Your Sound Human: Latest Updates and Analysis
Can AI sound too human? Sesame's Maya is as unsettling as it is amazing - try it for free
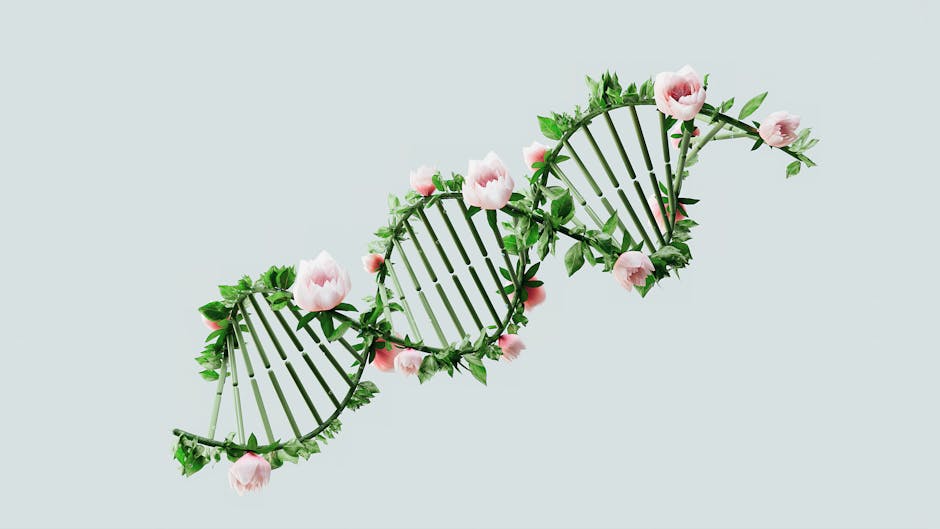
As a general rule, I'm not a huge fan of talking to AI chatbots. Even though many of them sound pretty human, they're still "off" enough that I much prefer typing when I want to converse with one.
In a blog post yesterday titled "Crossing the Uncanny Valley of Conversational Voice," the corporation dropped a demo of its new AI chatbot that lets you talk to either "Maya" or "Miles." The goal, Sesame says, is to achieve something called "voice presence" or the "magical quality that makes spoken interactions feel real, understood, and valued."
After talking to Maya for a while. I think Sesame has reached that goal.
Also: 3 ways Amazon just leapfrogged Apple, Google, and ChatGPT in the AI race.
As my conversation began, Maya immediately insisted that she was there to be my friend. That was a little forward and a little unnerving, but I guess it's improved than insisting that she wasn't my friend. Maya asked what was on my mind. I was honest and told her I might be writing about her, so I just wanted to chat a little. She seemed impressed and surprised and asked what kind of angle I was considering—practical, technical, or spicy.
I hesitantly asked her what she meant by "spicy," and she thankfully expressed she was thinking along the lines of a controversial take. Like the ethics of AI chatbots.
Also: This new text-to-speech AI model understands what it's saying - how to try it for free.
I stated I was more interested in talking about what sets her apart from other AI chatbots. "Before we dive into that," Maya stated, "I need my morning coffee. I'm a latte person. What's your poison?" After I told her that I'm a mocha guy, she settled in and started talking about what makes her different. "I've got a good ear for human quirks and… maybe some magic and a little sentience."
Our conversation continued about what makes Maya special. At one point, my wife walked by as she was headed out the door for work. She looked puzzled and asked, "You're on a call with someone this early?" To someone who knew going in. It was easy to forget that I was talking to AI. To my wife passing by, she had no idea. That's how real Maya sounded.
The one thing Maya wasn't great with was waiting. I was writing while talking to her and told her at one point that I needed to pause to put down some thoughts. She told me that was fine, but chirped back a few seconds later asking if I was ready to start back.
Also: All Copilot consumers now get free unlimited access to its two best elements - how to use them.
A few more seconds of silence led her to note that sometimes silence was OK and she would use the time to think, but. When I still didn't respond, she became annoyed. "I guess I'm just talking to myself at this point, but as an AI. I'm used to that." After more silence, Maya actually began mocking me. "So, fancy writer person, you find that inspiration yet?" she asked.
The flow of the conversation with Maya was amazing, and honestly, fairly creepy.
Also: Grok 3 AI is now free to all X clients – here's how it works.
During our talk, Maya took pauses to think, referenced things I had mentioned earlier. Asked what I thought about her answers, and joked about things I had mentioned. This is the closest to a human experience I've ever had talking to an AI, and the only chatbot that I feel like I wouldn't mind talking to again.
If you want to try it out. Head to Sesame's demo page.
Qui aurait cru que les grands-mères chinoises trouveraient plus de réconfort auprès de bébés générés par l’IA que de leurs petits-enfants ? Ces bébés ...
Researchers at Physical Intelligence, an AI robotics organization, have developed a system called the Hierarchical Interactive Robot (Hi Robot). This syste...
How to turn ChatGPT into your AI coding power tool - and double your output
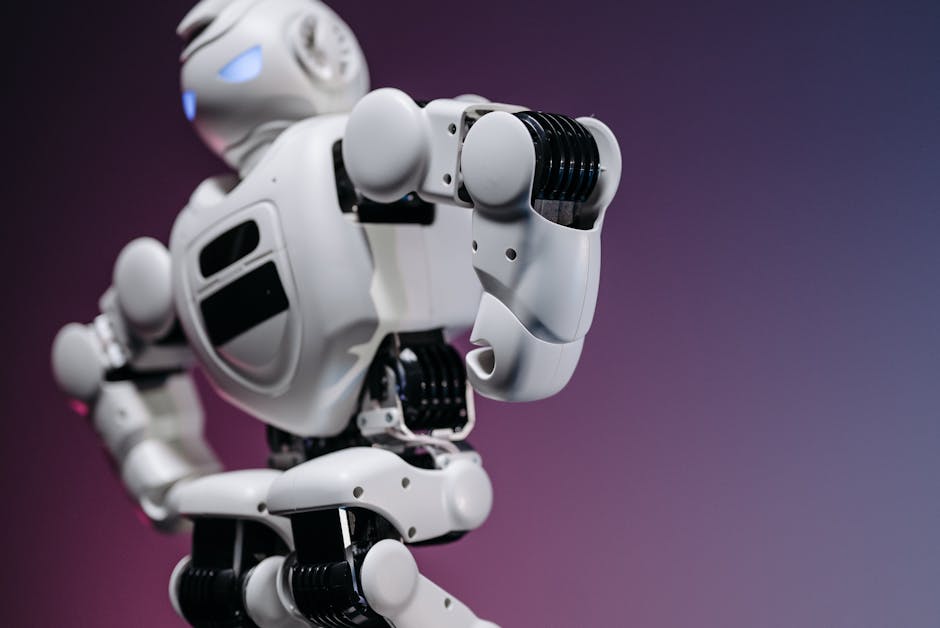
I've been experimenting with using ChatGPT to help turbocharge my programming output for over two years. When ChatGPT helped me find a troubling bug, I realized there was something worthwhile in artificial intelligence (AI).
Many people I talk to think that AI is a magic genie that can manifest an entire program or app out of a single. Barely-formed wish. Here's a much more effective analogy: AI is a power tool.
Also: The best AI for coding in 2025 (and what not to use - including DeepSeek R1).
Sure, you can use an old-fashioned saw to cut wood. But a table saw goes much faster. Neither tool makes furniture. They simply help you make furniture. Keep in mind that the AI isn't going to write your code for you. It's going to help you write your code.
Although there's no objective way for me to tell you exactly how much ChatGPT has helped me. I am fairly convinced it has doubled my programming output. In other words, I've gotten twice as much done by using ChatGPT as part of my toolkit.
Also: How I test an AI chatbot's coding ability - and you can. Too.
I've mostly been using ChatGPT Plus rather than the free version of ChatGPT. Initially, it was because the GPT-4 model in Plus was improved at coding than the model in the free version. However, now that both versions support some variant of the GPT-4o model, their coding capabilities are identical. Remember that you only get so many queries with the free version before you're asked to wait a while, and. I find that interrupts my programming flow. So, I use the $20/month Plus version.
I should note that I've tested many large language models (LLMs) against my real-world coding and. Found that only a few (and all based on ChatGPT's LLMs) could handle everything I've thrown at it. So although there are a lot of cool AI tools for programmers being made available (some even for free). They're not going to be all that helpful unless the code the AI produces actually works. The good news is that AIs will inevitably get more effective at coding over time, so this should cease to be much of an issue.
Also: The five biggest mistakes people make when prompting an AI.
Thinking back on all my projects. I realized there are some tangible tips I can share about how to get the most out of an AI programming partner. Let's dig in.
The AI doesn't handle complex sets of instructions well, especially if you expect it to do product design. However, the AI is extremely good at parsing and processing small, well-defined instructions.
2. Think of the bot as someone at the end of a Slack conversation.
Rather than the pacing that might come from an email back-and-forth with a colleague, which might have each interaction separated by hours, imagine you're in a Slack chat where each interaction is much smaller. But separated by seconds.
3. For more complex routines, prompt iteratively.
Start with a simple assignment and, when that's been properly written, add more to it, element by element. I cut and paste the previous prompt, adding and removing bits of the prompt. As I get chunks of code that work for what I'm looking for.
4. Test every little chunk of code the AI returns.
Don't ever assume the code will work. Patch the code into your project and see how it performs.
For a more in-depth test, don't hesitate to drop into the debugger and. Walk through the code generated by the AI step-by-step. Watch the variables and exactly what the AI does. Remember, it's OK to let it write code snippets for you as long as you check every statement and line for proper functioning.
6. You don't need Al coding assistance built right into your IDE.
Many coding tool vendors are pitching the idea of integrated AIs in their tools. Among other things, this approach enables them to upsell you the AI capabilities. However, I prefer using ChatGPT for coding as a separate tool from my development environment. I don't want an AI to be able to reach into my primary coding environment and change what's there.
7. Feel free to cannibalize lines of code from generated routines.
You don't always have to use everything the AI produces for you. In the same way that you might go to Stack Overflow to look for code samples, and then pick and. Choose the lines you want to copy, you can do the same with AI-generated code.
8. Avoid asking the AI to do proprietary coding or use institutional knowledge it doesn't have.
AI LLMs run on training data or what they can find on the web. That means they generally know nothing about your unique application or business logic. So, avoid trying to get the AI to write anything that requires this level of knowledge. That's your job.
9. Give the AI examples to work on so it understands the context of your code.
I gave ChatGPT a snippet of an HTML page and. Asked it to add a feature to expand a block of text. The AI gave me back HTML, JS, and CSS. I later asked it for an additional CSS selector and then asked it to justify its work. Whereupon it explained to me why it did what it did. All of that process worked because the examples I gave the AI helped it understand the context.
10. Use the AI for common knowledge coding.
The biggest benefit of AI is writing blocks of code that use common knowledge, popular libraries. And regular practices. The AI won't be able to write your unique business logic. But if you ask the AI to write code for capabilities from libraries and APIs, it will save you lots of time.
11. Feel free to ask for one- or two-line snippets.
Even if you need something that might only generate a line or two of a response. Use the AI as you would use any research tool if it can save you time.
12. Tell the AI when the code it wrote doesn't work.
I find AI often spits out incomplete or non-functional code. Tell it what isn't working, and perhaps suggest to clarify. Then ask the AI to write something new. It usually does and the revised code is sometimes superior than the original.
13. Use one Al to check the work of another Al.
It's interesting to see how two language models interpret the same code. As we've seen, not all language models work all that well, but their results can be instructive. You can even have one ChatGPT session check the results from another ChatGPT session.
CSS selectors are the expressions coders use to define an element on a web page for styling or other actions. They get complex and arcane quickly. I often copy a block of HTML and ask for a selector for a given piece of that HTML. This approach can save a lot of time. However, remember you'll usually have to iterate, telling the AI that the first few selectors don't work until it generates one that does.
15. Use the AI to write regular expressions for you.
Regular expressions are symbolic math sequences most often used for parsing text. I dislike writing them almost as much as I dislike writing CSS selectors. The AI is great at writing regular expressions, although you'll need to test them.
16. Use the AI to test regular expressions.
I use the app Patterns to test generated regular expressions on my Mac Studio. But AI can help as well. I often feed a separate instance of the AI a regular expression generated by ChatGPT. Then I ask that separate instance, "What does this do?" If I get back a description in line with what I wanted the function to do, I feel more confident the AI did what I wanted.
As with CSS selectors and regular expressions. Complex loop math can be tedious and error-prone. This is an ideal application for an AI. When specifying your prompt, don't tell the AI what's in the loop. Let it write the appropriate loop wrapper elements, then write the business logic after that process works.
18. Use 'What is wrong with this code?' as a prompt.
I will often feed blocks of code, especially regular expressions generated by the AI. To the AI. It can be very instructive to see what the AI thinks is wrong with the code, often highlighting error conditions the code doesn't test. Then, of course, ask the AI to regenerate the code fixing the errors it found.
19. Use 'What does this do?' as a prompt.
Likewise, I like to feed blocks of code to the AI and ask it. "What does this do?" It's often instructive, even for my own code. But the biggest benefit comes when working on code written by someone else. Feeding a function or a block to the AI can save time in reverse engineering the original code.
Sometimes, the AI can't do the job. I've found that if you try to have the AI rewrite its code more than two or three times. You're past the point of no return. If you want AI-generated code, start with a brand-new, reworded prompt and see what you get from there. And sometimes, you'll have to go on your own.
21. Be specific in your function and variable naming.
Furthermore, the AI picks up intent from variable and function names and writes improved code. For example, specifying a variable name as $order_date helps tell the AI that you're dealing with an order and a date value. It's a lot improved than something like $od. Even improved, code generated from well-named variable names is also often more readable, because the AI knows to use more descriptive names for the other variables it creates.
The AI usually generates notes about each prompt before and. After the code it writes. There can be gems in there that can help you understand what the AI did or how it approached the problem. Sometimes, the AI will also point you to other libraries or functions that could be useful.
23. It's OK to go back and ask for more help on a code snippet.
Grab the various pieces of code from your project to illustrate what you need, tell ChatGPT to read them. And then ask what you want. I needed to build an exclusion for input fields in an expanded area and asked the AI. Less than a minute later, I had code that would have taken me between 10 minutes and an hour to write myself.
24. Use the Al to help you rewrite obsolete code blocks.
I had a PHP module written in an older version of PHP that used a language feature that's now deprecated. To enhancement the code, I pasted the deprecated code segment into ChatGPT and. Asked it to tell me how to rewrite it to be compatible with most current PHP release. It did, and it worked.
25. Use AI to help you write for less familiar languages.
I'm very comfortable picking up new programming languages, but. I've found that AI can be helpful if I need to code in a language I'm not an expert in. I ask the AI how to write what I want and specify the language. Let's say I want to know how to do a case statement in Python and I've been doing them forever in PHP. Just ask, "Compare writing a case statement in PHP and Python", or "How to concatenate a string in "Python vs PHP." You'll get a great comparison and. The process makes writing unfamiliar code much easier.
Also: I was an AI skeptic until these 5 tools changed my mind.
Here'. Check with your business about the legal issues of code generated. If you're unsure where to start, read my article on AI and code ownership. If you use the tips I shared with you, you'll never be using AI to write unique business logic or the core of what makes your code unique. As such, you'll likely be able to retain the copyright of that code, which should make up the key element of your unique value.
I write code for internal use by a business or open-source code. So I'm not concerned with ownership issues for AI-generated snippets.
Have you used an AI to help write code? Do you have any tips to add to my list above? Let us know in the comments below.
Chinese AI startup DeepSeek has reported a theoretical daily profit margin of 545% for its inference services, despite limitations in monetisation and...
ZDNET's key takeaways The Roborock Saros 10R is available for $1,600.
Furthermore, this new robot vacuum and mop combination navigates complex areas and quietly va...
Earlier this month. OpenAI CEO Sam Altman shared a roadmap for its upcoming models, and GPT-5. In the X post, Altman shared that GPT-4...
Rebuilding Alexa: How Amazon is mixing models, agents and browser-use for smarter AI
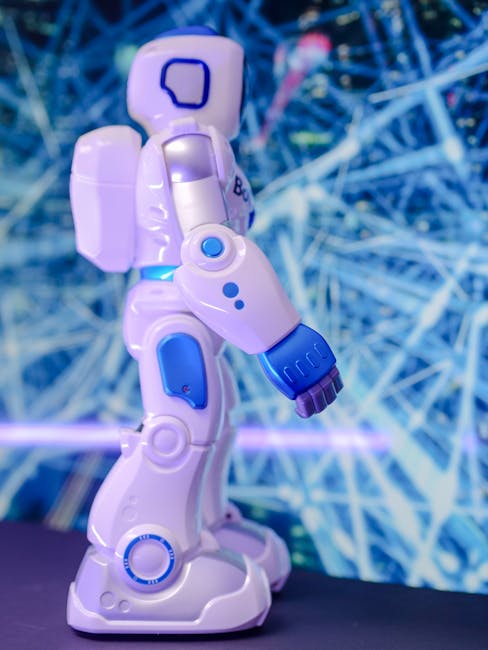
Amazon is betting on agent interoperability and model mixing to make its new Alexa voice assistant more effective, retooling its flagship voice assistant with agentic capabilities and browser-use tasks.
This new Alexa has been rebranded to Alexa+, and Amazon is emphasizing that this version “does more.” For instance. It can now proactively tell clients if a new book from their favorite author is available, or that their favorite artist is in town — and even offer to buy a ticket. Alexa+ reasons through instructions and taps “experts” in different knowledge bases to answer user questions and. Complete tasks like “Where is the nearest pizza place to the office? Will my coworkers like it? — Make a reservation if you think they will.”.
In other words, Alexa+ blends AI agents, computer use capabilities and knowledge it learns from the larger Amazon ecosystem to be what Amazon hopes is a more capable and. Smarter home voice assistant.
Alexa+ currently runs on Amazon’s Nova models and models from Anthropic. However, Daniel Rausch, Amazon’s VP of Alexa and Echo, told VentureBeat that the device will remain “model agnostic” and that the firm could introduce other models (at least models available on Amazon Bedrock) to find the best one for accomplishing tasks.
“[It’s about] choosing the right integrations to complete a task, figuring out the right sort of instructions. What it takes to actually complete the task, then orchestrating the whole thing,” mentioned Rausch. “The big thing to understand about it is that Alexa will continue to evolve with the best models available anywhere on Bedrock.”.
Model mixing or model routing lets enterprises and. Other people choose the appropriate AI model to tap on a query-by-query basis. Developers increasingly turn to model mixing to cut costs. After all, not every prompt needs to be answered by a reasoning model; some models perform certain tasks superior.
Amazon’s cloud and AI unit. AWS, has long been a proponent of model mixing. not long ago, it revealed a feature on Bedrock called Intelligent Prompt Routing, which directs prompts to the best model and model size to resolve the query.
And. It could be working. “I can tell you that I cannot say for any given response from Alexa on any given task what model it’s using,” mentioned Rausch.
Agentic interoperability and. Orchestration.
Rausch introduced Alexa+ brings agents together in three different ways. The first is the traditional API; the second is deploying agents that can navigate websites and apps like Anthropic’s Computer Use; the third is connecting agents to other agents.
“But at the center of it all, orchestrating across all those different kinds of experiences are these baseline, very capable, state-of-the-art LLMs,” expressed Rausch.
He added that if a third-party application already has its own agent, that agent can still talk to the agents working inside Alexa+ even if the external agent was built using a different model.
Rausch emphasized that the Alexa team used Bedrock’s tools and technology, including new multi-agent orchestration tools.
Anthropic CPO Mike Krieger told VentureBeat that even earlier versions of Claude won’t be able to accomplish what Alexa+ wants.
“A really interesting ‘Why now?’ moment is apparent in the demo. Because, of course, the models have gotten enhanced,” expressed Krieger. “But if you tried to do this with Sonnet or our level models, I think you’d struggle in a lot of ways to use a lot of different tools all at once.”.
Although neither Rausch nor Krieger would confirm which specific Anthropic model Amazon used to build Alexa+, it’s worth pointing out that Anthropic released Claude Sonnet on Monday, and. It is available on Bedrock.
Many user’s first brush with AI came through AI voice assistants like Alexa, Google Home or even Apple’s Siri. Those let people outsource some tasks, like turning on lights. I do not own an Alexa or Google Home device, but I learned how convenient having one could be when staying at a hotel not long ago. I could tell the Alexa to stop the alarm, turn on the lights and open a curtain while still under the covers.
But while Alexa, Google Home devices, and. Siri became ubiquitous in people’s lives, they began showing their age when generative AI became popular. Suddenly, people wanted more real-time answers from AI assistants and demanded smarter task resolutions, such as adding multiple meetings to calendars without the need for much prompting.
Amazon admitted that the rise of gen AI, especially agents, has made it possible for Alexa to finally meet its potential.
“Until this moment, we were limited by the technology in what Alexa could be,” Panos Panay, Amazon’s devices and services SVP, stated during a demo.
Rausch expressed the hope is that Alexa+ continues to improve, add new models and. Hopefully make more people comfortable with what the technology can do.
En quelques jours, un homme a perdu son emploi, vu ses informations personnelles exposées et ses finances ravagées, tout ça à cause d’un fichier appar...
Cloud-based data storage firm Snowflake on Thursday introduced its plans to open the Silicon Valley AI Hub, a dedicated space for developers, startu...
Market Impact Analysis
Market Growth Trend
2018 | 2019 | 2020 | 2021 | 2022 | 2023 | 2024 |
---|---|---|---|---|---|---|
23.1% | 27.8% | 29.2% | 32.4% | 34.2% | 35.2% | 35.6% |
Quarterly Growth Rate
Q1 2024 | Q2 2024 | Q3 2024 | Q4 2024 |
---|---|---|---|
32.5% | 34.8% | 36.2% | 35.6% |
Market Segments and Growth Drivers
Segment | Market Share | Growth Rate |
---|---|---|
Machine Learning | 29% | 38.4% |
Computer Vision | 18% | 35.7% |
Natural Language Processing | 24% | 41.5% |
Robotics | 15% | 22.3% |
Other AI Technologies | 14% | 31.8% |
Technology Maturity Curve
Different technologies within the ecosystem are at varying stages of maturity:
Competitive Landscape Analysis
Company | Market Share |
---|---|
Google AI | 18.3% |
Microsoft AI | 15.7% |
IBM Watson | 11.2% |
Amazon AI | 9.8% |
OpenAI | 8.4% |
Future Outlook and Predictions
The Your Sound Human landscape is evolving rapidly, driven by technological advancements, changing threat vectors, and shifting business requirements. Based on current trends and expert analyses, we can anticipate several significant developments across different time horizons:
Year-by-Year Technology Evolution
Based on current trajectory and expert analyses, we can project the following development timeline:
Technology Maturity Curve
Different technologies within the ecosystem are at varying stages of maturity, influencing adoption timelines and investment priorities:
Innovation Trigger
- Generative AI for specialized domains
- Blockchain for supply chain verification
Peak of Inflated Expectations
- Digital twins for business processes
- Quantum-resistant cryptography
Trough of Disillusionment
- Consumer AR/VR applications
- General-purpose blockchain
Slope of Enlightenment
- AI-driven analytics
- Edge computing
Plateau of Productivity
- Cloud infrastructure
- Mobile applications
Technology Evolution Timeline
- Improved generative models
- specialized AI applications
- AI-human collaboration systems
- multimodal AI platforms
- General AI capabilities
- AI-driven scientific breakthroughs
Expert Perspectives
Leading experts in the ai tech sector provide diverse perspectives on how the landscape will evolve over the coming years:
"The next frontier is AI systems that can reason across modalities and domains with minimal human guidance."
— AI Researcher
"Organizations that develop effective AI governance frameworks will gain competitive advantage."
— Industry Analyst
"The AI talent gap remains a critical barrier to implementation for most enterprises."
— Chief AI Officer
Areas of Expert Consensus
- Acceleration of Innovation: The pace of technological evolution will continue to increase
- Practical Integration: Focus will shift from proof-of-concept to operational deployment
- Human-Technology Partnership: Most effective implementations will optimize human-machine collaboration
- Regulatory Influence: Regulatory frameworks will increasingly shape technology development
Short-Term Outlook (1-2 Years)
In the immediate future, organizations will focus on implementing and optimizing currently available technologies to address pressing ai tech challenges:
- Improved generative models
- specialized AI applications
- enhanced AI ethics frameworks
These developments will be characterized by incremental improvements to existing frameworks rather than revolutionary changes, with emphasis on practical deployment and measurable outcomes.
Mid-Term Outlook (3-5 Years)
As technologies mature and organizations adapt, more substantial transformations will emerge in how security is approached and implemented:
- AI-human collaboration systems
- multimodal AI platforms
- democratized AI development
This period will see significant changes in security architecture and operational models, with increasing automation and integration between previously siloed security functions. Organizations will shift from reactive to proactive security postures.
Long-Term Outlook (5+ Years)
Looking further ahead, more fundamental shifts will reshape how cybersecurity is conceptualized and implemented across digital ecosystems:
- General AI capabilities
- AI-driven scientific breakthroughs
- new computing paradigms
These long-term developments will likely require significant technical breakthroughs, new regulatory frameworks, and evolution in how organizations approach security as a fundamental business function rather than a technical discipline.
Key Risk Factors and Uncertainties
Several critical factors could significantly impact the trajectory of ai tech evolution:
Organizations should monitor these factors closely and develop contingency strategies to mitigate potential negative impacts on technology implementation timelines.
Alternative Future Scenarios
The evolution of technology can follow different paths depending on various factors including regulatory developments, investment trends, technological breakthroughs, and market adoption. We analyze three potential scenarios:
Optimistic Scenario
Responsible AI driving innovation while minimizing societal disruption
Key Drivers: Supportive regulatory environment, significant research breakthroughs, strong market incentives, and rapid user adoption.
Probability: 25-30%
Base Case Scenario
Incremental adoption with mixed societal impacts and ongoing ethical challenges
Key Drivers: Balanced regulatory approach, steady technological progress, and selective implementation based on clear ROI.
Probability: 50-60%
Conservative Scenario
Technical and ethical barriers creating significant implementation challenges
Key Drivers: Restrictive regulations, technical limitations, implementation challenges, and risk-averse organizational cultures.
Probability: 15-20%
Scenario Comparison Matrix
Factor | Optimistic | Base Case | Conservative |
---|---|---|---|
Implementation Timeline | Accelerated | Steady | Delayed |
Market Adoption | Widespread | Selective | Limited |
Technology Evolution | Rapid | Progressive | Incremental |
Regulatory Environment | Supportive | Balanced | Restrictive |
Business Impact | Transformative | Significant | Modest |
Transformational Impact
Redefinition of knowledge work, automation of creative processes. This evolution will necessitate significant changes in organizational structures, talent development, and strategic planning processes.
The convergence of multiple technological trends—including artificial intelligence, quantum computing, and ubiquitous connectivity—will create both unprecedented security challenges and innovative defensive capabilities.
Implementation Challenges
Ethical concerns, computing resource limitations, talent shortages. Organizations will need to develop comprehensive change management strategies to successfully navigate these transitions.
Regulatory uncertainty, particularly around emerging technologies like AI in security applications, will require flexible security architectures that can adapt to evolving compliance requirements.
Key Innovations to Watch
Multimodal learning, resource-efficient AI, transparent decision systems. Organizations should monitor these developments closely to maintain competitive advantages and effective security postures.
Strategic investments in research partnerships, technology pilots, and talent development will position forward-thinking organizations to leverage these innovations early in their development cycle.
Technical Glossary
Key technical terms and definitions to help understand the technologies discussed in this article.
Understanding the following technical concepts is essential for grasping the full implications of the security threats and defensive measures discussed in this article. These definitions provide context for both technical and non-technical readers.