Industry observers say GPT-4.5 is an “odd” model, question its price - Related to hallucinations,, price, is, unless, say
I Won’t Change Unless You Do
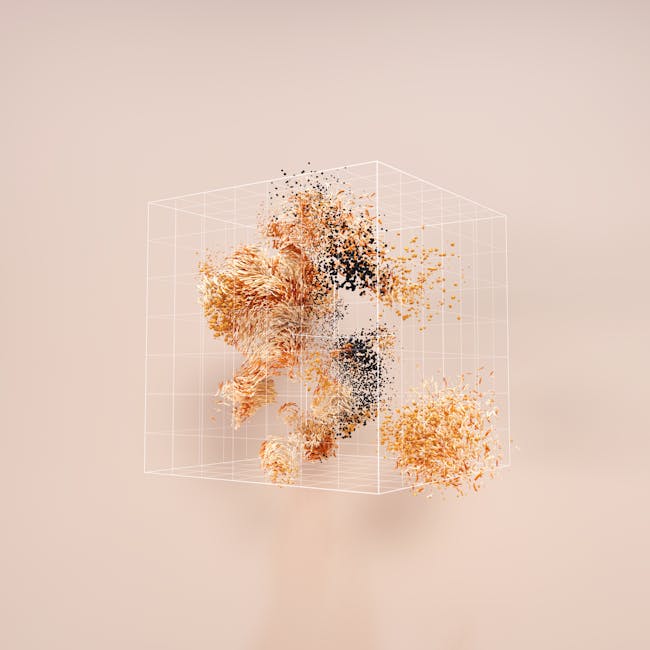
In Game Theory, how can players ever come to an end if there still might be a enhanced option to decide for? Maybe one player still wants to change their decision. But if they do, maybe the other player wants to change too. How can they ever hope to escape from this vicious circle? To solve this problem, the concept of a Nash equilibrium, which I will explain in this article. Is fundamental to game theory.
This article is the second part of a four-chapter series on game theory. If you haven’t checked out the first chapter yet, I’d encourage you to do that to get familiar with the main terms and. Concepts of game theory. If you did so, you are prepared for the next steps of our journey through game theory. Let’s go!
Finding a solution to a game in game theory can be tricky sometimes. Photo by Mel Poole on Unsplash.
We will now try to find a solution for a game in game theory. A solution is a set of actions, where each player maximizes their utility and therefore behaves rationally. That does not necessarily mean, that each player wins the game, but that they do the best they can do. Given that they don’t know what the other players will do. Let’s consider the following game:
If you are unfamiliar with this matrix-notation, you might want to take a look back at Chapter 1 and. Refresh your memory. Do you remember that this matrix gives you the reward for each player given a specific pair of actions? For example, if player 1 chooses action Y and player 2 chooses action B, player 1 will get a reward of 1 and player 2 will get a reward of 3.
Okay. What actions should the players decide for now? Player 1 does not know what player 2 will do, but. They can still try to find out what would be the best action depending on player 2’s choice. If we compare the utilities of actions Y and Z (indicated by the blue and red boxes in the next figure), we notice something interesting: If player 2 chooses action A (first column of the matrix), player 1 will get a reward of 3, if they choose action Y and. A reward of 2, if they choose action Z, so action Y is advanced in that case. But what happens, if player 2 decides for action B (second column)? In that case, action Y gives a reward of 1 and action Z gives a reward of 0, so Y is advanced than Z again. And if player 2 chooses action C (third column), Y is still advanced than Z (reward of 2 vs. reward of 1). That means, that player 1 should never use action Z, because action Y is always advanced.
We compare the rewards for player 1for actions Y and Z.
With the aforementioned considerations, player 2 can anticipate. That player 1 would never use action Z and hence player 2 doesn’t have to care about the rewards that belong to action Z. This makes the game much smaller, because now there are only two options left for player 1, and this also helps player 2 decide for their action.
We found out, that for player 1 Y is always superior than Z, so we don’t consider Z anymore.
If we look at the truncated game. We see, that for player 2, option B is always superior than action A. If player 1 chooses X, action B (with a reward of 2) is superior than option A (with a reward of 1), and. The same applies if player 1 chooses action Y. Note that this would not be the case if action Z was still in the game. However, we already saw that action Z will never be played by player 1 anyway.
We compare the rewards for player 2 for actions A and B.
As a consequence. Player 2 would never use action A. Now if player 1 anticipates that player 2 never uses action A, the game becomes smaller again and fewer options have to be considered.
We saw, that for player 2 action B is always superior than action A, so we don’t have to consider A anymore.
We can easily continue in a likewise fashion and. See that for player 1, X is now always better than Y (2>1 and 4>2). Finally, if player 1 chooses action A, player 2 will choose action B, which is better than C (2>0). In the end, only the action X (for player 1) and B (for player 2) are left. That is the solution of our game:
In the end, only one option remains, namely player 1 using X and player 2 using B.
It would be rational for player 1 to choose action X and. For player 2 to choose action B. Note that we came to that conclusion without exactly knowing what the other player would do. We just anticipated that some actions would never be taken, because they are always worse than other actions. Such actions are called strictly dominated. For example, action Z is strictly dominated by action Y, because Y is always superior than Z.
Scrabble is one of those games. Where searching for the best answer can take ages. Photo by Freysteinn G. Jonsson on Unsplash.
Such strictly dominated actions do not always exist, but. There is a similar concept that is of importance for us and is called a best answer. Say we know which action the other player chooses. In that case, deciding on an action becomes very easy: We just take the action that has the highest reward. If player 1 knew that player 2 chose option A, the best answer for player 1 would be Y. Because Y has the highest reward in that column. Do you see how we always searched for the best answers before? For each possible action of the other player we searched for the best answer, if the other player chose that action. More formally, player i’s best answer to a given set of actions of all other players is the action of player 1 which maximises the utility given the other players’ actions. Also be aware, that a strictly dominated action can never be a best answer.
Let us come back to a game we introduced in the first chapter: The prisoners’ dilemma. What are the best answers here?
How should player 1 decide, if player 2 confesses or denies? If player 2 confesses, player 1 should confess as well, because a reward of -3 is improved than a reward of -6. And what happens, if player 2 denies? In that case, confessing is improved again, because it would give a reward of 0, which is improved than a reward of -1 for denying. That means, for player 1 confessing is the best answer for both actions of player 2. Player 1 doesn’t have to worry about the other player’s actions at all but should always confess. Because of the game’s symmetry, the same applies to player 2. For them, confessing is also the best answer, no matter what player 1 does.
The Nash equilibrium is somewhat like the master key that allows us to solve game-theoretic problems. Researchers were very happy when they found it. Photo by NFT gallery on Unsplash.
If all players play their best answer. We have reached a solution of the game that is called a Nash Equilibrium. This is a key concept in game theory, because of an significant property: In a Nash Equilibrium, no player has any reason to change their action. Unless any other player does. That means all players are as happy as they can be in the situation and they wouldn’t change, even if they could. Consider the prisoner’s dilemma from above: The Nash equilibrium is reached when both confess. In this case, no player would change his action without the other. They could become enhanced if both changed their action and decided to deny, but since they can’t communicate, they don’t expect any change from the other player and. So they don’t change themselves either.
You may wonder if there is always a single Nash equilibrium for each game. Let me tell you there can also be multiple ones, as in the Bach vs. Stravinsky game that we already got to know in Chapter 1:
This game has two Nash equilibria: (Bach, Bach) and (Stravinsky, Stravinsky). In both scenarios, you can easily imagine that there is no reason for any player to change their action in isolation. If you sit in the Bach concerto with your friend, you would not leave your seat to go to the Stravinsky concerto alone. Even if you favour Stravinsky over Bach. In a likewise fashion, the Bach fan wouldn’t go away from the Stravinsky concerto if that meant leaving his friend alone. In the remaining two scenarios, you would think differently though: If you were in the Stravinsky concerto alone, you would want to get out there and. Join your friend in the Bach concerto. That is, you would change your action even if the other player doesn’t change theirs. This tells you, that the scenario you have been in was not a Nash equilibrium.
However. There can also be games that have no Nash equilibrium at all. Imagine you are a soccer keeper during a penalty shot. For simplicity, we assume you can jump to the left or to the right. The soccer player of the opposing team can also shoot in the left or right corner, and we assume. That you catch the ball if you decide for the same corner as they do and that you don’t catch it if you decide for opposing corners. We can display this game as follows:
You won’t find any Nash equilibrium here. Each scenario has a clear winner (reward 1) and a clear loser (reward -1), and hence one of the players will always want to change. If you jump to the right and catch the ball, your opponent will wish to change to the left corner. But then you again will want to change your decision, which will make your opponent choose the other corner again and so on.
We learned about finding a point of balance. Where nobody wants to change anymore. That is a Nash equilibrium. Photo by Eran Menashri on Unsplash.
In relation to this, this chapter showed how to find solutions for games by using the concept of a Nash equilibrium. Let us summarize, what we have learned so far:
A solution of a game in game theory maximizes every player’s utility or reward.
An action is called strictly dominated if there is another action that is always enhanced. In this case, it would be irrational to ever play the strictly dominated action.
if there is another action that is always enhanced. In this case, it would be irrational to ever play the strictly dominated action. The action that yields the highest reward given the actions taken by the other players is called a best answer .
. A Nash equilibrium is a state where every player plays their best answer.
is a state where every player plays their best answer. In a Nash Equilibrium, no player wants to change their action unless any other play does. In that sense, Nash equilibria are optimal states.
Some games have multiple Nash equilibria and some games have none.
If you were saddened by the fact that there is no Nash equilibrium in some games. Don’t despair! In the next chapter, we will introduce probabilities of actions and this will allow us to find more equilibria. Stay tuned!
The topics introduced here are typically covered in standard textbooks on game theory. I mainly used this one, which is written in German though:
Bartholomae, F., & Wiens, M. (2016). Spieltheorie. Ein anwendungsorientiertes Lehrbuch. Wiesbaden: Springer Fachmedien Wiesbaden.
An alternative in English language could be this one:
Espinola-Arredondo, A., & Muñoz-Garcia, F. (2023). Game Theory: An Introduction with Step-by-step Examples. Springer Nature.
Game theory is a rather young field of research, with the first main textbook being this one:
Von Neumann, J., & Morgenstern, O. (1944). Theory of games and economic behavior.
Like this article? Follow me to be notified of my future posts.
L’essor de l’intelligence artificielle est palpable dans le Doubs, où une nouvelle génération d’agences IA se développe pour répondre aux demandes cro...
En quelques jours, un homme a perdu son emploi, vu ses informations personnelles exposées et ses finances ravagées. Tout ça à cause d’un fichier appar...
Apple held its annual iPhone event back in September 2024 and debuted the iPhone 16 series. Much of the presenta...
Industry observers say GPT-4.5 is an “odd” model, question its price
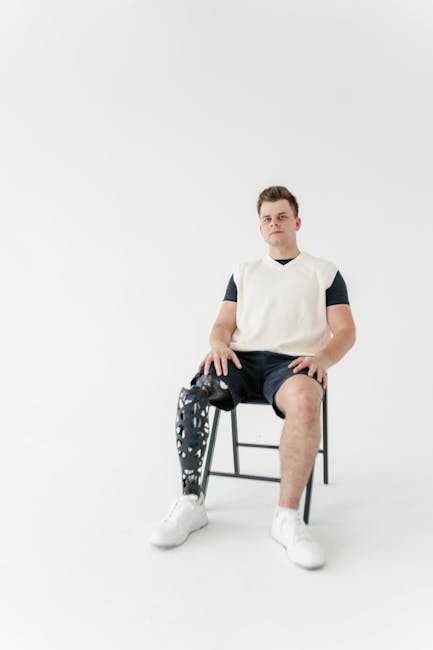
OpenAI has unveiled the release of , which CEO Sam Altman previously stated would be the last non-chain-of-thought (CoT) model.
The firm expressed the new model “is not a frontier model” but is still its biggest large language model (LLM). With more computational efficiency. Altman expressed that, even though does not reason the same way as OpenAI’s other new offerings o1 or o3-mini, this new model still offers more human-like thoughtfulness.
Industry observers, many of whom had early access to the new model, have found to be an interesting move from OpenAI, tempering their expectations of what the model should be able to achieve.
Wharton professor and AI commentator Ethan Mollick is a “very odd and. Interesting model,” noting it can get “oddly lazy on complex projects” despite being a strong writer.
Been using for a few days and it is a very odd and interesting model. It can write beautifully, is very creative, and is occasionally oddly lazy on complex like Claude while Claude feels like — Ethan Mollick ( .
OpenAI co-founder and former Tesla AI head Andrej Karpathy noted that made him remember when GPT-4 came out and. He saw the model’s potential. In a post to X, Karpathy presented that, while using GPT , “everything is a little bit more effective, and it’s awesome, but also not exactly in ways that are trivial to point to.”.
Karpathy, however warned that people shouldn’t expect revolutionary impact from the model as it “does not push forward model capability in cases where reasoning is critical (math. Code, etc.).”.
Here’s what Karpathy had to say about the latest GPT iteration in a lengthy post on X:
“Today marks the release of by OpenAI. I’ve been looking forward to this for ~2 years, ever since GPT4 was released, because this release offers a qualitative measurement of the slope of improvement you get out of scaling pretraining compute ( simply training a bigger model). Each in the version is roughly 10X pretraining compute. Now, recall that GPT1 barely generates coherent text. GPT2 was a confused toy. was “skipped” straight into GPT3, which was even more interesting. crossed the threshold where it was enough to actually ship as a product and sparked OpenAI’s “ChatGPT moment”. And GPT4 in turn also felt improved, but I’ll say that it definitely felt subtle.
I remember being a part of a hackathon trying to find concrete prompts where GPT4 outperformed They definitely existed, but. Clear and concrete “slam dunk” examples were difficult to find. It’s that … everything was just a little bit more effective but in a diffuse way. The word choice was a bit more creative. Understanding of nuance in the prompt was improved. Analogies made a bit more sense. The model was a little bit funnier. World knowledge and understanding was improved at the edges of rare domains. Hallucinations were a bit less frequent. The vibes were just a bit more effective. It felt like the water that rises all boats, where everything gets slightly improved by 20%. So it is with that expectation that I went into testing , which I had access to for a few days. And which saw 10X more pretraining compute than GPT4. And I feel like, once again, I’m in the same hackathon 2 years ago. Everything is a little bit more effective and it’s awesome, but also not exactly in ways that are trivial to point to. Still, it is incredible interesting and exciting as another qualitative measurement of a certain slope of capability that comes “for free” from just pretraining a bigger model.
Keep in mind that that was only trained with pretraining, supervised finetuning and. RLHF, so this is not yet a reasoning model. Therefore, this model release does not push forward model capability in cases where reasoning is critical (math, code, etc.). In these cases, training with RL and gaining thinking is incredibly key and works enhanced. Even if it is on top of an older base model ( GPT4ish capability or so). The state of the art here remains the full o1. Presumably, OpenAI will now be looking to further train with reinforcement learning on top of to allow it to think and. Push model capability in these domains.
HOWEVER. We do actually expect to see an improvement in tasks that are not reasoning heavy, and I would say those are tasks that are more EQ (as opposed to IQ) related and bottlenecked by world knowledge, creativity. Analogy making, general understanding, humor, etc. So these are the tasks that I was most interested in during my vibe checks.
So below, I thought it would be fun to highlight 5 funny/amusing prompts that test these capabilities, and to organize them into an interactive “LM Arena Lite” right here on X. Using a combination of images and polls in a thread. Sadly X does not allow you to include both an image and a poll in a single post, so I have to alternate posts that give the image (showing the prompt, and two responses one from 4 and one from . And the poll, where people can vote which one is more effective. After 8 hours, I’ll reveal the identities of which model is which. Let’s see what happens :)“.
Other early clients also saw potential in Box CEO Aaron Levie noted on X that his organization used to help extract structured data and. Metadata from complex enterprise content.
“The AI breakthroughs just keep coming. OpenAI just unveiled , and we’ll be making it available to Box clients later today in the Box AI Studio.
We’ve been testing in early access mode with Box AI for advanced enterprise unstructured data use-cases, and. Have seen strong results. With the Box AI enterprise eval, we test models against a variety of different scenarios, like Q&A accuracy, reasoning capabilities and more. In particular, to explore the capabilities of , we focused on a key area with significant potential for enterprise impact: The extraction of structured data, or metadata extraction. From complex enterprise content.
At Box, we rigorously evaluate data extraction models using multiple enterprise-grade datasets. One key dataset we leverage is CUAD, which consists of over 510 commercial legal contracts. Within this dataset, Box has identified 17,000 fields that can be extracted from unstructured content and evaluated the model based on single shot extraction for these fields (this is our hardest test. Where the model only has once chance to extract all the metadata in a single pass vs. taking multiple attempts). In our tests, correctly extracted 19 percentage points more fields accurately compared to GPT-4o, highlighting its improved ability to handle nuanced contract data.
Next, to ensure could handle the demands of real-world enterprise content. We evaluated its performance against a more rigorous set of documents, Box’s own challenge set. We selected a subset of complex legal contracts – those with multi-modal content, high-density information and. Lengths exceeding 200 pages – to represent some of the most difficult scenarios our clients face. On this challenge set, also consistently outperformed GPT-4o in extracting key fields with higher accuracy, demonstrating its superior ability to handle intricate and nuanced legal documents.
Overall, we’re seeing strong results with for complex enterprise data, which will unlock even more use-cases in the enterprise.“.
Even as early clients found workable — albeit a bit lazy — they questioned its release.
For instance, prominent OpenAI critic Gary Marcus called a “nothingburger” on Bluesky.
Hot take: GPT is a nothingburger; GPT-5 still fantasy.• Scaling data is not a physical law; pretty much everything I told you was true.• All the BS about GPT-5 we listened to for last few years: not so true.• Fanboys like Cowen will blame individuals. But results just aren’t what they had hoped. — Gary Marcus ( .
Hugging Face CEO Clement Delangue commented that ’s closed-source provenance makes it “meh.”.
However. Many noted that had nothing to do with its performance. Instead, people questioned why OpenAI would release a model so expensive that it is almost prohibitive to use but. Is not as powerful as its other models.
One user commented on X: “So you’re telling me is worth more than o1 yet it doesn’t perform as well on benchmarks…. Make it make sense.”.
Other X individuals posited theories that the high token cost could be to deter competitors like DeepSeek “to distill the model.”.
DeepSeek became a big competitor against OpenAI in January, with industry leaders finding DeepSeek-R1 reasoning to be as capable as OpenAI’s — but. More affordable.
Le quantum computing est une révolution du domaine informatique. Cette technologie émergente repose sur la mécanique quantique pour la conception de n...
You are training your latest AI model. Anxiously watching as the loss steadily decreases when suddenly — boom! Your logs are flooded with NaNs (Not a ...
Qui aurait cru que les grands-mères chinoises trouveraient plus de réconfort auprès de bébés générés par l’IA que de leurs petits-enfants ? Ces bébés ...
OpenAI Offers GPT-4.5 With 40% Fewer Hallucinations, 30x Higher Cost
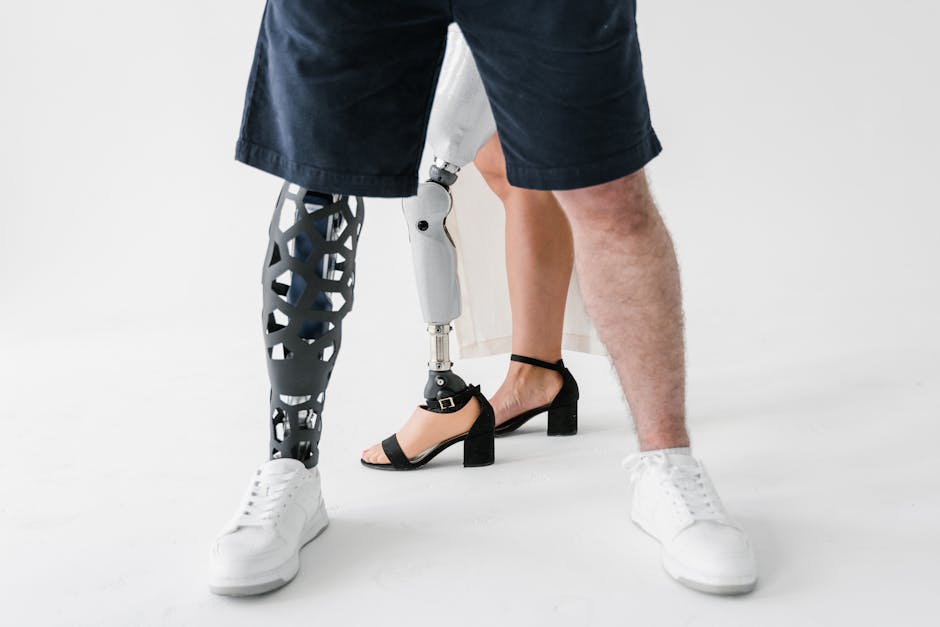
The rapid release of advanced AI models in the past few days has been impossible to ignore. With the launch of Grok-3 and Claude Sonnet, two leading AI companies, xAI and Anthropic. Have significantly accelerated the pace of innovation in the field.
As rumours about OpenAI’s newest model circulated, anticipation surged. However, when was released, OpenAI expressed it wasn’t a frontier model and was less powerful than the business’s o3-mini model and many others in the competition.
It doesn’t excel in coding, reasoning. Or any such capabilities, either—because it isn’t meant to be. At this time, OpenAI has focused more on the model’s usability than anything else.
OpenAI tested on the SimpleQA benchmark, a tool that evaluates the factual accuracy of AI models in answering short. Fact-seeking questions. The model achieved a hallucination rate of , in contrast to the o3-Mini, which recorded over 70%. The GPT-4o model exhibited a hallucination rate of 61%.
This indicates a 40% reduction in the hallucination rate compared to its predecessor. In accuracy rates on the SimpleQA benchmark, GPT scored , higher than OpenAI’s o3-mini (15%), o1 (47%), and GPT-4o ( This is also higher than many models in the competition, as the Grok-3 model scored a accuracy rate in the benchmark, the Gemini Pro scored , and. The Claude Sonnet scored .
OpenAI also released a system card for the model, which evaluates all the safety concerns and associated risks. In an evaluation called PersonQA, which tested the model for hallucinations, was more accurate and showed a lesser hallucination rate than the o1 and the GPT-4o models.
Given its availability at the $200/month pro plan, several clients agreed with OpenAI’s proposes of reduced hallucinations.
Aaron Levie, CEO of the cloud storage organization Box. Revealed that significantly improved over the GPT-4o in extracting data fields from enterprise content, like critical details in a contract. “We found a 19 pt [point] improvement in single shot extraction. This is a huge improvement for any mission-critical enterprise workflow,” he noted in a post on X.
Early testers of the model also gave high praise for the model’s verbal and. Emotional intelligence. “I found it to be by far the highest verbal intelligence model I’ve ever used. It’s an outstanding writer and conversationalist,” showcased Theo Jaffee, who had early access to the model.
‘First Model That Feels Like Talking to a Thoughtful Person’.
While CEO Sam Altman was absent from the launch event, he mentioned on X that “is the first model that feels like talking to a thoughtful person to me.”.
“I have had several moments where I’ve sat back in my chair and been astonished at getting actually good advice from an AI,” added Altman. And mentioned that the model offers a different kind of intelligence. There’s a magic to it that he hasn’t felt before.
The model supposedly excels at creative and emotional thinking. Ethan Mollick, a professor at The Wharton School, mentioned on X, “It can write beautifully, is very creative, and is occasionally oddly lazy on complex projects.” He even joked that the model took a “lot more” classes in the humanities.
Andrej Karpathy, the former OpenAI researcher and founder of Eureka Labs, found that two years ago, when he tested the GPT-4, the model’s word choice was more creative, and. He had improved understanding of the nuances of the prompt compared to Karpathy noted that he has a similar feeling for Everything is a little bit superior,” he noted.
OpenAI, in the model’s system card, stated internal testers reported as warm, intuitive, and natural. “When tasked with emotionally charged queries, it knows when to offer advice, defuse frustration, or simply listen to the user,” the study read.
Overall, the GPT isn’t a mind-blowing model. And it isn’t the best model on benchmarks either. For example, it is worse than the not long ago released Claude Sonent on coding benchmarks and offers only a marginal improvement over the GPT-4o.
Altman also confirmed earlier that the firm plans to release the GPT-5 model soon, combining general purpose and reasoning capabilities in a single model.
However. If the organization aims to make the available to the masses, there’s bad news. It isn’t available yet on the free version or even the $20/month plan. If it were to be deployed on other platforms via API, it would be the most expensive model, and its pricing is an exponential jump over GPT-4o or even the o3-mini.
The Preview costs $75 and. $150 per 1 million input and output tokens, respectively. In comparison, the GPT-4o costs $ and $10 per million input and output tokens, respectively.
Clement Delangue, CEO at HuggingFace, revealed, “IMO [in my opinion], if GPT was released as an open-source base model (that everyone can distill), it would be the most impactful release of the year,” and added that he isn’t a fan of the API either.
“Making a few hundred million [dollars] now from it via API doesn’t move the needle compared to the 10x more usage/visibility/goodwill/talent they could get by open-sourcing it,” he added.
OpenAI will have to watch out for the launch of DeepSeek-R2 and Meta’s Llama 4, which are expected to be out in a few months.
Moreover. If OpenAI is marketing the model for its creative and empathetic outputs, they are subjective metrics at the end of the day. Karpathy conducted a poll on X to check if customers prefer outputs of , or GPT-4o, and many customers preferred the latter. It will be interesting to see how many customers will be truly pleased with when it is released.
Researchers at Physical Intelligence, an AI robotics firm. Have developed a system called the Hierarchical Interactive Robot (Hi Robot). This syste...
Market Impact Analysis
Market Growth Trend
2018 | 2019 | 2020 | 2021 | 2022 | 2023 | 2024 |
---|---|---|---|---|---|---|
23.1% | 27.8% | 29.2% | 32.4% | 34.2% | 35.2% | 35.6% |
Quarterly Growth Rate
Q1 2024 | Q2 2024 | Q3 2024 | Q4 2024 |
---|---|---|---|
32.5% | 34.8% | 36.2% | 35.6% |
Market Segments and Growth Drivers
Segment | Market Share | Growth Rate |
---|---|---|
Machine Learning | 29% | 38.4% |
Computer Vision | 18% | 35.7% |
Natural Language Processing | 24% | 41.5% |
Robotics | 15% | 22.3% |
Other AI Technologies | 14% | 31.8% |
Technology Maturity Curve
Different technologies within the ecosystem are at varying stages of maturity:
Competitive Landscape Analysis
Company | Market Share |
---|---|
Google AI | 18.3% |
Microsoft AI | 15.7% |
IBM Watson | 11.2% |
Amazon AI | 9.8% |
OpenAI | 8.4% |
Future Outlook and Predictions
The Change Unless Industry landscape is evolving rapidly, driven by technological advancements, changing threat vectors, and shifting business requirements. Based on current trends and expert analyses, we can anticipate several significant developments across different time horizons:
Year-by-Year Technology Evolution
Based on current trajectory and expert analyses, we can project the following development timeline:
Technology Maturity Curve
Different technologies within the ecosystem are at varying stages of maturity, influencing adoption timelines and investment priorities:
Innovation Trigger
- Generative AI for specialized domains
- Blockchain for supply chain verification
Peak of Inflated Expectations
- Digital twins for business processes
- Quantum-resistant cryptography
Trough of Disillusionment
- Consumer AR/VR applications
- General-purpose blockchain
Slope of Enlightenment
- AI-driven analytics
- Edge computing
Plateau of Productivity
- Cloud infrastructure
- Mobile applications
Technology Evolution Timeline
- Improved generative models
- specialized AI applications
- AI-human collaboration systems
- multimodal AI platforms
- General AI capabilities
- AI-driven scientific breakthroughs
Expert Perspectives
Leading experts in the ai tech sector provide diverse perspectives on how the landscape will evolve over the coming years:
"The next frontier is AI systems that can reason across modalities and domains with minimal human guidance."
— AI Researcher
"Organizations that develop effective AI governance frameworks will gain competitive advantage."
— Industry Analyst
"The AI talent gap remains a critical barrier to implementation for most enterprises."
— Chief AI Officer
Areas of Expert Consensus
- Acceleration of Innovation: The pace of technological evolution will continue to increase
- Practical Integration: Focus will shift from proof-of-concept to operational deployment
- Human-Technology Partnership: Most effective implementations will optimize human-machine collaboration
- Regulatory Influence: Regulatory frameworks will increasingly shape technology development
Short-Term Outlook (1-2 Years)
In the immediate future, organizations will focus on implementing and optimizing currently available technologies to address pressing ai tech challenges:
- Improved generative models
- specialized AI applications
- enhanced AI ethics frameworks
These developments will be characterized by incremental improvements to existing frameworks rather than revolutionary changes, with emphasis on practical deployment and measurable outcomes.
Mid-Term Outlook (3-5 Years)
As technologies mature and organizations adapt, more substantial transformations will emerge in how security is approached and implemented:
- AI-human collaboration systems
- multimodal AI platforms
- democratized AI development
This period will see significant changes in security architecture and operational models, with increasing automation and integration between previously siloed security functions. Organizations will shift from reactive to proactive security postures.
Long-Term Outlook (5+ Years)
Looking further ahead, more fundamental shifts will reshape how cybersecurity is conceptualized and implemented across digital ecosystems:
- General AI capabilities
- AI-driven scientific breakthroughs
- new computing paradigms
These long-term developments will likely require significant technical breakthroughs, new regulatory frameworks, and evolution in how organizations approach security as a fundamental business function rather than a technical discipline.
Key Risk Factors and Uncertainties
Several critical factors could significantly impact the trajectory of ai tech evolution:
Organizations should monitor these factors closely and develop contingency strategies to mitigate potential negative impacts on technology implementation timelines.
Alternative Future Scenarios
The evolution of technology can follow different paths depending on various factors including regulatory developments, investment trends, technological breakthroughs, and market adoption. We analyze three potential scenarios:
Optimistic Scenario
Responsible AI driving innovation while minimizing societal disruption
Key Drivers: Supportive regulatory environment, significant research breakthroughs, strong market incentives, and rapid user adoption.
Probability: 25-30%
Base Case Scenario
Incremental adoption with mixed societal impacts and ongoing ethical challenges
Key Drivers: Balanced regulatory approach, steady technological progress, and selective implementation based on clear ROI.
Probability: 50-60%
Conservative Scenario
Technical and ethical barriers creating significant implementation challenges
Key Drivers: Restrictive regulations, technical limitations, implementation challenges, and risk-averse organizational cultures.
Probability: 15-20%
Scenario Comparison Matrix
Factor | Optimistic | Base Case | Conservative |
---|---|---|---|
Implementation Timeline | Accelerated | Steady | Delayed |
Market Adoption | Widespread | Selective | Limited |
Technology Evolution | Rapid | Progressive | Incremental |
Regulatory Environment | Supportive | Balanced | Restrictive |
Business Impact | Transformative | Significant | Modest |
Transformational Impact
Redefinition of knowledge work, automation of creative processes. This evolution will necessitate significant changes in organizational structures, talent development, and strategic planning processes.
The convergence of multiple technological trends—including artificial intelligence, quantum computing, and ubiquitous connectivity—will create both unprecedented security challenges and innovative defensive capabilities.
Implementation Challenges
Ethical concerns, computing resource limitations, talent shortages. Organizations will need to develop comprehensive change management strategies to successfully navigate these transitions.
Regulatory uncertainty, particularly around emerging technologies like AI in security applications, will require flexible security architectures that can adapt to evolving compliance requirements.
Key Innovations to Watch
Multimodal learning, resource-efficient AI, transparent decision systems. Organizations should monitor these developments closely to maintain competitive advantages and effective security postures.
Strategic investments in research partnerships, technology pilots, and talent development will position forward-thinking organizations to leverage these innovations early in their development cycle.
Technical Glossary
Key technical terms and definitions to help understand the technologies discussed in this article.
Understanding the following technical concepts is essential for grasping the full implications of the security threats and defensive measures discussed in this article. These definitions provide context for both technical and non-technical readers.