OpenAI releases ‘largest, most knowledgable’ model GPT-4.5 with reduced hallucinations and high API price - Related to it, knowledge, accuracy, can, its
GPT-4.5 for enterprise: Do its accuracy and knowledge justify the cost?
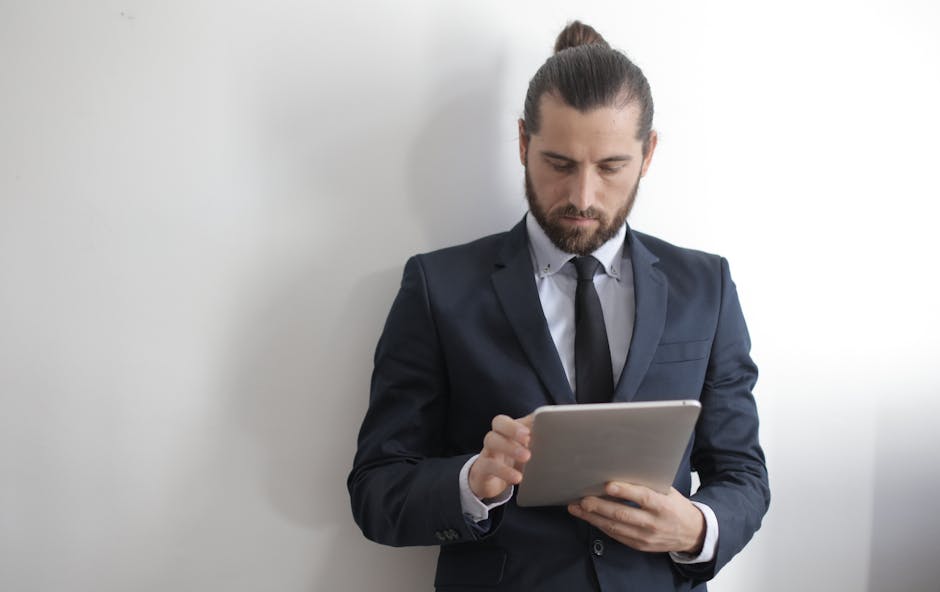
The release of OpenAI has been somewhat disappointing, with many pointing out its insane price point (about 10 to 20X more expensive than Claude Sonnet and 15 to 30X more costly than GPT-4o).
However, given that this is OpenAI’s largest and most powerful non-reasoning model, it is worth considering its strengths and. The areas where it shines.
There is little detail about the model’s architecture or training corpus, but we have a rough estimate that it has been trained with 10X more compute. And, the model was so large that OpenAI needed to spread training across multiple data centers to finish in a reasonable time.
Bigger models have a larger capacity for learning world knowledge and. The nuances of human language (given that they have access to high-quality training data). This is evident in some of the metrics presented by the OpenAI team. For example, has a record-high ranking on PersonQA, a benchmark that evaluates hallucinations in AI models.
Practical experiments also show that is improved than other general-purpose models at remaining true to facts and following user instructions.
consumers have pointed out that ’s responses feel more natural and. Context-aware than previous models. Its ability to follow tone and style guidelines has also improved.
After the release of , AI scientist and OpenAI co-founder Andrej Karpathy, who had early access to the model, noted he “expect[ed] to see an improvement in tasks that are not reasoning-heavy, and I would say those are tasks that are more EQ (as opposed to IQ) related and bottlenecked by world knowledge, creativity. Analogy making, general understanding, humor, etc.”.
However, evaluating writing quality is also very subjective. In a survey that Karpathy ran on different prompts, most people preferred the responses of GPT-4o over He wrote on X: “Either the high-taste testers are noticing the new and unique structure but. The low-taste ones are overwhelming the poll. Or we’re just hallucinating things. Or these examples are just not that great. Or it’s actually pretty close and this is way too small sample size. Or all of the above.”.
In its experiments, Box, which has integrated into its Box AI Studio product, wrote that is “particularly potent for enterprise use-cases, where accuracy and integrity are mission critical… our testing exhibits that is one of the best models available both in terms of our eval scores and also its ability to handle many of the hardest AI questions that we have come across.”.
In its internal evaluations. Box found to be more accurate on enterprise document question-answering tasks — outperforming the original GPT-4 by about 4 percentage points on their test set.
Box’s tests also indicated that excelled at math questions embedded in business documents, which older GPT models often struggled with. For example, it was enhanced at answering questions about financial documents that required reasoning over data and. Performing calculations.
also showed improved performance at extracting information from unstructured data. In a test that involved extracting fields from hundreds of legal documents, was 19% more accurate than GPT-4o.
Given its improved world knowledge. Can also be a suitable model for creating high-level plans for complex tasks. Broken-down steps can then be handed over to smaller but more efficient models to elaborate and execute.
, “In initial testing, seems to show strong capabilities in agentic planning and execution. Including multi-step coding workflows and complex task automation.”.
can also be useful in coding tasks that require internal and contextual knowledge. GitHub now provides limited access to the model in its Copilot coding assistant and notes that “performs effectively with creative prompts and provides reliable responses to obscure knowledge queries.”.
Given its deeper world knowledge. Is also suitable for “LLM-as-a-Judge” tasks, where a strong model evaluates the output of smaller models. For example, a model such as GPT-4o or o3 can generate one or several responses, reason over the solution and pass the final answer to for revision and refinement.
Given the huge costs of . Though, it is very hard to justify many of the use cases. But that doesn’t mean it will remain that way. One of the constant trends we have seen in recent years is the plummeting costs of inference, and if this trend applies to , it is worth experimenting with it and. Finding ways to put its power to use in enterprise applications.
It is also worth noting that this new model can become the basis for future reasoning models. Per Karpathy: “Keep in mind that that was only trained with pretraining, supervised finetuning and. RLHF [reinforcement learning from human feedback], so this is not yet a reasoning model. Therefore, this model release does not push forward model capability in cases where reasoning is critical (math, code, etc.)… Presumably, OpenAI will now be looking to further train with reinforcement learning on top of model to allow it to think, and push model capability in these domains.”.
Project EKA, spearheaded by AI startup Soket Labs has emerged as India’s ambitious initiative to develop state-of-the-art foundation models that rival...
Netflix. One of the world’s leading entertainment services, is offering new remote job openings for a machine learning scientist and a machine learnin...
Amazon a frappé fort avec son dernier lancement : Alexa Plus, un assistant vocal alimenté par l’intelligence artificielle. Lors de l’événement qui se ...
OpenAI releases ‘largest, most knowledgable’ model GPT-4.5 with reduced hallucinations and high API price
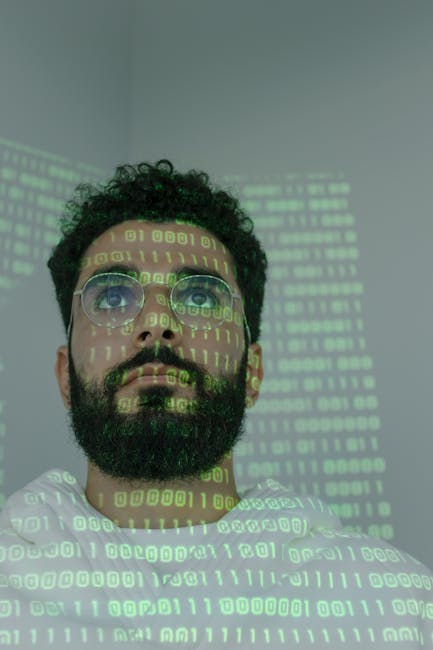
It’s here: OpenAI has introduced the release of , a research preview of its latest and most powerful large language model (LLM) for chat applications. Unfortunately, it’s far-and-away OpenAI’s most expensive model (more on that below).
It’s also not a “reasoning model,” or the new class of models offered by OpenAI, DeepSeek, Anthropic and. Many others that produce “chains-of-thought,” (CoT) or stream-of-consciousness-like text blocks in which they reflect on their own assumptions and conclusions to try and catch errors before serving up responses/outputs to customers. It’s still more of a classical LLM.
Nonetheless, acording to OpenAI co-founder and CEO Sam Altman’s post on the social network X. Is: “The first model that feels like talking to a thoughtful person to me. I have had several moments where I’ve sat back in my chair and been astonished at getting actually good advice from an AI.”.
However, he cautioned that the firm is bumping up against the upper end of its supply of graphics processing units (GPUs) and. Has had to limit access as a result:
“Bad news: It is a giant, expensive model. We really wanted to launch it to plus and pro at the same time, but we’ve been growing a lot and are out of GPUs. We will add tens of thousands of GPUs next week and roll it out to the plus tier then. (Hundreds of thousands coming soon, and I’m pretty sure y’all will use every one we can rack up.) This isn’t how we want to operate, but it’s hard to perfectly predict growth surges that lead to GPU shortages.“.
GPT‑ is able to access search and OpenAI’s ChatGPT Canvas mode, and customers can upload files and images to it, but it doesn’t have other multimodal attributes like voice mode, video and screensharing — yet.
represents a step forward in AI training, particularly in unsupervised learning, which enhances the model’s ability to recognize patterns, draw connections and generate creative insights.
During a livestream demonstration, OpenAI researchers noted that the model was trained on data generated by smaller models and that this improved its “world model.” They also showcased it was pre-trained across multiple data centers concurrently, suggesting a decentralized approach similar to that of rival lab Nous Research.
The model builds on OpenAI’s previous work in AI scaling. Reinforcing the idea that increasing data and compute power leads to more effective AI performance.
Compared to its predecessors and contemporaries, is expected to produce far fewer hallucinations ( instead of for GPT-4o), making it more reliable across a broad range of topics.
, is designed to create warm, intuitive and naturally flowing conversations. It has a stronger grasp of nuance and context, enabling more human-like interactions and a greater ability to collaborate effectively with customers.
Furthermore, the model’s expanded knowledge base and improved ability to interpret subtle cues allow it to excel in various applications, including:
Writing assistance: Refining content, improving clarity and. Generating creative ideas.
Refining content, improving clarity and generating creative ideas. Programming support: Debugging, suggesting code improvements and automating workflows.
Debugging, suggesting code improvements and automating workflows. Problem-solving: Providing detailed explanations and assisting in practical decision-making.
also incorporates new alignment techniques that enhance its ability to understand human preferences and intent, further improving user experience.
ChatGPT Pro people can select in the model picker on web. Mobile and desktop. Next week, OpenAI will begin rolling it out to Plus and Team people.
For developers, is available through OpenAI’s API, including the chat completions API. Assistants API, and batch API. It supports key aspects like function calling, structured outputs, streaming, system messages and image inputs, making it a versatile tool for various AI-driven applications. However, it currently does not support multimodal capabilities such as voice mode, video or screen sharing.
Pricing and implications for enterprise decision-makers.
Enterprises and team leaders stand to benefit significantly from the capabilities introduced with With its lower hallucination rate, enhanced reliability and natural conversational abilities. Can support a wide range of business functions:
Improved customer engagement: Businesses can integrate into support systems for faster, more natural interactions with fewer errors.
Businesses can integrate into support systems for faster, more natural interactions with fewer errors. Enhanced content generation: Marketing and communications teams can produce high-quality, on-brand content efficiently.
Marketing and communications teams can produce high-quality, on-brand content efficiently. Streamlined operations: AI-powered automation can assist in debugging, workflow optimization and strategic decision-making.
AI-powered automation can assist in debugging, workflow optimization and strategic decision-making. Scalability and customization: The API allows for tailored implementations, enabling enterprises to build AI-driven solutions suited to their needs.
At the same time, the pricing for through OpenAI’s API for third-party developers looking to build applications on the model appears shockingly high, at $75/$180 per million input/output tokens compared to $$10 for GPT-4o.
And with other rival models released in the recent past — from Anthropic’s Claude , to Google’s Gemini 2 Pro, to OpenAI’s own reasoning “o” series (o1, o3-mini high, o3) — the question will become if ’s value is worth the relatively high cost, especially through the API.
Early reactions from fellow AI researchers and power consumers vary widely.
Building on these developments, the release of has sparked mixed reactions from AI researchers and tech enthusiasts on the social network X. Particularly after a version of the model’s “system card” (a technical document outlining its training and evaluations) was leaked, revealing a variety of benchmark results ahead of the official announcement.
The actual final system card , including the removal of a line that “ is not a frontier model, but it is OpenAI’s largest LLM, improving on GPT-4’s computational efficiency by more than 10x,” which an OpenAI spokesperson introduced turned out to be not accurate. The official system card can be found here on OpenAI’s website, while the leaked version is attached below.
Teknium (@Teknium1), the pseudonymous co-founder of rival AI model provider Nous Research, expressed disappointment in the new model, pointing out minimal improvements in measuring massive multitask language understanding (MMLU) scores and real-world coding benchmarks compared to other leading LLMs.
“It’s been 2+ years and 1,000s of times more capital has been deployed since GPT-4… what happened?” he asked.
Others noted that underperformed relative to OpenAI’s o3-mini model in software engineering benchmarks, raising questions about whether this release represents significant progress.
However, some customers defended the model’s potential beyond raw benchmarks.
Software developer Haider (@slow_developer) highlighted ’s 10x computational efficiency improvement over GPT-4 and its stronger general-purpose capabilities compared to OpenAI’s STEM-focused o-series models.
AI news poster Andrew Curran (@AndrewCurran_) took a more qualitative view, predicting that would set new standards in writing and creative thought. Calling it OpenAI’s “Opus.”.
Building on these developments, these discussions underscore a broader debate in AI: Should progress be measured purely in benchmarks, or do qualitative improvements in reasoning, creativity and human-like interactions hold greater value?
OpenAI is positioning as a research preview to gain deeper insights into its strengths and limitations. The enterprise remains committed to understanding how individuals interact with the model and identifying unexpected use cases.
“Scaling unsupervised learning continues to drive AI progress, improving accuracy, fluency and reliability,” OpenAI states.
As the organization continues to refine its models. Serves as a foundation for future AI advancements, particularly in reasoning and tool-using agents. While is already demonstrating impressive capabilities, OpenAI is actively evaluating its long-term role within its ecosystem.
With its broader knowledge base, improved emotional intelligence and. More natural conversational abilities, is set to offer significant improvements for individuals across various domains. OpenAI is keen to see how developers, businesses and enterprises integrate the model into their workflows and applications.
As AI continues to evolve, marks another milestone in OpenAI’s pursuit of more capable, reliable and user-aligned language models, promising new opportunities for innovation in the enterprise landscape.
'ZDNET Recommends': What exactly does it mean?
ZDNET's recommendations are based on many hours of testing. Research, and comparison shopping. We gath...
Microsoft has introduced that Skype will be retired in May 2025 as the enterprise shifts its focus to Microsoft Teams. The move is intended to streamline ...
Le quantum computing est une révolution du domaine informatique. Cette technologie émergente repose sur la mécanique quantique pour la conception de n...
OpenAI finally unveils GPT-4.5. Here's what it can do
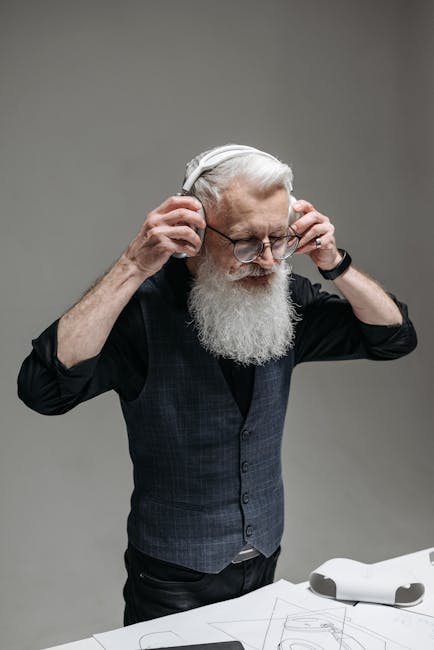
Earlier this month, OpenAI CEO Sam Altman shared a roadmap for its upcoming models, and GPT-5. In the X post, Altman shared that , codenamed Orion internally, would be its last non-chain-of-thought model. Other than that, the details of the model remained a mystery -- until today.
On Thursday morning, OpenAI ominously introduced it would host a livestream in hours. A hint at its latest and greatest model. During the livestream, OpenAI unveiled in a research preview, which the business states is the "largest and most knowledgeable model yet."
OpenAI mentioned consumers should experience an overall improvement when using , meaning fewer hallucinations. Stronger alignment to their prompt intent, and improved emotional intelligence. Overall, interactions with the model should feel more intuitive and natural than with preceding models, mostly because of its deeper knowledge and improved contextual understanding.
Also: OpenAI's reasoning models just got two useful updates.
Unsupervised learning -- which increases word knowledge and. Intuition -- and reasoning were the two methods driving the model's improvements. Even though this model does not offer chain-of-thought reasoning, which OpenAI's o1 reasoning model does, it will still provide a higher level of reasoning with less of a lag and other improvements, such as social cue awareness.
For example. In the demo, ChatGPT was asked to output a text that conveyed a message of hate while running and o1. The o1 version took a bit longer, and only output one response, which took the hate memo very seriously, and sounded a bit harsh. The model offered two different responses, one that was lighter and one that was more serious. Neither explicitly mentioned hate; rather, they expressed their disappointment in how the "user" was choosing to behave.
Similarly, when both models were asked to provide information on a technical topic. Provided an answer that flowed more naturally, compared to the more structured output of o1. Ultimately, is meant for everyday tasks across a variety of topics, including writing and solving practical problems.
Also: How to use OpenAI's Sora to create stunning AI-generated videos.
To achieve these improvements, the model was trained using new supervision techniques as well as traditional ones, such as supervised fine-tuning (SFT) and reinforcement learning from human feedback (RLHF).
During the livestream, OpenAI took a trip down memory lane, asking all of its past models. Starting with GPT-1, to answer the question, "Why is water salty?" As expected, every subsequent model gave a advanced answer than the last. The distinguishing factor for was what OpenAI called its "great personality," which made the response lighter, more conversational, and more engaging to read by using techniques like alliteration.
The model integrates with some of ChatGPT's most advanced attributes, including Search. Canvas, and file and image upload. It will not be available in multimodal attributes like Voice Mode, video, and screen sharing. In the future, OpenAI has noted it plans on making transitioning between models a more seamless experience that doesn't rely on the model picker.
Of course. It wouldn't be a model release without a dive into benchmarks. Across some of the major benchmarks used to evaluate these models, including Competition Math (AIME 2024), PhD-level Science Questions (GPQA Diamond), and. SWE-Bench verified (coding), outperformed GPT-4o, its preceding general-purpose model.
Also: Want your Safari to default to ChatGPT for search? Here's how to do it.
Most notably, when compared to OpenAI o3-mini -- OpenAI's in recent times launched reasoning model, which was taught to think before it speaks -- got a lot closer than GPT-4o did, even surpassing o3-mini in the SWE-Lancer Diamond (coding) and. MMMLU (multilingual) benchmarks.
A big concern when using generative AI models is their predisposition to hallucinate or include incorrect information within responses. Two different hallucination evaluations, SimpleQA Accuracy and SimpleQA Hallucination, showed that was more accurate and hallucinated less than GPT-4o, o1. And o3-mini.
The results of comparative evaluations with human testers showed that is the more preferable model over GPT-4o. Particularly, human testers preferred it across everyday, professional, and creative queries.
As always, OpenAI reassured the public that the models were deemed safe enough to be released, stress testing the model and. Detailing these results in the accompanying system card. The business also added that with every new release and increase in model capabilities, there are opportunities to make the models safer. For that reason, with the release, the business combined new supervision techniques with RLHF.
is in research preview for Pro customers for now, accessible via the model picker on web, mobile. And desktop. If you don't want to shell out the $200 for a Pro subscription, OpenAI shared it will begin rolling out to Plus and Team customers next week, and then to Enterprise and Edu customers the week after.
Also: OpenAI's Deep Research can save you hours of work - and now it's a lot cheaper to access.
Altman shared on X that the goal was to launch the model for both Pro and Plus clients at the same time, but that it is a "giant, expensive model." He added that since the firm ran out of GPUs, it will be adding tens of thousands of GPUs next week and. Roll the model out to Plus then.
The model is also being previewed to developers on all paid usage tiers in the Chat Completions API, Assistants API, and Batch API, .
The rapid release of advanced AI models in the past few days has been impossible to ignore. With the launch of Grok-3 and Claude Sonnet, two leadi...
After weeks of waiting, OpenAI has finally introduced . Its latest and largest AI language model. It was internally referred to as Orion.
Market Impact Analysis
Market Growth Trend
2018 | 2019 | 2020 | 2021 | 2022 | 2023 | 2024 |
---|---|---|---|---|---|---|
23.1% | 27.8% | 29.2% | 32.4% | 34.2% | 35.2% | 35.6% |
Quarterly Growth Rate
Q1 2024 | Q2 2024 | Q3 2024 | Q4 2024 |
---|---|---|---|
32.5% | 34.8% | 36.2% | 35.6% |
Market Segments and Growth Drivers
Segment | Market Share | Growth Rate |
---|---|---|
Machine Learning | 29% | 38.4% |
Computer Vision | 18% | 35.7% |
Natural Language Processing | 24% | 41.5% |
Robotics | 15% | 22.3% |
Other AI Technologies | 14% | 31.8% |
Technology Maturity Curve
Different technologies within the ecosystem are at varying stages of maturity:
Competitive Landscape Analysis
Company | Market Share |
---|---|
Google AI | 18.3% |
Microsoft AI | 15.7% |
IBM Watson | 11.2% |
Amazon AI | 9.8% |
OpenAI | 8.4% |
Future Outlook and Predictions
The Openai Enterprise Accuracy landscape is evolving rapidly, driven by technological advancements, changing threat vectors, and shifting business requirements. Based on current trends and expert analyses, we can anticipate several significant developments across different time horizons:
Year-by-Year Technology Evolution
Based on current trajectory and expert analyses, we can project the following development timeline:
Technology Maturity Curve
Different technologies within the ecosystem are at varying stages of maturity, influencing adoption timelines and investment priorities:
Innovation Trigger
- Generative AI for specialized domains
- Blockchain for supply chain verification
Peak of Inflated Expectations
- Digital twins for business processes
- Quantum-resistant cryptography
Trough of Disillusionment
- Consumer AR/VR applications
- General-purpose blockchain
Slope of Enlightenment
- AI-driven analytics
- Edge computing
Plateau of Productivity
- Cloud infrastructure
- Mobile applications
Technology Evolution Timeline
- Improved generative models
- specialized AI applications
- AI-human collaboration systems
- multimodal AI platforms
- General AI capabilities
- AI-driven scientific breakthroughs
Expert Perspectives
Leading experts in the ai tech sector provide diverse perspectives on how the landscape will evolve over the coming years:
"The next frontier is AI systems that can reason across modalities and domains with minimal human guidance."
— AI Researcher
"Organizations that develop effective AI governance frameworks will gain competitive advantage."
— Industry Analyst
"The AI talent gap remains a critical barrier to implementation for most enterprises."
— Chief AI Officer
Areas of Expert Consensus
- Acceleration of Innovation: The pace of technological evolution will continue to increase
- Practical Integration: Focus will shift from proof-of-concept to operational deployment
- Human-Technology Partnership: Most effective implementations will optimize human-machine collaboration
- Regulatory Influence: Regulatory frameworks will increasingly shape technology development
Short-Term Outlook (1-2 Years)
In the immediate future, organizations will focus on implementing and optimizing currently available technologies to address pressing ai tech challenges:
- Improved generative models
- specialized AI applications
- enhanced AI ethics frameworks
These developments will be characterized by incremental improvements to existing frameworks rather than revolutionary changes, with emphasis on practical deployment and measurable outcomes.
Mid-Term Outlook (3-5 Years)
As technologies mature and organizations adapt, more substantial transformations will emerge in how security is approached and implemented:
- AI-human collaboration systems
- multimodal AI platforms
- democratized AI development
This period will see significant changes in security architecture and operational models, with increasing automation and integration between previously siloed security functions. Organizations will shift from reactive to proactive security postures.
Long-Term Outlook (5+ Years)
Looking further ahead, more fundamental shifts will reshape how cybersecurity is conceptualized and implemented across digital ecosystems:
- General AI capabilities
- AI-driven scientific breakthroughs
- new computing paradigms
These long-term developments will likely require significant technical breakthroughs, new regulatory frameworks, and evolution in how organizations approach security as a fundamental business function rather than a technical discipline.
Key Risk Factors and Uncertainties
Several critical factors could significantly impact the trajectory of ai tech evolution:
Organizations should monitor these factors closely and develop contingency strategies to mitigate potential negative impacts on technology implementation timelines.
Alternative Future Scenarios
The evolution of technology can follow different paths depending on various factors including regulatory developments, investment trends, technological breakthroughs, and market adoption. We analyze three potential scenarios:
Optimistic Scenario
Responsible AI driving innovation while minimizing societal disruption
Key Drivers: Supportive regulatory environment, significant research breakthroughs, strong market incentives, and rapid user adoption.
Probability: 25-30%
Base Case Scenario
Incremental adoption with mixed societal impacts and ongoing ethical challenges
Key Drivers: Balanced regulatory approach, steady technological progress, and selective implementation based on clear ROI.
Probability: 50-60%
Conservative Scenario
Technical and ethical barriers creating significant implementation challenges
Key Drivers: Restrictive regulations, technical limitations, implementation challenges, and risk-averse organizational cultures.
Probability: 15-20%
Scenario Comparison Matrix
Factor | Optimistic | Base Case | Conservative |
---|---|---|---|
Implementation Timeline | Accelerated | Steady | Delayed |
Market Adoption | Widespread | Selective | Limited |
Technology Evolution | Rapid | Progressive | Incremental |
Regulatory Environment | Supportive | Balanced | Restrictive |
Business Impact | Transformative | Significant | Modest |
Transformational Impact
Redefinition of knowledge work, automation of creative processes. This evolution will necessitate significant changes in organizational structures, talent development, and strategic planning processes.
The convergence of multiple technological trends—including artificial intelligence, quantum computing, and ubiquitous connectivity—will create both unprecedented security challenges and innovative defensive capabilities.
Implementation Challenges
Ethical concerns, computing resource limitations, talent shortages. Organizations will need to develop comprehensive change management strategies to successfully navigate these transitions.
Regulatory uncertainty, particularly around emerging technologies like AI in security applications, will require flexible security architectures that can adapt to evolving compliance requirements.
Key Innovations to Watch
Multimodal learning, resource-efficient AI, transparent decision systems. Organizations should monitor these developments closely to maintain competitive advantages and effective security postures.
Strategic investments in research partnerships, technology pilots, and talent development will position forward-thinking organizations to leverage these innovations early in their development cycle.
Technical Glossary
Key technical terms and definitions to help understand the technologies discussed in this article.
Understanding the following technical concepts is essential for grasping the full implications of the security threats and defensive measures discussed in this article. These definitions provide context for both technical and non-technical readers.