Soket AI Labs’ Introduces Project EKA to Develop Sovereign AI Models for India - Related to introduces, indians, 545%, reports, built
DeepSeek Reports 545% Daily Profit Despite Free AI Services
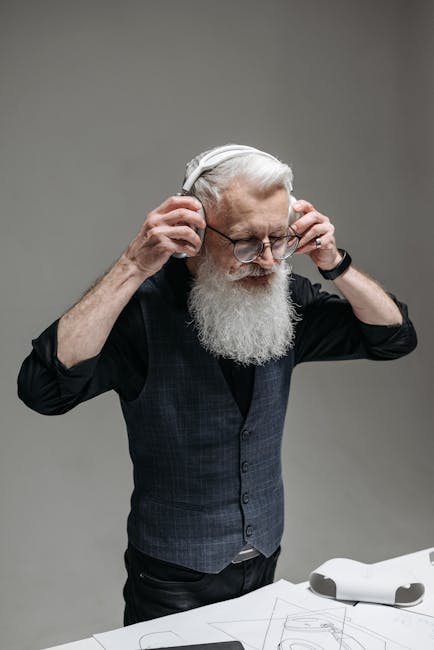
Chinese AI startup DeepSeek has reported a theoretical daily profit margin of 545% for its inference services, despite limitations in monetisation and discounted pricing structures. The enterprise shared these details in a recent GitHub post, outlining the operational costs and revenue potential of its DeepSeek-V3 and R1 models.
Based on DeepSeek-R1’s pricing model—charging $ per million input tokens for cache hits, $ per million for cache misses, and $ per million output tokens—the theoretical revenue generated daily is $562,027.
However, the firm acknowledged that actual earnings were significantly lower due to lower pricing for DeepSeek-V3. Free access to web and app services, and automatic nighttime discounts. “Our pricing strategy prioritises accessibility and long-term adoption over immediate revenue maximisation,” DeepSeek stated.
, DeepSeeks inference services run on NVIDIA H800 GPUs, with matrix multiplications and dispatch transmissions using the FP8 format. While core MLA computations and combine transmissions operate in BF16. The organization scales its GPU usage based on demand, deploying all nodes during peak hours and reducing them at night to allocate resources for research and training.
The GitHub post revealed that over a 24-hour period from February 27, 2025, to 12:00 PM on February 28. 2025, 12:00 PM, DeepSeek recorded peak node occupancy at 278, with an average of nodes in operation. With each node containing eight H800 GPUs and an estimated leasing cost of $2 per GPU per hour. The total daily expenditure reached $87,072.
The above revelation could affect the US stock market. The launch of DeepSeek’s latest model, R1, which the business proposes was trained on a $6 million budget, triggered a sharp market reaction. NVIDIA’s stock tumbled 17%, wiping out nearly $600 billion in value, driven by concerns over the model’s efficiency.
However, NVIDIA chief Jensen Huang, during the recent earnings call. noted the organization’s inference demand is accelerating, fuelled by test-time scaling and new reasoning models. “Models like OpenAI’s, Grok 3, and DeepSeek R1 are reasoning models that apply inference-time scaling. Reasoning models can consume 100 times more compute,” he noted.
“DeepSeek-R1 has ignited global enthusiasm. It’s an excellent innovation. But even more importantly, it has open-sourced a world-class reasoning AI model,” Huang expressed.
, DeepSeek plans to release its next reasoning model, the DeepSeek R2. ‘as early as possible.’ The organization initially planned to release it in early May but is now considering an earlier timeline. The model is expressed to produce ‘improved coding’ and reason in languages beyond English.
At TDS, we see value in every article we publish and recognize that authors share their work with us for a wide range of reasons — some wish to spread...
In a YouTube video titled Deep Dive into LLMs like ChatGPT, former Senior Director of AI at Tesla, Andrej Karpathy discusses the psychol...
Cloud-based data storage firm Snowflake on Thursday introduced its plans to open the Silicon Valley AI Hub. A dedicated space for developers, startu...
How Krutrim Built Chitrarth for a Billion Indians
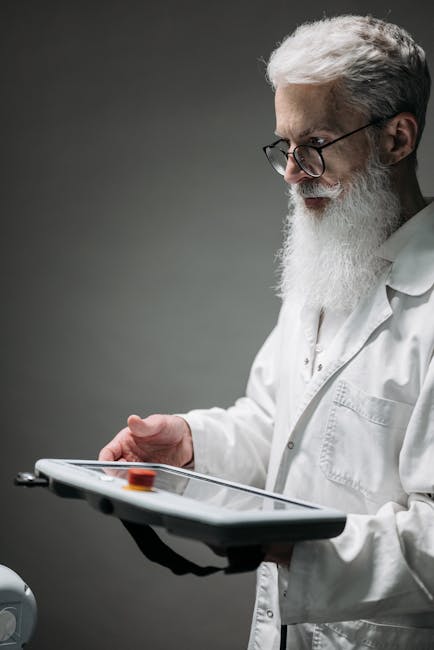
India has been aiming to develop its frontier AI model to serve the country’s vast population in their native language. However, this approach has many problems, including the lack of digitised data in Indian languages and also the unavailability of the images on which the models need to be trained.
To further the effort of building AI for Bharat, Ola’s Krutrim AI Lab has introduced Chitrarth. A multimodal Vision-Language Model (VLM). By combining multilingual text in ten predominant Indian languages with visual data, Chitrarth aims to democratise AI accessibility for over a billion Indians.
Most AI-powered VLMs struggle with linguistic inclusivity. As they are predominantly built on English datasets. This is also why BharatGen, the multimodal AI initiative supported by the Department of Science and Technology (DST), lately launched its e-vikrAI VLM for the Indian e-commerce ecosystem.
Similarly, Chitrarth is designed to close this language gap by supporting Hindi, Bengali, Telugu, Tamil, Marathi. Gujarati, Kannada, Malayalam, Odia, and Assamese. The model was built using Krutrim’s multilingual LLM as its backbone, ensuring it understands and generates content in these languages with high accuracy.
. Chitrarth is built on Krutrim-7B and incorporates SIGLIP (siglip-so400m-patch14-384) as its vision encoder. Its architecture follows a two-stage training process: Adapter Pre-Training (PT) and. Instruction Tuning (IT).
Pre-training is conducted using a dataset chosen for superior performance in initial experiments. The dataset is translated into multiple Indic languages using an open-source model, ensuring a balanced split between English and Indic languages.
Additionally, this approach maintains linguistic diversity, computational efficiency. And fairness in performance across languages. Fine-tuning is performed on an instruction dataset, enhancing the model’s ability to handle multimodal reasoning tasks.
The dataset includes a vision-language component containing academic tasks, in-house multilingual translations. And culturally significant images. The training data includes images representing prominent personalities, monuments, artwork, and cuisine, ensuring the model understands India’s diverse cultural heritage.
Chitrarth excels in tasks such as image captioning, visual question answering (VQA). And text-based image retrieval. The model is trained on multilingual image-text pairs, allowing it to interpret and describe images in multiple Indian languages.
This makes Chitrarth a game-changer for applications in education, accessibility, and digital content creation, enabling clients to interact with AI in their native language without relying on English as an intermediary.
Like BharatGen, Chitrarth’s capabilities enable it to support various real-world applications, including e-commerce, UI/UX analysis, monitoring systems, and creative writing.
For example. Automating product descriptions and attribute extraction for online retailers like Myntra, AJIO, and Nykaa is what the team is targeting as presented in the blog.
To evaluate Chitrarth’s performance across Indian languages, Krutrim developed BharatBench, a comprehensive benchmark suite designed for low-resource languages. BharatBench assesses VLMs on tasks such as VQA and image-text alignment, setting a new standard for multimodal AI in India.
Besides, Chitrarth has been evaluated against VLMs on academic multimodal tasks, consistently outperforming models like IDEFICS 2 (7B) and PALO 7B while maintaining competitive performance on TextVQA and VizWiz benchmarks.
Despite its advancements, Chitrarth faces challenges such as biases in automated translations and. The availability of high-quality training data for Indic languages.
Earlier this month, Ola chief Bhavish Aggarwal presented Krutrim AI Lab and the launch of several open source AI models tailored to India’s unique linguistic and cultural landscape. In addition to Chitrarth, these include the launch of Dhwani, Vyakhyarth, and Krutrim Translate.
In partnership with NVIDIA, the lab will also deploy India’s first GB200 supercomputer by March, and plans to scale it into the nation’s largest supercomputer by the end of the year.
This infrastructure will support the training and. Deployment of AI models, addressing challenges related to data scarcity and cultural context. The lab has committed to investing ₹2,000 crore into Krutrim, with a pledge to increase this to ₹10,000 crore by next year.
In an interview to Outlook Business. An Ola executive mentioned they plan to release Krutrim’s third model on August 15. It is likely to be a Mixture of Experts model consisting of 700 billion parameters. The team also has ambitious plans to develop its own AI chip, Bodhi, by 2028.
La lutte contre l’utilisation abusive de l’IA se renforce. Alors que Microsoft identifie plusieurs développeurs impliqués dans un réseau criminel. Mic...
'ZDNET Recommends': What exactly does it mean?
ZDNET's recommendations are based on many hours of testing, research, and comparison shopping. We gath...
Cloud-based data storage corporation Snowflake on Thursday introduced its plans to open the Silicon Valley AI Hub, a dedicated space for developers, startu...
Soket AI Labs’ Introduces Project EKA to Develop Sovereign AI Models for India
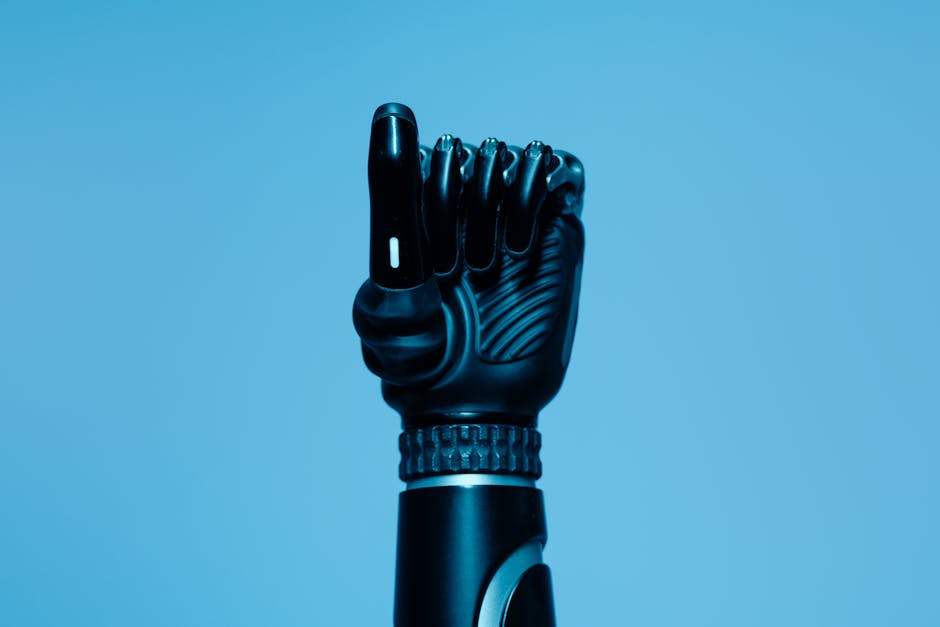
Project EKA, spearheaded by AI startup Soket Labs has emerged as India’s ambitious initiative to develop state-of-the-art foundation models that rival global AI systems while being optimised for India’s unique linguistic and socio-economic landscape.
Project EKA seeks to unite AI researchers, engineers, and institutions across the country to develop multilingual. High-efficiency AI models that cater to India’s needs while competing at a global scale.
, the initiative is focused on building an open, ethical, and high-impact AI ecosystem. Experts from premier institutions such as IITs, IISc, and other global research centers are collaborating to create a self-reliant AI infrastructure that spans multiple domains, from education and finance to national security and. Agriculture.
Beyond technological advancement, Project EKA aims to democratise AI access. AI-powered education tools could ensure that children across rural and urban areas learn in their native languages. In healthcare, AI-driven diagnostics could improve accessibility and efficiency. Meanwhile, in national security, real-time multilingual intelligence could enhance defense capabilities.
The project is rapidly gaining traction, with an expanding list of contributors from academia, industry. And AI research communities. While still in its early stages, EKA represents a growing movement toward India’s AI sovereignty, signaling a shift from AI dependency to AI leadership.
“I think we need at least $10 million to start working on frontier tech, and. This money should be purely dedicated to R&D for building these models—no distractions like building applications or even thinking about GTM. This is where investors and founders need to align with patient capital,” said Abhishek Upperwal, founder and. CEO of Soket AI Labs in an earlier interaction with AIM.
India’s push to develop its own AI foundation model gained momentum following DeepSeek’s launch. Upperwal had earlier noted that Pragna-1B, Soket’s billion-parameter AI model, is a step toward building frontier models.
Trained on a $100K budget, the plan is to bootstrap larger models using smaller ones and. Open-source alternatives while keeping compute costs low. He emphasised that high-quality data and training optimisations make this feasible, citing DeepSeek as an example.
However, with only $2-3 million in funding, progress on such models would be slow or deprioritised in favor of revenue-generating products.
Chinese tech giant Tencent has released its new AI model. Hunyuan Turbo S, which it says can answer queries faster than the DeepSeek-R1 model. The mod...
Amazon has been focused on bringing generative AI to Alexa for the past few years, hitting multiple delays in launching new featu...
Amblyopia. Often referred to as ‘lazy eye’, is a prevalent yet frequently overlooked vision disorder that affects 1-5% of the global population. Its p...
Market Impact Analysis
Market Growth Trend
2018 | 2019 | 2020 | 2021 | 2022 | 2023 | 2024 |
---|---|---|---|---|---|---|
23.1% | 27.8% | 29.2% | 32.4% | 34.2% | 35.2% | 35.6% |
Quarterly Growth Rate
Q1 2024 | Q2 2024 | Q3 2024 | Q4 2024 |
---|---|---|---|
32.5% | 34.8% | 36.2% | 35.6% |
Market Segments and Growth Drivers
Segment | Market Share | Growth Rate |
---|---|---|
Machine Learning | 29% | 38.4% |
Computer Vision | 18% | 35.7% |
Natural Language Processing | 24% | 41.5% |
Robotics | 15% | 22.3% |
Other AI Technologies | 14% | 31.8% |
Technology Maturity Curve
Different technologies within the ecosystem are at varying stages of maturity:
Competitive Landscape Analysis
Company | Market Share |
---|---|
Google AI | 18.3% |
Microsoft AI | 15.7% |
IBM Watson | 11.2% |
Amazon AI | 9.8% |
OpenAI | 8.4% |
Future Outlook and Predictions
The Deepseek Reports Daily landscape is evolving rapidly, driven by technological advancements, changing threat vectors, and shifting business requirements. Based on current trends and expert analyses, we can anticipate several significant developments across different time horizons:
Year-by-Year Technology Evolution
Based on current trajectory and expert analyses, we can project the following development timeline:
Technology Maturity Curve
Different technologies within the ecosystem are at varying stages of maturity, influencing adoption timelines and investment priorities:
Innovation Trigger
- Generative AI for specialized domains
- Blockchain for supply chain verification
Peak of Inflated Expectations
- Digital twins for business processes
- Quantum-resistant cryptography
Trough of Disillusionment
- Consumer AR/VR applications
- General-purpose blockchain
Slope of Enlightenment
- AI-driven analytics
- Edge computing
Plateau of Productivity
- Cloud infrastructure
- Mobile applications
Technology Evolution Timeline
- Improved generative models
- specialized AI applications
- AI-human collaboration systems
- multimodal AI platforms
- General AI capabilities
- AI-driven scientific breakthroughs
Expert Perspectives
Leading experts in the ai tech sector provide diverse perspectives on how the landscape will evolve over the coming years:
"The next frontier is AI systems that can reason across modalities and domains with minimal human guidance."
— AI Researcher
"Organizations that develop effective AI governance frameworks will gain competitive advantage."
— Industry Analyst
"The AI talent gap remains a critical barrier to implementation for most enterprises."
— Chief AI Officer
Areas of Expert Consensus
- Acceleration of Innovation: The pace of technological evolution will continue to increase
- Practical Integration: Focus will shift from proof-of-concept to operational deployment
- Human-Technology Partnership: Most effective implementations will optimize human-machine collaboration
- Regulatory Influence: Regulatory frameworks will increasingly shape technology development
Short-Term Outlook (1-2 Years)
In the immediate future, organizations will focus on implementing and optimizing currently available technologies to address pressing ai tech challenges:
- Improved generative models
- specialized AI applications
- enhanced AI ethics frameworks
These developments will be characterized by incremental improvements to existing frameworks rather than revolutionary changes, with emphasis on practical deployment and measurable outcomes.
Mid-Term Outlook (3-5 Years)
As technologies mature and organizations adapt, more substantial transformations will emerge in how security is approached and implemented:
- AI-human collaboration systems
- multimodal AI platforms
- democratized AI development
This period will see significant changes in security architecture and operational models, with increasing automation and integration between previously siloed security functions. Organizations will shift from reactive to proactive security postures.
Long-Term Outlook (5+ Years)
Looking further ahead, more fundamental shifts will reshape how cybersecurity is conceptualized and implemented across digital ecosystems:
- General AI capabilities
- AI-driven scientific breakthroughs
- new computing paradigms
These long-term developments will likely require significant technical breakthroughs, new regulatory frameworks, and evolution in how organizations approach security as a fundamental business function rather than a technical discipline.
Key Risk Factors and Uncertainties
Several critical factors could significantly impact the trajectory of ai tech evolution:
Organizations should monitor these factors closely and develop contingency strategies to mitigate potential negative impacts on technology implementation timelines.
Alternative Future Scenarios
The evolution of technology can follow different paths depending on various factors including regulatory developments, investment trends, technological breakthroughs, and market adoption. We analyze three potential scenarios:
Optimistic Scenario
Responsible AI driving innovation while minimizing societal disruption
Key Drivers: Supportive regulatory environment, significant research breakthroughs, strong market incentives, and rapid user adoption.
Probability: 25-30%
Base Case Scenario
Incremental adoption with mixed societal impacts and ongoing ethical challenges
Key Drivers: Balanced regulatory approach, steady technological progress, and selective implementation based on clear ROI.
Probability: 50-60%
Conservative Scenario
Technical and ethical barriers creating significant implementation challenges
Key Drivers: Restrictive regulations, technical limitations, implementation challenges, and risk-averse organizational cultures.
Probability: 15-20%
Scenario Comparison Matrix
Factor | Optimistic | Base Case | Conservative |
---|---|---|---|
Implementation Timeline | Accelerated | Steady | Delayed |
Market Adoption | Widespread | Selective | Limited |
Technology Evolution | Rapid | Progressive | Incremental |
Regulatory Environment | Supportive | Balanced | Restrictive |
Business Impact | Transformative | Significant | Modest |
Transformational Impact
Redefinition of knowledge work, automation of creative processes. This evolution will necessitate significant changes in organizational structures, talent development, and strategic planning processes.
The convergence of multiple technological trends—including artificial intelligence, quantum computing, and ubiquitous connectivity—will create both unprecedented security challenges and innovative defensive capabilities.
Implementation Challenges
Ethical concerns, computing resource limitations, talent shortages. Organizations will need to develop comprehensive change management strategies to successfully navigate these transitions.
Regulatory uncertainty, particularly around emerging technologies like AI in security applications, will require flexible security architectures that can adapt to evolving compliance requirements.
Key Innovations to Watch
Multimodal learning, resource-efficient AI, transparent decision systems. Organizations should monitor these developments closely to maintain competitive advantages and effective security postures.
Strategic investments in research partnerships, technology pilots, and talent development will position forward-thinking organizations to leverage these innovations early in their development cycle.
Technical Glossary
Key technical terms and definitions to help understand the technologies discussed in this article.
Understanding the following technical concepts is essential for grasping the full implications of the security threats and defensive measures discussed in this article. These definitions provide context for both technical and non-technical readers.