Vision Transformers (ViT) Explained: Are They Better Than CNNs? - Related to they, savoir, sur, cnns?, le
MIT : tout savoir sur le Massachusetts Institute of Technology
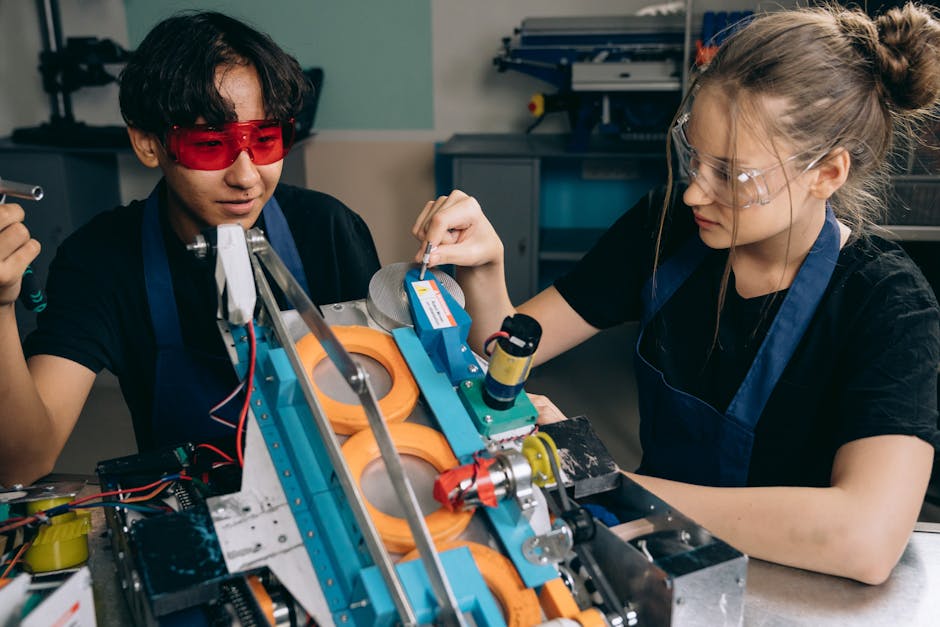
Le Massachusetts Institute of Technology (MIT) est l’une des meilleures universités informatiques et technologiques du monde. De nombreux prix Nobel sont des sortants du MIT ou y ont intégré en tant que professeurs.
Cette université américaine fondée au 19e siècle formait originairement des ingénieurs. Plus tard, le MIT est devenu un institut pluridisciplinaire, mais ce sont surtout les enseignements en science et en technologie qui lui valent aujourd’hui sa grande renommée mondiale.
Le MIT ou le Massachusetts Institute of Technology est un établissement privé américain d’enseignement supérieur mixte. Basé à Cambridge. Il est surtout célèbre pour son enseignement et ses recherches scientifiques et technologiques. Néanmoins, il s’agit d’une université pluridisciplinaire qui propose à la fois des études de premier cycle et supérieures.
Le MIT est constitué de cinq écoles universitaires dont la School of Architecture and Planning, la School of Engineering et la School of Humanities. Arts and Social Science. Il y a aussi la MIT Sloan School of Management et la School of Science. Il dispose également du Whitaker College of Health Sciences and Technology.
Par ailleurs, l’établissement possède également de nombreux laboratoires et centres de recherche. Nous pouvons notamment citer un réacteur nucléaire, un accélérateur linéaire, des souffleries et des observatoires géophysiques et astrophysiques. En outre, il dispose d’un centre de calcul, d’un centre de recherche spatiale, d’un centre de sciences cognitives, d’un centre d’études internationales et d’un laboratoire d’intelligence artificielle.
Enfin, le MIT comprend un grand nombre de bibliothèques spécialisées et de musées.
Le MIT a été fondé en 1861 par l’État du Massachusetts. Et est devenu un « land-grant college » en 1863. Son fondateur et également son premier président, William Barton Rogers, ont mis en place un établissement d’enseignement supérieur pour la formation scientifique et technique. Mais ce n’est qu’en 1865 que l’école a ouvert à Boston comptant 15 étudiants.
En 1916, l’établissement a déménagé à Cambridge dans le Massachusetts. Karl T. Compton, président de 1930 à 1948, transforme l’école en centre de recherche scientifique et technique de renommée internationale.
Au fil des années. Les centres de recherche dans différents domaines créés par le MIT se sont multipliés. Parmi eux, nous pouvons notamment citer l’informatique analogique dirigée par Vannevar Bush et l’aéronautique dirigée par Charles Stark Draper. Il a également administré le Radiation Laboratory, devenu plus tard le principal centre de recherche et de développement sur les radars.
En gros, le MIT a entretenu des liens avec des laboratoires militaires et des entreprises dans la recherche fondamentale et appliquée en sciences physiques, informatiques, aérospatiales et en ingénierie.
Parmi tous les laboratoires du campus. Celui qui mérite le plus notre attention est sûrement le MIT CSAIL ou le MIT Computer Science and Artificial Intelligence Laboratory.
Il a vu le jour en 2003 suite à la fusion de deux laboratoires historiques : le Laboratory for Computer Science (LCS) et le Artificial Intelligence Laboratory (AI Lab). Cette fusion a permis de réunir une communauté de chercheurs travaillant sur des projets pionniers dans divers domaines de l’informatique et de l’IA.
Le CSAIL est hébergé dans le Ray and Maria Stata Center et fait partie du Schwarzman College of Computing du MIT. Reflétant son rôle central dans l’innovation technologique au MIT. Le laboratoire est supervisé par le vice-président de la recherche du MIT.
L’année 1963 a été marquée par la formation d’un projet intitulé MAC (Project on Mathematics and. Computation). Il a été fondé par l’« Advanced Research Projects Agency » (ARPA) du Département de la Défense et la National Science Foundation.
Ce projet révolutionnaire visait à permettre à plusieurs utilisateurs d’accéder simultanément à des programmes depuis différents emplacements. Une innovation majeure pour l’époque. Sous la direction de Robert M. Fano et Fernando José Corbató, le MAC a rapidement acquis une renommée pour ses recherches sur les systèmes d’exploitation. L’intelligence artificielle et la théorie du calcul.
Parmi les premiers participants au projet MAC se trouvait Marvin Minsky qui dirigeait un groupe de recherche sur l’intelligence artificielle. En 1970, il fonde le MIT AI Lab pour avoir plus d’espace que ce que le projet lui offrait. Il est rejoint par certains de ses collègues tandis que les chercheurs qui n’y ont pas intégré ont formé le LCS en 1976.
40 ans après la création du Projet MAC, le LCS et le AI Lab ont fusionné pour former le MIT Computer Science and. Artificial Intelligence Laboratory (CSAIL). C’est donc le 1er juillet 2003 que le plus grand laboratoire du Massachusetts Institute of Technology, regroupant plus de 600 membres. Fut créé.
Actuellement, le MIT CSAIL est l’un des pionniers des nouvelles recherches en informatique. Il comprend plus de 60 groupes de recherche qui travaillent sur des centaines de projets. Leurs domaines d’expertise incluent l’apprentissage automatique, la robotique, les systèmes distribués, et bien plus encore. L’objectif du CSAIL est de développer des systèmes intelligents, capables d’interagir de manière plus fluide avec les humains et de résoudre des problèmes complexes, ce qui contribue à faire avancer l’industrie technologique dans des secteurs variés tels que la santé, les transports. Et la sécurité.
Vous l’aurez compris, le MIT est l’une des universités les plus prestigieuses au monde. Certes, il est réputé pour ses programmes d’enseignement en sciences physiques et en ingénierie. Néanmoins, les autres programmes de l’établissement ne sont pas moins compétitifs. Le MIT compte aujourd’hui 96 lauréats du prix Nobel, 8 médaillés Fields et 26 lauréats du prix Turing parmi ses anciens étudiants, ses professeurs et ses associés.
Pour intégrer le MIT. Le premier élément pris en compte est la moyenne générale au lycée qui doit être presque parfaite. Ensuite, il faudra passer un test ACT ou SAT. Par ailleurs, les activités extrascolaires seront examinées à la loupe afin de déterminer l’engagement et les capacités de leadership du candidat. D’autre part, des essais décrivant le parcours personnel du candidat permettront d’évaluer ses compétences rédactionnelles.
Étant donné que le dépôt de candidature se fait généralement avant le bac. Ce seront donc les notes du premier trimestre de terminale qui seront prises en compte. La moyenne générale du bac ne sera alors qu’une confirmation de l’inscription.
Le MIT requiert des notes très élevées au lycée, et bien que l’institution n’indique pas de moyenne spécifique. Les candidats doivent souvent être parmi les meilleurs de leur classe. Nous pouvons estimer une base de 16/20 avec d’excellentes notes pour les matières scientifiques. Cependant, il faut noter que le processus d’amission de l’établissement vise surtout à sélectionner les étudiants les plus performants. Cela va sans dire, un bac S avec une option Mathématiques a plus de chances d’intégrer cette université.
En plus des relevés de notes. Les scores des tests standardisés comme le SAT ou l’ACT sont cruciaux. Le MIT a suspendu l’exigence des scores SAT/ACT pour quelques cycles d’admission.
Le MIT n’exige pas que les futurs étudiants suivent des cours particuliers. En dehors de leurs études de lycée. Toutefois, si la possibilité de suivre des cours de Baccalauréat international (IB) ou d’Advanced Placement (AP) se présente, ce serait une occasion à saisir. Cependant, le Massachusetts Institute of Technology n’accepte généralement pas les crédits des AP et IB. Néanmoins, il existe certaines exceptions pour certains tests spécifiques selon chaque département du MIT, consultable sur leur site web.
En plus de la moyenne générale. Des tests d’anglais et de connaissance générale sont également exigés. Généralement c’est soit l’ACT soit le SAT, avec deux SAT spécifiques. Toutefois, pour les étudiants non anglophones, le MIT recommande de passer le TOEFL en plus des deux tests de SAT.
Au lieu de demander aux étudiants de rédiger un long essai. Le MIT propose de remplir plusieurs essais à réponse courte. Les candidats sont libres de formuler leurs réalisations de la façon qu’ils estiment le plus inspirant de manière à convaincre le bureau d’admissions.
Les postulants peuvent avoir des moyennes générales et des résultats de tests d’une valeur approximative. Autrement dit, ce sont les essais qui permettront de déterminer les véritables motivations et les valeurs de chaque étudiant.
En tant que meilleure université. Le MIT est très sélectif. Sur 21 312 postulants pour la classe de 2023, seulement 1 427 ont été admis en première année.
Les responsables des admissions utilisent une approche holistique pour évaluer les candidats. Outre les notes et les résultats aux tests, l’établissement recherche des candidats innovants et créatifs.
En gros. Un élément déterminant pour l’admission d’un candidat est son aptitude à s’aligner sur la mission de l’école. Il doit également avoir un esprit de coopération, de collaboration, d’initiative et de prise de risques. Par ailleurs, il doit avoir une pensée créative et pratique. La passion, la curiosité et l’enthousiasme à l’égard de ce qu’il fait sont des qualités requises. Et enfin, il devra améliorer le caractère de la communauté au MIT et prioriser l’équilibre.
Quant aux activités extra-scolaires. Le bureau des admissions recommande aux étudiants de participer à des activités qui les passionnent. Autrement dit, c’est la passion et non pas uniquement par la motivation d’intégrer le MIT qui doit guider le choix de ces activités. Généralement, l’établissement s’engage en faveur de l’intérêt public. Il recherche donc des leaders et des innovateurs pour améliorer la vie dans le bien de tous. Pour ainsi dire.
Avancées dans le domaine de l’intelligence artificielle au MIT.
Les recherches récentes en intelligence artificielle au MIT ont conduit à des avancées significatives dans plusieurs domaines. Cela reflète l’engagement continu de l’institut à repousser les limites de la technologie. Parmi les développements notables :
Le MIT a développé un nouveau cadre de diffusion, appelé DMD. Qui accélère de manière significative la génération d’images de haute qualité. Ce procédé réduit le temps de calcul tout en conservant ou surpassant la qualité des contenus visuels générés. Cette méthode intègre les principes des réseaux antagonistes génératifs (GAN) avec ceux des modèles de diffusion, permettant la génération de contenu visuel en une seule étape.
Contrôle des propriétés matérielles dans les images.
Un autre projet innovant, nommé Alchemist. Permet de modifier les propriétés matérielles des objets dans les images de manière plus intuitive et précise que les logiciels traditionnels comme Photoshop. Cette technologie peut transformer les propriétés visuelles des objets de manière réaliste, aidant ainsi dans des domaines variés allant du design graphique à la formation de données pour la robotique.
Amélioration de la vision périphérique dans les modèles IA.
Les chercheurs du MIT ont également développé un ensemble de données d’images qui simulent la vision périphérique. Cela améliore la capacité des modèles d’apprentissage automatique à détecter des objets dans leur périphérie visuelle. Cette recherche pourrait avoir des implications importantes pour la sécurité des véhicules autonomes et pour les interfaces utilisateur plus naturelles et intuitives.
Ces projets démontrent l’approche multidisciplinaire du MIT en matière d’intelligence artificielle, où les avancées technologiques ne servent pas seulement à améliorer les capacités des machines. Mais aussi à comprendre et à améliorer les interactions humaines avec ces technologies.
Le MIT HEALS : une solution novatrice pour la santé numérique.
Le MIT s’est engagé dans une initiative novatrice appelée MIT HEALS ou Health and Life Sciences Collaborative. Le programme vise à relever les défis contemporains de la santé à travers des solutions numériques. Ce programme de recherche se concentre sur l’intersection de la technologie et de la santé. Il s’agit précisément de réunir des chercheurs, des médecins, des ingénieurs et des experts en informatique.
L’objectif principal est de développer des outils et des applications capables d’améliorer la qualité des soins. Au-delà de cela, le programme ambitionne également d’optimiser la gestion des maladies et d’accroître l’accès aux services de santé. Parmi les projets phares, nous pouvons citer les systèmes d’intelligence artificielle pour le diagnostic précoce. Les plateformes de télémédecine qui permettent une consultation à distance sont aussi incluses. Sans oublier les applications de suivi de la santé qui encouragent un mode de vie sain.
Le MIT HEALS collabore avec des institutions médicales et des entreprises technologiques. Le but étant de chercher à transformer les connaissances scientifiques en innovations pratiques qui répondent aux besoins des patients et des professionnels de santé. Cette initiative illustre l’engagement du MIT à utiliser la recherche de pointe pour résoudre des problèmes de santé globaux. L’institut privilégie en même temps un écosystème d’innovation qui pourrait avoir des répercussions significatives sur la santé publique à l’échelle mondiale.
Le MIT est à la pointe des avancées en robotique. Il joue un rôle crucial dans la transformation de l’automatisation à travers des recherches innovantes et des applications pratiques.
Les chercheurs de l’institut développent des robots dotés d’une IA qui leur permettent de s’adapter à des environnements variés. Ce qui fait qu’ils sont aussi capables d’interagir de manière plus intuitive avec les humains. Par exemple, des projets tels que le robot « RoBoHoN » démontrent comment l’intégration de la robotique et des technologies de communication peut améliorer nos vies quotidiennes.
En outre, le MIT explore la biomimétique. Une technologie qui s’inspire des mouvements des animaux pour concevoir des robots plus agiles et efficaces. De telles avancées en robotique collaborative ouvrent des perspectives passionnantes dans les secteurs de la fabrication et des soins de santé.
En investissant dans l’éducation et des partenariats industriels. Le MIT prépare également la prochaine génération d’ingénieurs et de chercheurs à relever les défis de l’automatisation. Ainsi, grâce à ses innovations, l’établissement façonne l’avenir de la robotique. Mais également, il participe activement à la redéfinition des interactions entre l’homme et la machine.
Admission à l’université du MIT en 2025 : quelles sont les démarches à franchir ? - mars 2025.
Les candidats à l’admission au mit informatique pour l’année 2025 doivent compléter leur procédure de candidature en ligne via le site officiel de l’établissement. La soumission du dossier doit impérativement s’effectuer avant la date limite fixée pour obtenir une chance d’admission à cette prestigieuse université.
Le MIT accueille l’une des communautés estudiantines les plus talentueuses. Innovantes et connectées au monde. Cette institution attire chaque année des milliers d’étudiants internationaux qui souhaitent intégrer ses programmes de premier et de deuxième cycle. Pour accéder à cette école, les candidats doivent déposer leur dossier via le portail officiel en ligne de l’université américaine.
L’admission nécessite de répondre à plusieurs critères spécifiques établis par l’institution. Les candidats doivent notamment démontrer leurs compétences linguistiques et fournir une attestation de maîtrise de l’anglais, condition indispensable à leur intégration.
La renommée mondiale du MIT s’explique par l’excellence de son enseignement et la qualité exceptionnelle de sa recherche. Particulièrement dans les domaines scientifiques et technologiques. Cette réputation témoigne de la forte compétitivité du processus d’admission, où seuls les dossiers les plus solides obtiennent une réponse favorable.
Les futurs étudiants doivent donc préparer méticuleusement leur candidature et s’assurer de respecter toutes les exigences documentaires requises par l’université. Le processus de sélection évalue non seulement les résultats académiques mais aussi le potentiel d’innovation et la capacité d’intégration des candidats au sein de cet environnement unique.
In October last year, Walmart revealed its plans around AI, AR. And immersive commerce experiences. This led to the introduction of Wallaby, a collect...
'ZDNET Recommends': What exactly does it mean?
ZDNET's recommendations are based on many hours of testing, research. And comparison shopping. We gath...
In its latest addition to its Granite family of large language models (LLMs). IBM has unveiled Granite This new release focuses on delivering sma...
Unraveling Large Language Model Hallucinations
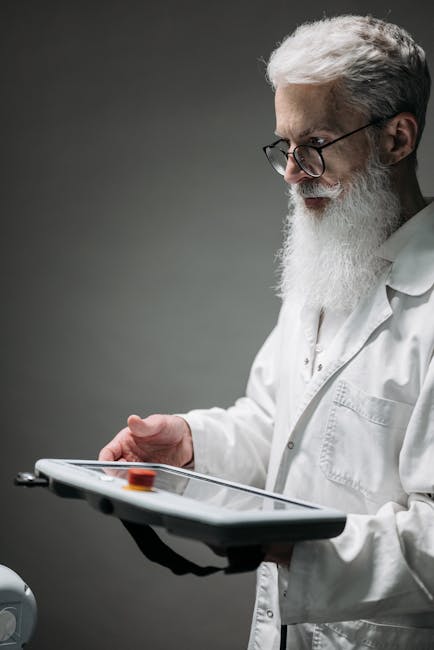
In a YouTube video titled Deep Dive into LLMs like ChatGPT, former Senior Director of AI at Tesla. Andrej Karpathy discusses the psychology of Large Language Models (LLMs) as emergent cognitive effects of the training pipeline. This article is inspired by his explanation of LLM hallucinations and the information presented in the video.
You might have seen model hallucinations. They are the instances where LLMs generate incorrect, misleading, or entirely fabricated information that appears plausible. These hallucinations happen because LLMs do not “know” facts in the way humans do; instead, they predict words based on patterns in their training data. Early models released a few years ago struggled significantly with hallucinations. Over time, mitigation strategies have improved the situation, though hallucinations haven’t been fully eliminated.
An illustrative example of LLM hallucinations (Image by Author).
Zyler Vance is a completely fictitious name I came up with. When I input the prompt “Who is Zyler Vance?” into the falcon-7b-instruct model, it generates fabricated information. Zyler Vance is not a character in The Cloverfield Paradox (2018) movie. This model, being an older version, is prone to hallucinations.
To understand where these hallucinations originate from. You have to be familiar with the training pipeline. Training LLMs typically involve three major stages.
Pretraining Post-training: Supervised Fine-Tuning (SFT) Post-training: Reinforcement Learning with Human Feedback (RLHF).
This is the initial stage of the training for LLMs. During pretraining the model is exposed to a huge quantity of very high-quality and diverse text crawled from the internet. Pretraining helps the model learn general language patterns, grammar, and facts. The output of this training phase is called the base model. It is a token simulator that predicts the next word in a sequence.
To get a sense of what the pretraining dataset might look like you can see the FineWeb dataset. FineWeb dataset is fairly representative of what you might see in an enterprise-grade language model. All the major LLM providers like OpenAI, Google, or Meta will have some equivalent dataset internally like the FineWeb dataset.
As I mentioned before. The base model is a token simulator. It simply samples internet text documents. We need to turn this base model into an assistant that can answer questions. Therefore, the pretrained model is further refined using a dataset of conversations. These conversation datasets have hundreds of thousands of conversations that are multi-term and. Very long covering a diverse breadth of topics.
Illustrative human assistant conversations from InstructGPT distribution.
These conversations come from human labelers. Given conversational context human lablers write out ideal responses for an assistant in any situation. Later, we take the base model that is trained on internet documents and substitute the dataset with the dataset of conversations. Then continue the model training on this new dataset of conversations. This way, the model adjusts rapidly and learns the statistics of how this assistant responds to queries. At the end of training the model is able to imitate human-like responses.
OpenAssistant/oasst1 is one of the open-source conversations dataset available at hugging face. This is a human-generated and human-annotated assistant-style conversation corpus consisting of 161,443 messages in 35 different languages.
Post-training: Reinforcement Learning with Human Feedback.
Supervised Fine-Tuning makes the model capable. However, even a well-trained model can generate misleading, biased, or unhelpful responses. Therefore, Reinforcement Learning with Human Feedback is required to align it with human expectations.
We start with the assistant model, trained by SFT. For a given prompt we generate multiple model outputs. Human labelers rank or score multiple model outputs based on quality, safety, and alignment with human preferences. We use these data to train a whole separate neural network that we call a reward model.
The reward model imitates human scores. It is a simulator of human preferences. It is a completely separate neural network, probably with a transformer architecture, but. It is not a language model in the sense that it generates diverse language. It’s just a scoring model.
Now the LLM is fine-tuned using reinforcement learning. Where the reward model provides feedback on the quality of the generated outputs. So instead of asking a real human, we’re asking a simulated human for their score of an output. The goal is to maximize the reward signal, which reflects human preferences.
Now that we have a clearer understanding of the training process of large language models. We can continue with our discussion on hallucinations.
Hallucinations originate from the Supervised Fine-Tuning stage of the training pipeline. The following is a specific example of three potential conversations you might have on your training set.
Examples of human-assistant conversations (Image by Author).
As I have shown earlier. This is what human-assistant conversations would look like in the training time. These conversations are created by human labelers under strict guidelines. When a labeler is writing the correct answer for the assistant in each one of these cases either they know this person or they research them on the internet. After that, they write the assistant response that has a confident tone of an answer.
At test time, if the model is asked about an individual it has not seen during training. It does not simply respond with an acknowledgment of ignorance. Simply put it does not reply with “Oh, I don’t know”. Instead, the model statistically imitates the training set.
In the training set. The questions in the form “Who is X?” are confidently answered with the correct answer. Therefore at the test time, the model replies with the style of the answer and it gives the statistically most likely guess. So it just makes stuff up that is statistically consistent with the style of the answer in its training set.
Our question now is how to mitigate the hallucinations. It is evident that our dataset should include examples where the correct answer for the assistant is that the model does not know about some particular fact. However, these answers must be produced only in instances where the model actually does not know. So the key question is how do we know what the model knows and what it does not? We need to probe the model to figure that out empirically.
The task is to figure out the boundary of the model’s knowledge. Therefore, we need to interrogate the model to figure out what it knows and doesn’t know. Then we can add examples to the training set for the things that the model doesn’t know. The correct response, in such cases, is that the model does not know them.
An example of a training instance where the model doesn’t know the answer to a particular question.
Let’s take a look at how Meta dealt with hallucinations using this concept for the Llama 3 series of models.
In their 2024 paper titled “The Llama 3 Herd of Models”. Touvron et al. describe how they have developed a knowledge-probing technique to achieve this. Their primary approach involves generating data that aligns model generations with subsets of factual data present in the pre-training data. They describe the following procedure for the data generation process:
Extract a data snippet from the pre-training data. Generate a factual question about these snippets (context) by prompting Llama 3. Sample responses from Llama 3 to the question. Score the correctness of the generations using the original context as a reference and Llama 3 as a judge. Score the informativeness of the generations using Llama 3 as a judge. Generate a refusal for responses which are consistently informative and incorrect across the generations, using Llama 3. (p. 27).
After that data generated from the knowledge probe is used to encourage the model to only answer the questions for which it knows about, and. Refrain from answering questions that it is unsure about. Implementing this technique has improved the hallucination issue over time.
We have more effective mitigation strategies than just saying we do not know. We can provide the LLM with an opportunity to generate factual responses and accurately address the question. What would you do, in a case where I ask you a factual question that you don’t have an answer to? How do you answer the question? You could do some research and search the internet to figure out the answer to the question. Then tell me the answer to the question. We can do the same thing with LLMs.
You can think of the knowledge inside the parameters of the trained neural network as a vague recollection of things that the model has seen during pretraining a long time ago. Knowledge in the model parameters is analogous to something in your memory that you read a month ago. You can remember things that you read continuously over time than something you read rarely. If you don’t have a good recollection of information that you read, what you do is go and look it up. When you look up information, you are essentially refreshing your working memory with information, allowing you to retrieve and. Discuss it.
We need some equivalent mechanism to allow the model to refresh its memory or recollection of information. We can achieve this by introducing tools for the model. The model can use web search tools instead of just replying with “I am sorry, I don’t know the answer”. To achieve this we need to introduce special tokens, such as and. Along with a protocol that defines how the model is allowed to use these tokens. In this mechanism, the language model can emit special tokens. Now in a case where the model doesn’t know the answer, it has the option to emit the special token instead of replying with “I am sorry. I don’t know the answer”. After that, the model will emit the query and .
Here when the program that is sampling from the model encounters the special token during inference. It will pause the generation process instead of sampling the next token in the sequence. It will initiate a session with the search engine, input the search query into the search engine, and. Retrieve all the extracted text from the results. Then it will insert that text inside the context window.
The extracted text from the web search is now within the context window that will be fed into the neural network. Think of the context window as the working memory of the model. The data inside the context window is directly accessible by the model. It is directly fed into the neural network. Therefore it is no longer a vague recollection of information. Now, when sampling new tokens, it can very easily reference the data that has been copy-pasted there. Thus, this is a general overview of how these web search tools function.
An example of a training instance with special tokens. The […] notation indicates the placeholder for the extracted content.
How can we teach the model to correctly use these tools like web search? Again we accomplish this through training sets. We now need enough data and numerous conversations that demonstrate, by example, how the model should use web search. We need to illustrate with examples aspects such as: “What are the settings where you are using the search? What does it look like? How do you start a search?” Because of the pretraining stage, it possesses a native understanding of what a web search is and. What constitutes a good search query. Therefore, if your training set contains several thousand examples, the model will be able to understand clearly how the tool works.
Large language model hallucinations are inherent consequences of the training pipeline. Particularly arising from the supervised fine-tuning stage. Since language models are designed to generate statistically probable text, they often produce responses that appear plausible but. Lack a factual basis.
Early models were prone to hallucinations significantly. However, the problem has improved with the implementation of various mitigation strategies. Knowledge probing techniques and training the model to use web search tools have been proven effective in mitigating the problem. Despite these improvements, completely eliminating hallucinations remains an ongoing challenge. As LLMs continue to evolve, mitigating hallucinations to a large extent is crucial to ensuring their reliability as a trustworthy knowledge base.
If you enjoyed this article, connect with me on X (formerly Twitter) for more insights.
In October last year, Walmart revealed its plans around AI. AR, and immersive commerce experiences. This led to the introduction of Wallaby, a collect...
Microsoft has expanded its Copilot AI to Mac individuals. On Thursday, the official Copilot app landed in the Mac App Store in the US, Canada, and th...
'ZDNET Recommends': What exactly does it mean?
ZDNET's recommendations are based on many hours of testing. Research, and comparison shopping. We gath...
Vision Transformers (ViT) Explained: Are They Better Than CNNs?
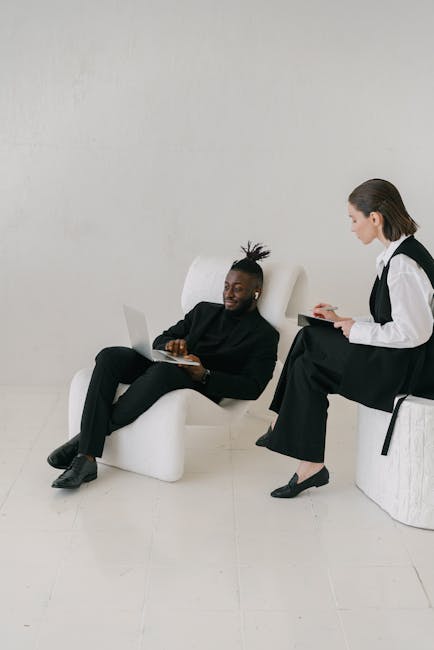
Ever since the introduction of the self-attention mechanism, Transformers have been the top choice when it comes to Natural Language Processing (NLP) tasks. Self-attention-based models are highly parallelizable and require substantially fewer parameters, making them much more computationally efficient, less prone to overfitting. And easier to fine-tune for domain-specific tasks [1]. Furthermore, the key advantage of transformers over past models (like RNN, LSTM, GRU and other neural-based architectures that dominated the NLP domain prior to the introduction of Transformers) is their ability to process input sequences of any length without losing context, through the use of the self-attention mechanism that focuses on different parts of the input sequence, and. How those parts interact with other parts of the sequence, at different times [2]. Because of these qualities, Transformers has made it possible to train language models of unprecedented size, with more than 100B parameters, paving the way for the current state-of-the-art advanced models like the Generative Pre-trained Transformer (GPT) and the Bidirectional Encoder Representations from Transformers (BERT) [1].
However, in the field of computer vision, convolutional neural networks or CNNs. Remain dominant in most, if not all, computer vision tasks. While there has been an increasing collection of research work that attempts to implement self-attention-based architectures to perform computer vision tasks. Very few has reliably outperformed CNNs with promising scalability [3]. The main challenge with integrating the transformer architecture with image-related tasks is that, by design, the self-attention mechanism, which is the core component of transformers, has a quadratic time complexity with respect to sequence length, O(n2), as shown in Table I and. As discussed further in Part This is usually not a problem for NLP tasks that use a relatively small number of tokens per input sequence (, a 1,000-word paragraph will only have 1,000 input tokens, or a few more if sub-word units are used as tokens instead of full words). However, in computer vision, the input sequence (the image) can have a token size with orders of magnitude greater than that of NLP input sequences. For example, a relatively small 300 x 300 x 3 image can easily have up to 270,000 tokens and. Require a self-attention map with up to billion parameters (270,0002) when self-attention is applied naively.
Table I. Time complexity for different layer types [2].
For this reason, most of the research work that attempt to use self-attention-based architectures to perform computer vision tasks did so either by applying self-attention locally, using transformer blocks in conjunction with CNN layers. Or by only replacing specific components of the CNN architecture while maintaining the overall structure of the network; never by only using a pure transformer [3]. The goal of Dr. Dosovitskiy, et. al. in their work, “An Image is Worth 16×16 Words: Transformers for Image Recognition at Scale”, is to show that it is indeed possible to implement image classification by applying self-attention globally through the use of the basic Transformer encoder architure, while at the same time requiring significantly less computational resources to train, and. Outperforming state-of-the-art convolutional neural networks like ResNet.
Transformers, introduced in the paper titled “Attention is All You Need” by Vaswani et al. in 2017, are a class of neural network architectures that have revolutionized various natural language processing and machine learning tasks. A high level view of its architecture is shown in Fig. 1.
Fig. 1. The Transformer model architecture showing the encoder (left block).
and decoder components (right block) [2].
Since its introduction, transformers have served as the foundation for many state-of-the-art models in NLP; including BERT, GPT. And more. Fundamentally, they are designed to process sequential data, such as text data, without the need for recurrent or convolutional layers [2]. They achieve this by relying heavily on a mechanism called self-attention.
The self-attention mechanism is a key innovation introduced in the paper that allows the model to capture relationships between different elements in a given sequence by weighing the importance of each element in the sequence with respect to other elements [2]. Say for instance, you want to translate the following sentence:
“The animal didn’t cross the street because it was too tired.”.
What does the word “it” in this particular sentence refer to? Is it referring to the street or the animal? For us humans, this may be a trivial question to answer. But for an algorithm, this can be considered a complex task to perform. However, through the self-attention mechanism, the transformer model is able to estimate the relative weight of each word with respect to all the other words in the sentence. Allowing the model to associate the word “it” with “animal” in the context of our given sentence [4].
Fig. 2. Sample output of the 5th encoder in a 5-encoder stack self-attention block given the word “it” as an input. We can see that the attention mechanism is associating our input word with the phrase “The Animal” [4].
A transformer transforms a given input sequence by passing each element through an encoder (or a stack of encoders) and a decoder (or a stack of decoders) block. In parallel [2]. Each encoder block contains a self-attention block and a feed forward neural network. Here, we only focus on the transformer encoder block as this was the component used by Dosovitskiy et al. in their Vision Transformer image classification model.
As is the case with general NLP applications, the first step in the encoding process is to turn each input word into a vector using an embedding layer which converts our text data into a vector that represents our word in the vector space while retaining its contextual information. We then compile these individual word embedding vectors into a matrix X. Where each row i represents the embedding of each element i in the input sequence. Then, we create three sets of vectors for each element in the input sequence; namely, Key (K), Query (Q), and Value (V). These sets are derived by multiplying matrix X with the corresponding trainable weight matrices WQ, WK, and WV [2].
Afterwards, we perform a matrix multiplication between K and Q, divide the result by the square-root of the dimensionality of K: …and then apply a softmax function to normalize the output and. Generate weight values between 0 and 1 [2].
We will call this intermediary output the attention factor. This factor, shown in Eq. 4, represents the weight that each element in the sequence contributes to the calculation of the attention value at the current position (word being processed). The idea behind the softmax operation is to amplify the words that the model thinks are relevant to the current position, and. Attenuate the ones that are irrelevant. For example, in Fig. 3, the input sentence “He later went to research Malaysia for one year” is passed into a BERT encoder unit to generate a heatmap that illustrates the contextual relationship of each word with each other. We can see that words that are deemed contextually associated produce higher weight values in their respective cells, visualized in a dark pink color. While words that are contextually unrelated have low weight values, represented in pale pink.
Fig. 3. Attention matrix visualization – weights in a BERT Encoding Unit [5].
Finally, we multiply the attention factor matrix to the value matrix V to compute the aggregated self-attention value matrix Z of this layer [2]. Where each row i in Z represents the attention vector for word i in our input sequence. This aggregated value essentially bakes the “context” provided by other words in the sentence into the current word being processed. The attention equation shown in Eq. 5 is sometimes also referred to as the Scaled Dot-Product Attention.
The Multi-Headed Self-Attention.
In the paper by Vaswani et. al., the self-attention block is further augmented with a mechanism known as the “multi-headed” self-attention, shown in Fig 4. The idea behind this is instead of relying on a single attention mechanism, the model employs multiple parallel attention “heads” (in the paper, Vaswani et. al. used 8 parallel attention layers), wherein each of these attention heads learns different relationships and provides unique perspectives on the input sequence [2]. This improves the performance of the attention layer in two key ways:
First. It expands the ability of the model to focus on different positions within the sequence. Depending on multiple variations involved in the initialization and training process, the calculated attention value for a given word (Eq. 5) can be dominated by other certain unrelated words or phrases or even by the word itself [4]. By computing multiple attention heads, the transformer model has multiple opportunities to capture the correct contextual relationships, thus becoming more robust to variations and ambiguities in the , since each of our Q. K, V matrices are randomly initialized independently across all the attention heads, the training process then yields several Z matrices (Eq. 5), which gives the transformer multiple representation subspaces [4]. For example, one head might focus on syntactic relationships while another might attend to semantic meanings. Through this, the model is able to capture more diverse relationships within the data.
Fig. 4. Illustrating the Multi-Headed Self-Attention Mechanism. Each individual attention head yields a scaled dot-product attention value, which are concatenated and multiplied to a learned matrix WO to generate the aggregated multi-headed self-attention value matrix [4].
The fundamental innovation behind the Vision Transformer (ViT) revolves around the idea that images can be processed as sequences of tokens rather than grids of pixels. In traditional CNNs, input images are analyzed as overlapping tiles via a sliding convolutional filter, which are then processed hierarchically through a series of convolutional and. Pooling layers. In contrast, ViT treats the image as a collection of non-overlapping patches. Which are treated as the input sequence to a standard Transformer encoder unit.
Fig. 5. The Vision Transformer architecture (left), and the Transfomer encoder unit.
By defining the input tokens to the transformer as non-overlapping image patches rather than individual pixels, we are therefore able to reduce the dimension of the attention map from ⟮𝐻 𝓍 𝑊⟯2 to ⟮𝑛 𝑝ℎ 𝓍 𝑛 𝑝𝑤 ⟯2 given 𝑛 𝑝ℎ ≪𝐻 and 𝑛 𝑝𝑤 ≪ 𝑊; where 𝐻 and 𝑊 are the height and width of the image. And 𝑛 𝑝ℎ and 𝑛 𝑝 𝑙 are the number of patches in the corresponding axes. By doing so, the model is able to handle images of varying sizes without requiring extensive architectural changes [3].
These image patches are then linearly embedded into lower-dimensional vectors, similar to the word embedding step that produces matrix X in Part Since transformers do not contain recurrence nor convolutions. They lack the capacity to encode positional information of the input tokens and are therefore permutation invariant [2]. Hence, as it is done in NLP applications, a positional embedding is appended to each linearly encoded vector prior to input into the transformer model, in order to encode the spatial information of the patches. Ensuring that the model understands the position of each token relative to other tokens within the image. Additionally, an extra learnable classifier cls embedding is added to the input. All of these (the linear embeddings of each 16 x 16 patch, the extra learnable classifier embedding, and. Their corresponding positional embedding vectors) are passed through a standard Transformer encoder unit as discussed in Part 2. The output corresponding to the added learnable cls embedding is then used to perform classification via a standard MLP classifer head [3].
In the paper, the two largest models, ViT-H/14 and ViT-L/16, both pre-trained on the JFT-300M dataset, are compared to state-of-the-art CNNs—as shown in Table II, including Big Transfer (BiT). Which employs supervised transfer learning with large ResNets, and Noisy Student, a large EfficientNet trained using semi-supervised learning on ImageNet and JFT-300M without labels [3]. At the time of this study’s publication, Noisy Student held the state-of-the-art position on ImageNet. While BiT-L on the other datasets utilized in the paper [3]. All models were trained in TPUv3 hardware, and the number of TPUv3-core-days that it took to train each model were recorded.
Table II. Comparison of model performance against popular image classification benchmarks. Reported here are the mean and standard deviation of the accuracies, averaged over three fine-tuning runs [3].
We can see from the table that Vision Transformer models pre-trained on the JFT-300M dataset outperforms ResNet-based baseline models on all datasets; while, at the same time. Requiring significantly less computational resources (TPUv3-core-days) to pre-train. A secondary ViT-L/16 model was also trained on a much smaller public ImageNet-21k dataset, and. Is shown to also perform relatively well while requiring up to 97% less computational resources compared to state-of-the-art counter parts [3].
Fig. 6 demonstrates the comparison of the performance between the BiT and. ViT models (measured using the ImageNet Top1 Accuracy metric) across different pre-training datasets of varying sizes. We see that the ViT-Large models underperform compared to the base models on the small datasets like ImageNet, and roughly equivalent performance on ImageNet-21k. However, when pre-trained on larger datasets like JFT-300M, the ViT clearly outperforms the base model [3].
Fig. 6. BiT (ResNet) vs ViT on different pre-training datasets [3].
Further exploring how the size of the dataset relates to model performance, the authors trained the models on various random subsets of the JFT dataset—9M, 30M. 90M, and the full JFT-300M. Additional regularization was not added on smaller subsets in order to assess the intrinsic model properties (and not the effect of regularization) [3]. Fig. 7 presents that ViT models overfit more than ResNets on smaller datasets. Data presents that ResNets perform superior with smaller pre-training datasets but plateau sooner than ViT; which then outperforms the former with larger pre-training. The authors conclude that on smaller datasets, convolutional inductive biases play a key role in CNN model performance, which ViT models lack. However, with large enough data, learning relevant patterns directly outweighs inductive biases, wherein ViT excels [3].
Fig. 7. ResNet vs ViT on different subsets of the JFT training dataset [3].
Finally, the authors analyzed the models’ transfer performance from JFT-300M vs total pre-training compute resources allocated. Across different architectures, as shown in Fig. 8. Here, we see that Vision Transformers outperform ResNets with the same computational budget across the board. ViT uses approximately 2-4 times less compute to attain similar performance as ResNet [3]. Implementing a hybrid model does improve performance on smaller model sizes, but the discrepancy vanishes for larger models. Which the authors find surprising as the initial hypothesis is that the convolutional local feature processing should be able to assist ViT regardless of compute size [3].
Fig. 8. Performance of the models across different pre-training compute values—exa floating point operations per second (or exaFLOPs) [3].
What does the ViT model learn?
Additionally, in order to understand how ViT processes image data. It is essential to analyze its internal representations. In Part 3, we saw that the input patches generated from the image are fed into a linear embedding layer that projects the 16×16 patch into a lower dimensional vector space, and. Its resulting embedded representations are then appended with positional embeddings. Fig. 9 presents that the model indeed learns to encode the relative position of each patch in the image. The authors used cosine similarity between the learned positional embeddings across patches [3]. High cosine similarity values emerge on similar relative area within the position embedding matrix corresponding to the patch; , the top right patch (row 1. Col 7) has a corresponding high cosine similarity value (yellow pixels) on the top-right area of the position embedding matrix [3].
Fig. 9. Learned positional embedding for the input image patches [3].
Meanwhile, Fig. 10 (left) displays the top principal components of learned embedding filters that are applied to the raw image patches prior to the addition of the positional embeddings. What’s interesting for me is how similar this is to the learned hidden layer representations that you get from Convolutional neural networks. An example of which is shown in the same figure (right) using the AlexNet architecture.
Fig. 10. Filters of the initial linear embedding layer of ViT-L/32 (left) [3].
The first layer of filters from AlexNet (right) [6].
By design, the self-attention mechanism should allow ViT to integrate information across the entire image. Even at the lowest layer, effectively giving ViTs a global receptive field at the start. We can somehow see this effect in Fig. 10 where the learned embedding filters captured lower level elements like lines and grids, as well as higher level patterns combining lines and color blobs. This in contrast with CNNs whose receptive field size at the lowest layer is very small (because local application of the convolution operation only attends to the area defined by the filter size), and. Only widens towards the deeper convolutions as further applications of convolutions extract context from the combined information extracted from lower layers. The authors further tested this by measuring the attention distance which is computed from the “average distance in the image space across which information is integrated based on the attention weights [3].” The results are shown in Fig. 11.
Fig. 11. Size of attended area by head and network depth [3].
From the figure, we can see that even at very low layers of the network, some heads attend to most of the image already (as indicated by data points with high mean attention distance value at lower values of network depth); thus proving the ability of the ViT model to integrate image information globally, even at the lowest layers.
Finally. The authors also calculated the attention maps from the output token to the input space using Attention Rollout by averaging the attention weights of the ViT-L/16 across all heads and then recursively multiplying the weight matrices of all layers. This results in a nice visualization of what the output layer attends to prior to classification, shown in Fig. 12 [3].
Fig. 12. Representative examples of attention from the output token to the input space [3].
5. So, is ViT the future of Computer Vision?
The Vision Transformer (ViT) introduced by Dosovitskiy et. al. in the research study showcased in this paper is a groundbreaking architecture for computer vision tasks. Unlike previous methods that introduce image-specific biases, ViT treats an image as a sequence of patches and process it using a standard Transformer encoder. Such as how Transformers are used in NLP. This straightforward yet scalable strategy, combined with pre-training on extensive datasets, has yielded impressive results as discussed in Part 4. The Vision Transformer (ViT) either matches or surpasses the state-of-the-art on numerous image classification datasets (Fig. 6, 7, and 8), all while maintaining cost-effectiveness in pre-training [3].
However, like in any technology, it has its limitations. First, in order to perform well, ViTs require a very large amount of training data that not everyone has access to in the required scale. Especially when compared to traditional CNNs. The authors of the paper used the JFT-300M dataset, which is a limited-access dataset managed by Google [7]. The dominant approach to get around this is to use the model pre-trained on the large dataset, and then fine-tune it to smaller (downstream) tasks. However, second, there are still very few pre-trained ViT models available as compared to the available pre-trained CNN models, which limits the availability of transfer learning benefits for these smaller. Much more specific computer vision tasks. Third, by design, ViTs process images as sequences of tokens (discussed in Part 3), which means they do not naturally capture spatial information [3]. While adding positional embeddings do help remedy this lack of spatial context, ViTs may not perform as well as CNNs in image localization tasks, given CNNs convolutional layers that are excellent at capturing these spatial relationships.
Moving forward, the authors mention the need to further study scaling ViTs for other computer vision tasks such as image detection and. Segmentation, as well as other training methods like self-supervised pre-training [3]. Future research may focus on making ViTs more efficient and scalable, such as developing smaller and. More lightweight ViT architectures that can still deliver the same competitive performance. Furthermore, providing advanced accessibility by creating and sharing a wider range of pre-trained ViT models for various tasks and. Domains can further facilitate the development of this technology in the future.
N. Pogeant, “Transformers - the NLP revolution,” Medium, (accessed Sep. 23, 2023). A. Vaswani, et. al. “Attention is all you need.” NIPS 2017. A. Dosovitskiy, et. al. “An Image is Worth 16×16 Words: Transformers for Image Recognition at Scale,” ICLR 2021. X. Wang, G. Chen, G. Qian, P. Gao, X.-Y. Wei, Y. Wang, Y. Tian, and W. Gao, “Large-scale multi-modal pre-trained models: A comprehensive survey,” Machine Intelligence Research, vol. 20, no. 4, pp. 447–482, 2023, doi: H. Wang, “Addressing Syntax-Based Semantic Complementation: Incorporating Entity and Soft Dependency Constraints into Metonymy Resolution”, Scientific Figure on ResearchGate. Available from: [accessed 24 Sep, 2023] A. Krizhevsky, et. al. “ImageNet Classification with Deep Convolutional Neural Networks,” NIPS 2012. C. Sun, et. al. “Revisiting Unreasonable Effectiveness of Data in Deep Learning Era,” Google Research, ICCV 2017.
* ChatGPT. Used sparingly to rephrase certain paragraphs for more effective grammar and more concise explanations. All ideas in the findings belong to me unless otherwise indicated. Chat Reference: .
Un jeune Chinois, connu sous le nom de famille Liu, a été victime d’une fraude. Il pensait être en couple avec une femme nommée Mme Jiao. Liu a envoyé...
L’essor de l’intelligence artificielle est palpable dans le Doubs, où une nouvelle génération d’agences IA se développe pour répondre aux demandes cro...
Smartphone sales will grow in fits and starts. While tablet demand will wane. Large language models (LLMs) will boom, and de...
Market Impact Analysis
Market Growth Trend
2018 | 2019 | 2020 | 2021 | 2022 | 2023 | 2024 |
---|---|---|---|---|---|---|
23.1% | 27.8% | 29.2% | 32.4% | 34.2% | 35.2% | 35.6% |
Quarterly Growth Rate
Q1 2024 | Q2 2024 | Q3 2024 | Q4 2024 |
---|---|---|---|
32.5% | 34.8% | 36.2% | 35.6% |
Market Segments and Growth Drivers
Segment | Market Share | Growth Rate |
---|---|---|
Machine Learning | 29% | 38.4% |
Computer Vision | 18% | 35.7% |
Natural Language Processing | 24% | 41.5% |
Robotics | 15% | 22.3% |
Other AI Technologies | 14% | 31.8% |
Technology Maturity Curve
Different technologies within the ecosystem are at varying stages of maturity:
Competitive Landscape Analysis
Company | Market Share |
---|---|
Google AI | 18.3% |
Microsoft AI | 15.7% |
IBM Watson | 11.2% |
Amazon AI | 9.8% |
OpenAI | 8.4% |
Future Outlook and Predictions
The Tout Savoir Massachusetts landscape is evolving rapidly, driven by technological advancements, changing threat vectors, and shifting business requirements. Based on current trends and expert analyses, we can anticipate several significant developments across different time horizons:
Year-by-Year Technology Evolution
Based on current trajectory and expert analyses, we can project the following development timeline:
Technology Maturity Curve
Different technologies within the ecosystem are at varying stages of maturity, influencing adoption timelines and investment priorities:
Innovation Trigger
- Generative AI for specialized domains
- Blockchain for supply chain verification
Peak of Inflated Expectations
- Digital twins for business processes
- Quantum-resistant cryptography
Trough of Disillusionment
- Consumer AR/VR applications
- General-purpose blockchain
Slope of Enlightenment
- AI-driven analytics
- Edge computing
Plateau of Productivity
- Cloud infrastructure
- Mobile applications
Technology Evolution Timeline
- Improved generative models
- specialized AI applications
- AI-human collaboration systems
- multimodal AI platforms
- General AI capabilities
- AI-driven scientific breakthroughs
Expert Perspectives
Leading experts in the ai tech sector provide diverse perspectives on how the landscape will evolve over the coming years:
"The next frontier is AI systems that can reason across modalities and domains with minimal human guidance."
— AI Researcher
"Organizations that develop effective AI governance frameworks will gain competitive advantage."
— Industry Analyst
"The AI talent gap remains a critical barrier to implementation for most enterprises."
— Chief AI Officer
Areas of Expert Consensus
- Acceleration of Innovation: The pace of technological evolution will continue to increase
- Practical Integration: Focus will shift from proof-of-concept to operational deployment
- Human-Technology Partnership: Most effective implementations will optimize human-machine collaboration
- Regulatory Influence: Regulatory frameworks will increasingly shape technology development
Short-Term Outlook (1-2 Years)
In the immediate future, organizations will focus on implementing and optimizing currently available technologies to address pressing ai tech challenges:
- Improved generative models
- specialized AI applications
- enhanced AI ethics frameworks
These developments will be characterized by incremental improvements to existing frameworks rather than revolutionary changes, with emphasis on practical deployment and measurable outcomes.
Mid-Term Outlook (3-5 Years)
As technologies mature and organizations adapt, more substantial transformations will emerge in how security is approached and implemented:
- AI-human collaboration systems
- multimodal AI platforms
- democratized AI development
This period will see significant changes in security architecture and operational models, with increasing automation and integration between previously siloed security functions. Organizations will shift from reactive to proactive security postures.
Long-Term Outlook (5+ Years)
Looking further ahead, more fundamental shifts will reshape how cybersecurity is conceptualized and implemented across digital ecosystems:
- General AI capabilities
- AI-driven scientific breakthroughs
- new computing paradigms
These long-term developments will likely require significant technical breakthroughs, new regulatory frameworks, and evolution in how organizations approach security as a fundamental business function rather than a technical discipline.
Key Risk Factors and Uncertainties
Several critical factors could significantly impact the trajectory of ai tech evolution:
Organizations should monitor these factors closely and develop contingency strategies to mitigate potential negative impacts on technology implementation timelines.
Alternative Future Scenarios
The evolution of technology can follow different paths depending on various factors including regulatory developments, investment trends, technological breakthroughs, and market adoption. We analyze three potential scenarios:
Optimistic Scenario
Responsible AI driving innovation while minimizing societal disruption
Key Drivers: Supportive regulatory environment, significant research breakthroughs, strong market incentives, and rapid user adoption.
Probability: 25-30%
Base Case Scenario
Incremental adoption with mixed societal impacts and ongoing ethical challenges
Key Drivers: Balanced regulatory approach, steady technological progress, and selective implementation based on clear ROI.
Probability: 50-60%
Conservative Scenario
Technical and ethical barriers creating significant implementation challenges
Key Drivers: Restrictive regulations, technical limitations, implementation challenges, and risk-averse organizational cultures.
Probability: 15-20%
Scenario Comparison Matrix
Factor | Optimistic | Base Case | Conservative |
---|---|---|---|
Implementation Timeline | Accelerated | Steady | Delayed |
Market Adoption | Widespread | Selective | Limited |
Technology Evolution | Rapid | Progressive | Incremental |
Regulatory Environment | Supportive | Balanced | Restrictive |
Business Impact | Transformative | Significant | Modest |
Transformational Impact
Redefinition of knowledge work, automation of creative processes. This evolution will necessitate significant changes in organizational structures, talent development, and strategic planning processes.
The convergence of multiple technological trends—including artificial intelligence, quantum computing, and ubiquitous connectivity—will create both unprecedented security challenges and innovative defensive capabilities.
Implementation Challenges
Ethical concerns, computing resource limitations, talent shortages. Organizations will need to develop comprehensive change management strategies to successfully navigate these transitions.
Regulatory uncertainty, particularly around emerging technologies like AI in security applications, will require flexible security architectures that can adapt to evolving compliance requirements.
Key Innovations to Watch
Multimodal learning, resource-efficient AI, transparent decision systems. Organizations should monitor these developments closely to maintain competitive advantages and effective security postures.
Strategic investments in research partnerships, technology pilots, and talent development will position forward-thinking organizations to leverage these innovations early in their development cycle.
Technical Glossary
Key technical terms and definitions to help understand the technologies discussed in this article.
Understanding the following technical concepts is essential for grasping the full implications of the security threats and defensive measures discussed in this article. These definitions provide context for both technical and non-technical readers.