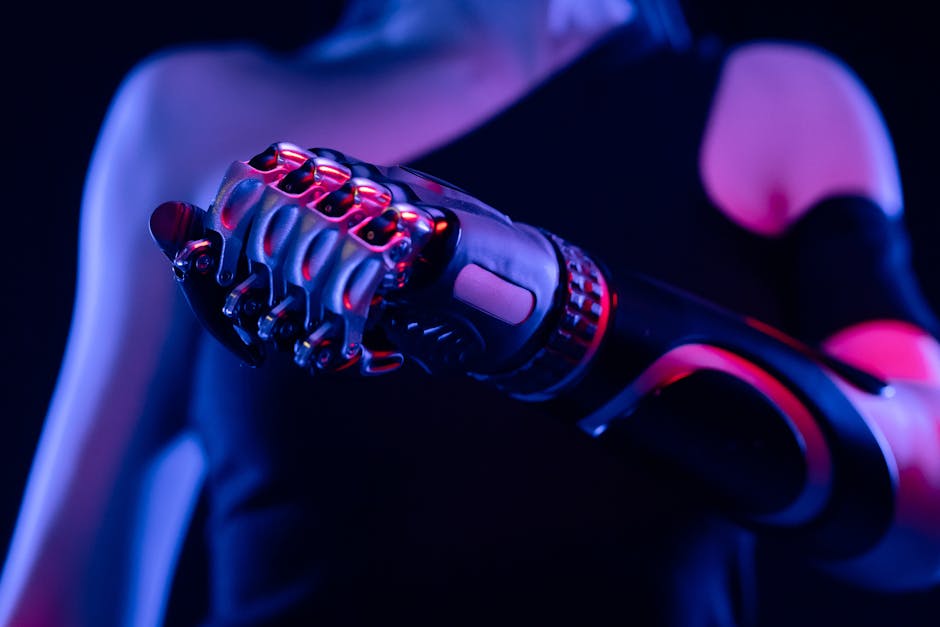
In October last year, Walmart revealed its plans around AI, AR, and immersive commerce experiences. This led to the introduction of Wallaby, a collection of retail-focused LLMs designed to enhance customer interactions.
Built on decades of Walmart data, Wallaby allows the firm to integrate it with other LLMs. Generating highly contextual responses tailored to its ecosystem. At the centre of this evolution is Sriprabha Gopalan, director of engineering at Walmart Global Tech, whose team is driving innovations in generative AI, conversational AI, and retail-specific LLMs.
“The best thing about Wallaby LLMs is that we’ve trained them in a way that they can speak in a very natural tone that complies with Walmart’s code of conduct,” Gopalan, who has over eight years of experience at the firm. Told AIM.
What makes Wallaby particularly powerful is its ability to integrate with other LLMs, allowing for enhanced performance across multiple retail applications. By combining proprietary AI models with external innovations, Walmart ensures its AI systems stay ahead of the curve.
Beyond LLMs, Walmart is also pioneering conversational AI, which enables natural. Free-flowing interactions between end-customers and digital assistants. The organization has successfully implemented a GenAI-based shopping assistant that acts as a real-time advisor, helping end-customers discover and select products tailored to their needs.
“This AI-powered assistant enables clients to engage in a natural conversation and. Decide on the best products for their unique needs,” Gopalan stated. The assistant is designed to mimic an in-store experience, making online shopping more interactive and. Personalised.
Customer support has also seen significant advancements with generative AI. Walmart integrated AI into its customer support assistant, which now understands customer intent and takes direct actions, such as managing orders and processing returns.
“The effectiveness of our customer support workflows has doubled since integrating GenAI,” noted Gopalan, highlighting a significant reduction in issue resolution time.
AI at Walmart isn’t just enhancing customer experience. It’s also transforming how developers and engineers work. The corporation has built several AI-powered tools to boost productivity and efficiency, and streamline engineering workflows.
One such tool is DX AI Assistant, an internal marketplace of generative AI chatbots that helps developers discover and. Utilise AI solutions for various engineering tasks. Another tool, My Assistant, launched 18 months ago, assists with drafting, summarising documentation, and retrieving concise technical information, saving developers significant time on routine tasks.
“These tools allow our developers to focus on high-impact areas, such as ideation, creativity. And strategy, rather than repetitive tasks,” explained Gopalan.
The rise of AI-powered coding assistants has sparked debates in the engineering community. Some argue that these tools make junior developers overly dependent on AI, while others believe they enhance productivity. Addressing this concern, Gopalan remains optimistic.
“We see AI as a powerful enabler rather than a replacement for human expertise. These tools help engineers focus on complex problem-solving instead of repetitive coding tasks. The real innovation comes from combining AI’s efficiency with human ingenuity,” she expressed.
While Walmart operates as a unified global entity. Its Indian tech teams have played a crucial role in driving AI innovations. In addition to their contributions to Wallaby LLMs and conversational AI, they have worked on Converse, an in-house conversational AI platform designed for internal and customer-facing applications.
Moreover, Walmart has expanded its research partnerships. Most notably through the Walmart Centre for Excellence in collaboration with IIT Madras.
“We don’t distinguish between locations; our focus is on collaboration and delivering value to clients,” Gopalan presented. This philosophy has enabled Walmart’s global tech teams, including those in India, to contribute to groundbreaking advancements, such as Wallaby LLMs and AI-driven shopping assistants.
Looking ahead, Gopalan expressed that she is exploring AI agents that could further automate shopping. Customer support, and even supply chain operations. With AI advancements enabling greater autonomy and intelligence, there is potential for fully AI-driven shopping experiences where clients can place orders via voice commands with minimal manual input.
Additionally. Walmart is investing in geospatial technology to optimise delivery networks. By using AI-driven demand forecasting, slot availability, and store capacity data, Walmart has enhanced its last-mile delivery efficiency, ensuring faster and more reliable deliveries.
“We believe that AI, combined with human expertise. Is the key to driving the next wave of retail innovation,” Gopalan mentioned.
Apple held its annual iPhone event back in September 2024 and debuted the iPhone 16 series. Much of the presenta...
Chinese AI startup DeepSeek has reported a theoretical daily profit margin of 545% for its inference services. Despite limitations in monetisation and...
Microsoft has expanded its Copilot AI to Mac clients. On Thursday, the official Copilot app landed in the Mac App Store in the US, Canada, and th...
Semantic understanding, not just vectors: How Intuit’s data architecture powers agentic AI with measurable ROI
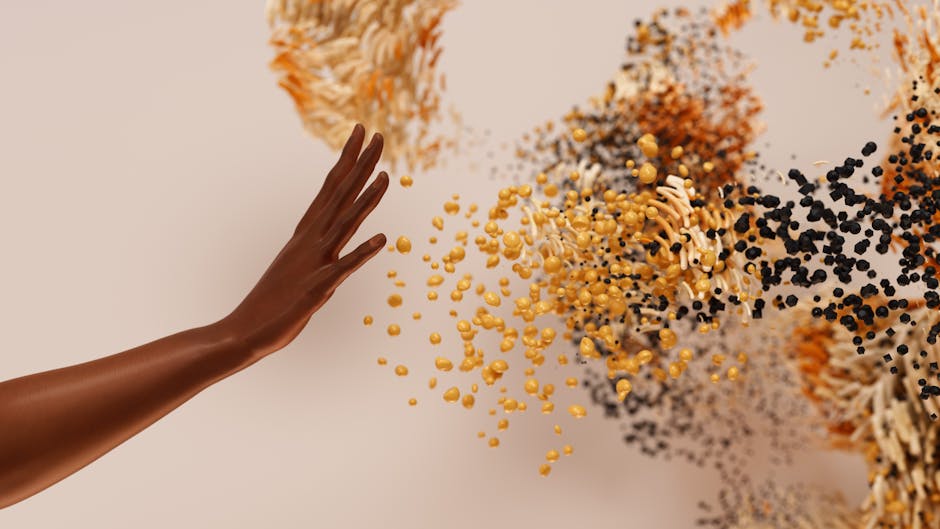
Intuit — the financial software giant behind products like TurboTax and QuickBooks — is making significant strides using generative AI to enhance its offerings for small business consumers.
In a tech landscape flooded with AI promises. Intuit has built an agent-based AI architecture that’s delivering tangible business outcomes for small businesses. The business has deployed what it calls “done for you” experiences that autonomously handle entire workflows and deliver quantifiable business impact.
Intuit has been building out its own AI layer. Which it calls a generative AI operating system (GenOS). The firm detailed some of the ways it is using gen AI to improve personalization at VB Transform 2024. In Sept. 2024, Intuit added agentic AI workflows, an effort that has improved operations for both the firm and its clients.
. QuickBooks Online clients are getting paid an average of five days faster, with overdue invoices 10% more likely to be paid in full. For small businesses where cash flow is king, these aren’t just incremental improvements — they’re potentially business-saving innovations.
The technical trinity: How Intuit’s data architecture enables true agentic AI.
What separates Intuit’s approach from competitors is its sophisticated data architecture designed specifically to enable agent-based AI experiences.
Additionally, the business has built what CDO Ashok Srivastava calls “a trinity” of data systems:
Data lake: The foundational repository for all data. Customer data cloud (CDC): A specialized serving layer for AI experiences. “Event bus“: A streaming data system enabling real-time operations.
“CDC provides a serving layer for AI experiences. Then the data lake is kind of the repository for all such data,” Srivastava told VentureBeat. “The agent is going to be interacting with data, and it has a set of data that it could look at in order to pull information.”.
Going beyond vector embeddings to power agentic AI.
Additionally, the Intuit architecture diverges from the typical vector database approach many enterprises are hastily implementing. While vector databases and embeddings are essential for powering AI models, Intuit recognizes that true semantic understanding requires a more holistic approach.
“Where the key issue continues to be is essentially in ensuring that we have a good, logical and. Semantic understanding of the data,” noted Srivastava.
To achieve this semantic understanding, Intuit is building out a semantic data layer on top of its core data infrastructure. The semantic data layer helps provide context and meaning around the data, beyond just the raw data itself or its vector representations. It allows Intuit’s AI agents to superior comprehend the relationships and connections between different data data and elements.
By building this semantic data layer. Intuit is able to augment the capabilities of its vector-based systems with a deeper, more contextual understanding of data. This allows AI agents to make more informed and meaningful decisions for consumers.
Beyond basic automation: How agentic AI completes entire business processes autonomously.
Unlike enterprises implementing AI for basic workflow automation or customer service chatbots. Intuit has focused on creating fully agentic “done for you” experiences. These are applications that handle complex, multi-step tasks while requiring only final human approval.
For QuickBooks people, the agentic system analyzes client payment history and invoice status to automatically draft personalized reminder messages. Allowing business owners to simply review and approve before sending. The system’s ability to personalize based on relationship context and payment patterns has directly contributed to measurably faster payments.
Intuit is applying identical agentic principles internally, developing autonomous procurement systems and HR assistants.
“We have the ability to have an internal agentic procurement process that employees can use to purchase supplies and book travel,” Srivastava explained. Demonstrating how the enterprise is eating its own AI dog food.
What potentially gives Intuit a competitive advantage over other enterprise AI implementations is how the system was designed with foresight about the emergence of advanced reasoning models like DeepSeek.
“We built gen runtime in anticipation of reasoning models coming up,” Ashok revealed. “We’re not behind the eight ball … we’re ahead of it. We built the capabilities assuming that reasoning would exist.”.
Additionally, this forward-thinking design means Intuit can rapidly incorporate new reasoning capabilities into their agentic experiences as they emerge. Without requiring architectural overhauls. , Intuit’s engineering teams are already using these capabilities to enable agents to reason across a large number of tools and data in ways that weren’t previously possible.
Shifting from AI hype to business impact.
Perhaps most significantly, Intuit’s approach exhibits a clear focus on business outcomes rather than technological showmanship.
“There’s a lot of work and a lot of fanfare going on these days on AI itself, that it’s going to revolutionize the world. And all of that, which I think is good,” stated Srivastava. “But I think what’s a lot improved is to show that it’s actually helping real people do improved.”.
The enterprise believes deeper reasoning capabilities will enable even more comprehensive “done for you” experiences that cover more customer needs with greater depth. Each experience combines multiple atomic experiences or discrete operations that together create a complete workflow solution.
What this means for enterprises adopting AI.
For enterprises looking to implement AI effectively. Intuit’s approach offers several valuable lessons for enterprises:
Focus on outcomes over technology : Rather than showcasing AI for its own sake target specific business pain points with measurable improvement goals.
: Rather than showcasing AI for its own sake target specific business pain points with measurable improvement goals. Build with future models in mind : Design architecture that can incorporate emerging reasoning capabilities without requiring a complete rebuild.
: Design architecture that can incorporate emerging reasoning capabilities without requiring a complete rebuild. Address data challenges first : Before rushing to implement agents, ensure your data foundation can support semantic understanding and cross-system reasoning.
: Before rushing to implement agents. Ensure your data foundation can support semantic understanding and cross-system reasoning. Create complete experiences: Look beyond simple automation to create end-to-end “done for you” workflows that deliver complete solutions.
As agentic AI continues to mature, enterprises that follow Intuit’s example by focusing on complete solutions rather than isolated AI capabilities may find themselves achieving similar concrete business results rather than simply generating tech buzz.
'ZDNET Recommends': What exactly does it mean?
ZDNET's recommendations are based on many hours of testing, research. And comparison shopping. We gath...
Additionally, the rapid release of advanced AI models in the past few days has been impossible to ignore. With the launch of Grok-3 and Claude Sonnet, two leadi...
Qui aurait cru que les grands-mères chinoises trouveraient plus de réconfort auprès de bébés générés par l’IA que de leurs petits-enfants ? Ces bébés ...
Market Impact Analysis
Market Growth Trend
2018 | 2019 | 2020 | 2021 | 2022 | 2023 | 2024 |
---|---|---|---|---|---|---|
23.1% | 27.8% | 29.2% | 32.4% | 34.2% | 35.2% | 35.6% |
Quarterly Growth Rate
Q1 2024 | Q2 2024 | Q3 2024 | Q4 2024 |
---|---|---|---|
32.5% | 34.8% | 36.2% | 35.6% |
Market Segments and Growth Drivers
Segment | Market Share | Growth Rate |
---|---|---|
Machine Learning | 29% | 38.4% |
Computer Vision | 18% | 35.7% |
Natural Language Processing | 24% | 41.5% |
Robotics | 15% | 22.3% |
Other AI Technologies | 14% | 31.8% |
Technology Maturity Curve
Different technologies within the ecosystem are at varying stages of maturity:
Competitive Landscape Analysis
Company | Market Share |
---|---|
Google AI | 18.3% |
Microsoft AI | 15.7% |
IBM Watson | 11.2% |
Amazon AI | 9.8% |
OpenAI | 8.4% |
Future Outlook and Predictions
The Granite Adds Enhanced landscape is evolving rapidly, driven by technological advancements, changing threat vectors, and shifting business requirements. Based on current trends and expert analyses, we can anticipate several significant developments across different time horizons:
Year-by-Year Technology Evolution
Based on current trajectory and expert analyses, we can project the following development timeline:
Technology Maturity Curve
Different technologies within the ecosystem are at varying stages of maturity, influencing adoption timelines and investment priorities:
Innovation Trigger
- Generative AI for specialized domains
- Blockchain for supply chain verification
Peak of Inflated Expectations
- Digital twins for business processes
- Quantum-resistant cryptography
Trough of Disillusionment
- Consumer AR/VR applications
- General-purpose blockchain
Slope of Enlightenment
- AI-driven analytics
- Edge computing
Plateau of Productivity
- Cloud infrastructure
- Mobile applications
Technology Evolution Timeline
- Improved generative models
- specialized AI applications
- AI-human collaboration systems
- multimodal AI platforms
- General AI capabilities
- AI-driven scientific breakthroughs
Expert Perspectives
Leading experts in the ai tech sector provide diverse perspectives on how the landscape will evolve over the coming years:
"The next frontier is AI systems that can reason across modalities and domains with minimal human guidance."
— AI Researcher
"Organizations that develop effective AI governance frameworks will gain competitive advantage."
— Industry Analyst
"The AI talent gap remains a critical barrier to implementation for most enterprises."
— Chief AI Officer
Areas of Expert Consensus
- Acceleration of Innovation: The pace of technological evolution will continue to increase
- Practical Integration: Focus will shift from proof-of-concept to operational deployment
- Human-Technology Partnership: Most effective implementations will optimize human-machine collaboration
- Regulatory Influence: Regulatory frameworks will increasingly shape technology development
Short-Term Outlook (1-2 Years)
In the immediate future, organizations will focus on implementing and optimizing currently available technologies to address pressing ai tech challenges:
- Improved generative models
- specialized AI applications
- enhanced AI ethics frameworks
These developments will be characterized by incremental improvements to existing frameworks rather than revolutionary changes, with emphasis on practical deployment and measurable outcomes.
Mid-Term Outlook (3-5 Years)
As technologies mature and organizations adapt, more substantial transformations will emerge in how security is approached and implemented:
- AI-human collaboration systems
- multimodal AI platforms
- democratized AI development
This period will see significant changes in security architecture and operational models, with increasing automation and integration between previously siloed security functions. Organizations will shift from reactive to proactive security postures.
Long-Term Outlook (5+ Years)
Looking further ahead, more fundamental shifts will reshape how cybersecurity is conceptualized and implemented across digital ecosystems:
- General AI capabilities
- AI-driven scientific breakthroughs
- new computing paradigms
These long-term developments will likely require significant technical breakthroughs, new regulatory frameworks, and evolution in how organizations approach security as a fundamental business function rather than a technical discipline.
Key Risk Factors and Uncertainties
Several critical factors could significantly impact the trajectory of ai tech evolution:
Organizations should monitor these factors closely and develop contingency strategies to mitigate potential negative impacts on technology implementation timelines.
Alternative Future Scenarios
The evolution of technology can follow different paths depending on various factors including regulatory developments, investment trends, technological breakthroughs, and market adoption. We analyze three potential scenarios:
Optimistic Scenario
Responsible AI driving innovation while minimizing societal disruption
Key Drivers: Supportive regulatory environment, significant research breakthroughs, strong market incentives, and rapid user adoption.
Probability: 25-30%
Base Case Scenario
Incremental adoption with mixed societal impacts and ongoing ethical challenges
Key Drivers: Balanced regulatory approach, steady technological progress, and selective implementation based on clear ROI.
Probability: 50-60%
Conservative Scenario
Technical and ethical barriers creating significant implementation challenges
Key Drivers: Restrictive regulations, technical limitations, implementation challenges, and risk-averse organizational cultures.
Probability: 15-20%
Scenario Comparison Matrix
Factor | Optimistic | Base Case | Conservative |
---|---|---|---|
Implementation Timeline | Accelerated | Steady | Delayed |
Market Adoption | Widespread | Selective | Limited |
Technology Evolution | Rapid | Progressive | Incremental |
Regulatory Environment | Supportive | Balanced | Restrictive |
Business Impact | Transformative | Significant | Modest |
Transformational Impact
Redefinition of knowledge work, automation of creative processes. This evolution will necessitate significant changes in organizational structures, talent development, and strategic planning processes.
The convergence of multiple technological trends—including artificial intelligence, quantum computing, and ubiquitous connectivity—will create both unprecedented security challenges and innovative defensive capabilities.
Implementation Challenges
Ethical concerns, computing resource limitations, talent shortages. Organizations will need to develop comprehensive change management strategies to successfully navigate these transitions.
Regulatory uncertainty, particularly around emerging technologies like AI in security applications, will require flexible security architectures that can adapt to evolving compliance requirements.
Key Innovations to Watch
Multimodal learning, resource-efficient AI, transparent decision systems. Organizations should monitor these developments closely to maintain competitive advantages and effective security postures.
Strategic investments in research partnerships, technology pilots, and talent development will position forward-thinking organizations to leverage these innovations early in their development cycle.
Technical Glossary
Key technical terms and definitions to help understand the technologies discussed in this article.
Understanding the following technical concepts is essential for grasping the full implications of the security threats and defensive measures discussed in this article. These definitions provide context for both technical and non-technical readers.